What is signal detection theory? It’s more than just figuring out if a faint sound is real or just background noise; it’s a powerful framework for understanding how we make decisions when faced with uncertainty. Think about a doctor interpreting a medical scan, an airport security agent screening luggage, or even you deciding if that rustle in the bushes is a threat or a squirrel.
Signal detection theory provides a mathematical model to analyze these situations, breaking down the decision-making process into key components like sensitivity (how well you distinguish signal from noise) and bias (your tendency to say “yes” or “no”). It’s a surprisingly versatile tool with applications spanning fields as diverse as medicine, psychology, and engineering.
At its core, signal detection theory revolves around the concept of separating a true signal from background noise. This isn’t always easy – sometimes the signal is weak, and sometimes the noise is overwhelming. The theory helps us understand how factors like the strength of the signal, the level of noise, and even our personal biases influence our decisions.
By analyzing the outcomes (hits, misses, false alarms, and correct rejections), we can quantitatively assess the performance of a detection system, whether it’s a human observer or a sophisticated piece of technology. We’ll explore these concepts in more detail, looking at the mathematical underpinnings, real-world applications, and limitations of this fascinating theory.
Signal Detection Theory
Signal detection theory (SDT) offers a powerful framework for understanding how we make decisions when faced with uncertainty. It moves beyond simply measuring the accuracy of a response and delves into the underlying processes of distinguishing a signal from noise, revealing the interplay between sensitivity and decision-making bias. This approach proves invaluable across diverse fields, from medical diagnosis to military surveillance.
Fundamental Principles of Signal Detection Theory
SDT’s core lies in distinguishing a signal (the target stimulus) from noise (background interference). Sensitivity (d’) quantifies the ability to discriminate between signal and noise; a higher d’ indicates better discrimination. Bias (β), on the other hand, reflects the decision criterion – the individual’s tendency to respond “yes” or “no” regardless of the evidence. Mathematically, these are related, though not directly through a simple formula.
A higher d’ means a greater separation between the signal and noise distributions, making it easier to distinguish them irrespective of bias. Bias shifts the decision criterion along the distribution of responses, altering the relative frequencies of hits, misses, false alarms, and correct rejections.For instance, in medical diagnosis, the signal might be the presence of a disease, and the noise could be background physiological variations.
A radiologist with high sensitivity (d’) will accurately identify diseased tissues even when the signal is weak, while a conservative bias (β) might lead to fewer false positives (diagnosing a healthy patient as sick) but also more missed diagnoses. In airport security, the signal is a concealed weapon, and the noise is other objects. A high-sensitivity security officer will detect more weapons, but a relaxed criterion might lead to more false alarms (flagging harmless items).A Receiver Operating Characteristic (ROC) curve visually represents the trade-off between hits and false alarms.
It’s constructed by plotting the hit rate (true positive rate) against the false alarm rate (false positive rate) at various decision criteria. A curve closer to the top-left corner indicates superior sensitivity. The area under the ROC curve (AUC) quantifies overall performance, with a perfect AUC of 1 indicating flawless discrimination.
Decision Criterion | Hits | Misses | False Alarms | Correct Rejections |
---|---|---|---|---|
Liberal | High | Low | High | Low |
Conservative | Low | High | Low | High |
Neutral | Moderate | Moderate | Moderate | Moderate |
Historical Overview of Signal Detection Theory
SDT emerged from the limitations of classical psychophysics, which focused solely on measuring the threshold of detection. Early contributions from researchers like W.J. McGill, and Wilson P. Tanner laid the groundwork. The seminal work of John A.
Swets in the 1950s and 60s significantly advanced the theory, emphasizing the importance of separating sensitivity and bias. This led to the widespread adoption of SDT across various disciplines.
Year | Milestone | Influential Figure(s) |
---|---|---|
1950s-1960s | Development of the formal theory of signal detection | John A. Swets, Wilson P. Tanner, David M. Green |
1960s-present | Broad application across various fields | Numerous researchers across diverse disciplines |
Signal, Noise, and Their Interaction
In SDT, a signal represents the target stimulus, while noise encompasses any interfering stimuli or internal variability. Noise can be external (e.g., background sounds, visual clutter) or internal (e.g., neural noise, attentional fluctuations). Different types of noise impact detection performance differently; for example, additive noise simply shifts the signal distribution, while multiplicative noise changes its spread.A graphical representation using probability density functions (PDFs) illustrates this interaction.
The signal PDF represents the probability distribution of responses when the signal is present, and the noise PDF represents the distribution when only noise is present. The overlap between these distributions determines the difficulty of discrimination. The decision criterion is a threshold on the response scale, separating “yes” from “no” responses. Shifting the criterion changes the balance between hits and false alarms.
Applications of Signal Detection Theory in Medical Diagnosis
In medical diagnosis, the signal is the presence of a disease (e.g., a cancerous tumor on an X-ray), and the noise comprises background variations in tissue density or imaging artifacts. Radiologists use SDT principles to interpret medical images, aiming for high sensitivity (detecting all cancers) while minimizing false alarms (incorrectly diagnosing healthy tissue as cancerous). By analyzing the ROC curve of radiologists’ performance, researchers can assess their diagnostic accuracy and identify areas for improvement.
Applications of Signal Detection Theory in Military Surveillance
In military surveillance, the signal could be an enemy aircraft on radar, and the noise might be atmospheric interference or other objects. Radar operators use SDT to interpret radar signals, attempting to maximize the detection of enemy aircraft while minimizing false alarms (identifying harmless objects as threats). The decision criterion will depend on the perceived risks and costs associated with missing a threat versus raising a false alarm.
Applications of Signal Detection Theory in Cognitive Psychology
In cognitive psychology, SDT helps understand attention and perception. The signal could be a faint visual stimulus, and the noise might be background visual clutter or internal neural noise. Researchers use SDT to measure the sensitivity of observers to different stimuli and to assess the impact of factors such as attention and cognitive load on detection performance. For example, studies might compare the ability to detect a target stimulus under different levels of distraction, quantifying the effects of noise on perception.
Case Study: Mammography Screening
A study on mammography screening examined the performance of radiologists in detecting breast cancer. The signal was the presence of a cancerous lesion, and the noise was normal breast tissue variations. The study used ROC curves to analyze the radiologists’ performance, comparing different age groups and experience levels. Results showed that experience improved sensitivity but did not significantly impact bias.
The study’s strength lay in its rigorous methodology and large sample size. However, it was limited by the specific imaging technology and patient population used.
Field | Signal | Noise | Decision Criterion | Consequences of Errors |
---|---|---|---|---|
Medical Diagnosis | Disease | Physiological variations | Balance between sensitivity and specificity | Missed diagnoses, false positives |
Military Surveillance | Enemy target | Environmental interference | Risk tolerance | Missed threats, false alarms |
Cognitive Psychology | Stimulus | Internal/external noise | Response bias | Missed detections, false positives |
Limitations of Signal Detection Theory
SDT assumes that the signal and noise distributions are normally distributed, which may not always hold true in real-world scenarios. The theory also simplifies the decision-making process, neglecting factors such as response time and confidence judgments. Furthermore, SDT might be insufficient when dealing with complex decisions involving multiple signals or sources of noise.
Extensions and Modifications of Signal Detection Theory
Researchers have extended SDT to accommodate more complex situations. Models incorporating multiple signals or multiple sources of noise have been developed, as well as models incorporating response time and confidence ratings. These extensions provide a more nuanced understanding of decision-making under uncertainty. For instance, extensions that account for response times allow for a more complete understanding of the underlying cognitive processes involved in signal detection.
Signal Detection Theory’s Components
Signal Detection Theory (SDT) isn’t just a fascinating theoretical framework; it’s a powerful tool for understanding how we make decisions amidst uncertainty. By breaking down the process of detecting a signal from noise, SDT provides valuable insights into various aspects of human perception and decision-making. Understanding its core components allows us to appreciate its wide-ranging applications in diverse fields.The cornerstone of SDT lies in its ability to analyze the four possible outcomes of a signal detection task.
Signal detection theory analyzes the ability to discern a signal from noise. Understanding this framework is crucial in healthcare, as accurate diagnosis relies on distinguishing relevant patient information from irrelevant data. This is directly related to the foundation of nursing knowledge, as explored in the resource, nursing knowledge is based on which of the following , which highlights the evidence-based nature of the profession.
Therefore, a strong grasp of signal detection theory enhances a nurse’s ability to interpret data and make informed decisions.
These outcomes, while seemingly simple, reveal a wealth of information about the observer’s sensitivity and response bias. Let’s explore these outcomes and their significance.
The Four Possible Outcomes of a Signal Detection Task
In any situation requiring the detection of a signal amidst background noise, four distinct outcomes are possible. These outcomes are crucial for understanding both the accuracy of detection and the decision-making strategy employed. Analyzing these outcomes allows us to separate the effects of sensitivity (the ability to discriminate between signal and noise) from response bias (the tendency to respond in a particular way).
- Hit: Correctly identifying the presence of a signal when a signal is indeed present. Imagine a security guard correctly identifying a potential intruder on a security camera feed.
- Miss: Failing to identify a signal when it is present. For example, a doctor missing a cancerous tumor on an X-ray.
- False Alarm: Incorrectly identifying a signal when no signal is present. A classic example would be a fire alarm going off due to steam, not a fire.
- Correct Rejection: Correctly identifying the absence of a signal when no signal is present. A radar system not detecting a non-existent aircraft is a correct rejection.
Real-World Applications of Signal Detection Theory
The elegance of SDT lies in its broad applicability. Its principles aren’t confined to laboratories; they extend to numerous real-world scenarios where decision-making under uncertainty is paramount.
- Medical Diagnosis: Doctors use SDT implicitly when interpreting medical images (X-rays, MRIs) to detect diseases. A high sensitivity is crucial to minimize misses, while controlling false alarms is essential to avoid unnecessary treatments.
- Air Traffic Control: Air traffic controllers must constantly scan radar screens for aircraft. SDT helps analyze their performance in detecting potential collisions, balancing the need for quick responses with the avoidance of false alarms that could disrupt air traffic.
- Military Surveillance: In military contexts, detecting enemy activity amidst background noise is critical. SDT can be used to evaluate the performance of surveillance systems and the decision-making processes of operators.
- Psychophysics: SDT is foundational in psychophysics, where it helps quantify the sensitivity of sensory systems (vision, hearing, touch) to various stimuli.
Sensitivity (d’) and its Implications
Sensitivity, often represented as ‘d-prime’ (d’), is a crucial measure within SDT. It quantifies the ability of an observer to distinguish between the presence and absence of a signal. A higher d’ indicates better discrimination between signal and noise. Importantly, d’ is independent of response bias. This means that a person could have high sensitivity but a tendency to respond “yes” more often (leading to more false alarms), or vice versa.
d’ represents the distance between the means of the noise and signal distributions. A larger distance indicates better discrimination.
Understanding d’ allows researchers and practitioners to assess the true discriminatory power of an observer or system, separate from any decision-making biases. For example, a security system with a high d’ would be more reliable in detecting intrusions regardless of whether its operators are prone to false alarms or misses. This distinction is critical for optimizing performance and designing more effective systems.
Receiver Operating Characteristic (ROC) Curve
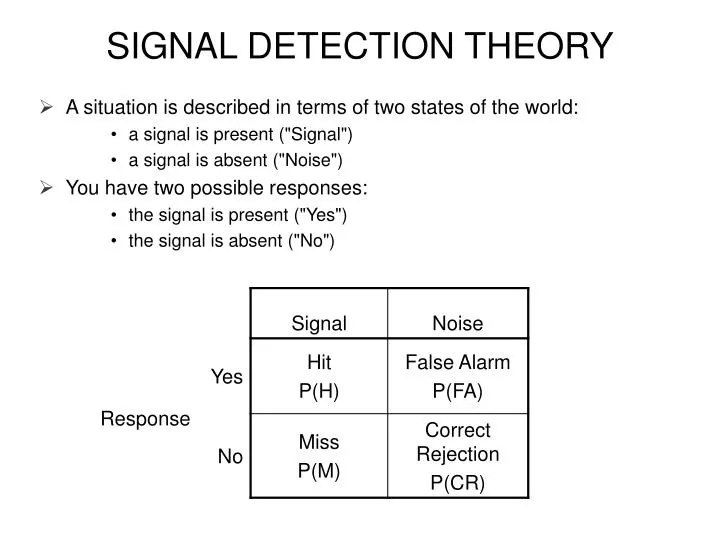
The Receiver Operating Characteristic (ROC) curve is a powerful graphical tool used to visualize, organize, and select classifiers based on their performance. It provides a comprehensive understanding of a system’s ability to distinguish between signal and noise, offering insights beyond simple accuracy metrics. Understanding the ROC curve allows for informed decisions about the optimal operating point of a system, balancing sensitivity and specificity based on the specific application.
The ROC curve plots the True Positive Rate (TPR) against the False Positive Rate (FPR) at various threshold settings. The TPR, also known as sensitivity or recall, represents the proportion of actual positives correctly identified by the system. Conversely, the FPR, also known as 1-specificity, represents the proportion of actual negatives incorrectly identified as positives. These rates are intrinsically linked; as the threshold for classifying a signal increases, the TPR typically decreases while the FPR decreases.
This trade-off is central to understanding the ROC curve.
True Positive Rate (TPR) and False Positive Rate (FPR)
The TPR and FPR are calculated from a confusion matrix, which summarizes the performance of a binary classification system. A higher TPR indicates better sensitivity (correctly identifying positive cases), while a lower FPR indicates higher specificity (correctly identifying negative cases). The optimal balance between TPR and FPR depends heavily on the context. For example, in medical diagnosis, a high TPR might be prioritized (minimizing false negatives, even at the cost of some false positives) to ensure that potentially life-threatening conditions are not missed.
Conversely, in spam filtering, a low FPR might be prioritized (minimizing false positives, even if it means missing some spam emails) to avoid mistakenly flagging legitimate emails as spam.
Area Under the ROC Curve (AUC) Calculation
The area under the ROC curve (AUC) provides a single numerical summary of the classifier’s performance. A higher AUC indicates better discriminatory power. Two common methods for calculating the AUC are the trapezoidal rule and numerical integration using more sophisticated methods.
Trapezoidal Rule
The trapezoidal rule approximates the area under the curve by dividing it into a series of trapezoids. The formula is:
AUC ≈ Σi=1n-1 [(FPR i+1
- FPR i)/2]
- (TPR i+1 + TPR i)
where n is the number of data points, FPR i and TPR i are the false positive rate and true positive rate at the i-th threshold.
Numerical Integration (Simpson’s Rule)
Simpson’s rule is a more accurate numerical integration method that uses quadratic polynomials to approximate the curve. The formula for Simpson’s rule is:
AUC ≈ (Δx/3)[f(x0) + 4f(x 1) + 2f(x 2) + 4f(x 3) + … + 2f(x n-2) + 4f(x n-1) + f(x n)]
where Δx is the interval width and f(x i) represents the function value (TPR) at the i-th FPR value. This method offers greater accuracy, especially with a larger number of data points.
ROC Curve Comparison of Three Systems
Let’s compare three hypothetical signal detection systems (A, B, and C) using ROC curves. The following table shows example data points:
System | Threshold | True Positives | False Positives | True Negatives | False Negatives | TPR | FPR |
---|---|---|---|---|---|---|---|
System A | 0.1 | 95 | 80 | 20 | 5 | 0.95 | 0.80 |
0.2 | 90 | 60 | 40 | 10 | 0.90 | 0.60 | |
0.3 | 85 | 40 | 60 | 15 | 0.85 | 0.40 | |
0.4 | 80 | 30 | 70 | 20 | 0.80 | 0.30 | |
0.5 | 75 | 20 | 80 | 25 | 0.75 | 0.20 | |
0.6 | 70 | 10 | 90 | 30 | 0.70 | 0.10 | |
0.7 | 65 | 5 | 95 | 35 | 0.65 | 0.05 | |
0.8 | 60 | 2 | 98 | 40 | 0.60 | 0.02 | |
0.9 | 55 | 1 | 99 | 45 | 0.55 | 0.01 | |
1.0 | 50 | 0 | 100 | 50 | 0.50 | 0.00 | |
System B | 0.1 | 80 | 20 | 80 | 20 | 0.80 | 0.20 |
0.2 | 75 | 15 | 75 | 25 | 0.75 | 0.15 | |
0.3 | 70 | 10 | 70 | 30 | 0.70 | 0.10 | |
0.4 | 65 | 5 | 65 | 35 | 0.65 | 0.05 | |
0.5 | 60 | 2 | 60 | 40 | 0.60 | 0.02 | |
0.6 | 55 | 1 | 55 | 45 | 0.55 | 0.01 | |
0.7 | 50 | 0 | 50 | 50 | 0.50 | 0.00 | |
0.8 | 45 | 0 | 45 | 55 | 0.45 | 0.00 | |
0.9 | 40 | 0 | 40 | 60 | 0.40 | 0.00 | |
1.0 | 35 | 0 | 35 | 65 | 0.35 | 0.00 | |
System C | 0.1 | 98 | 90 | 10 | 2 | 0.98 | 0.90 |
0.2 | 96 | 70 | 30 | 4 | 0.96 | 0.70 | |
0.3 | 94 | 50 | 50 | 6 | 0.94 | 0.50 | |
0.4 | 92 | 30 | 70 | 8 | 0.92 | 0.30 | |
0.5 | 90 | 20 | 80 | 10 | 0.90 | 0.20 | |
0.6 | 88 | 10 | 90 | 12 | 0.88 | 0.10 | |
0.7 | 86 | 5 | 95 | 14 | 0.86 | 0.05 | |
0.8 | 84 | 2 | 98 | 16 | 0.84 | 0.02 | |
0.9 | 82 | 1 | 99 | 18 | 0.82 | 0.01 | |
1.0 | 80 | 0 | 100 | 20 | 0.80 | 0.00 |
Bias in Signal Detection
Understanding bias in signal detection is crucial for accurate interpretation of results across numerous fields. It’s not simply about the presence or absence of a signal, but also about the decision-making process influenced by various factors, leading to systematic errors in judgment. This section delves into the nature, sources, measurement, and mitigation of response bias within the framework of signal detection theory.
Response Bias: Conceptualization and Influence on Decision-Making
Response bias, in signal detection theory, refers to a systematic tendency to respond in a particular way regardless of the strength of the evidence. This means a person might consistently say “yes” even when the evidence is weak, or consistently say “no” even when the evidence is strong. Examples include liberal bias (tending to say “yes” more often) and conservative bias (tending to say “no” more often).
A neutral bias represents a balanced approach.Response bias significantly impacts the accuracy of decisions, affecting the rates of hits (correctly identifying a signal), misses (failing to identify a signal), false alarms (incorrectly identifying noise as a signal), and correct rejections (correctly identifying noise as noise). This can be illustrated using a 2×2 confusion matrix:
Signal Present | Signal Absent | |
---|---|---|
Response: Yes | Hits | False Alarms |
Response: No | Misses | Correct Rejections |
A liberal bias increases hits and false alarms, while a conservative bias increases misses and correct rejections. In medical diagnosis, a liberal bias might lead to more false positives (diagnosing illness when none exists), while a conservative bias might lead to more false negatives (missing actual illnesses). In security screening, a liberal bias might lead to many false alarms (flagging innocent individuals), while a conservative bias might miss actual threats.
Eyewitness testimony can be heavily influenced by response bias, leading to misidentification of suspects.
Factors Contributing to Response Bias
Several factors contribute to response bias, often interacting in complex ways. These can be categorized into individual differences, situational factors, and task characteristics.
Factor | Description | Example | Impact on Decision-Making |
---|---|---|---|
Individual Differences (e.g., personality traits) | Personality traits like risk aversion or impulsivity can influence response tendencies. | A risk-averse individual might exhibit a conservative bias in medical diagnosis, avoiding false positives even at the cost of missing some illnesses. | Leads to consistently conservative or liberal responses, regardless of the actual signal strength. |
Situational Factors (e.g., time pressure) | Environmental pressures can alter decision-making strategies. | Airport security screeners under time pressure might adopt a more liberal bias, increasing false alarms to minimize the risk of missing a threat. | Can lead to hasty decisions with increased error rates, often favoring speed over accuracy. |
Task Characteristics (e.g., ambiguity of stimuli) | The clarity and complexity of the task influence response strategies. | Radiologists interpreting ambiguous medical images might show a conservative bias to avoid misdiagnosis, leading to missed diagnoses. | Ambiguous stimuli can increase uncertainty and lead to more cautious or less cautious responses. |
Instructional Factors (e.g., reward structure) | The way a task is presented influences the response strategy. | Offering a higher reward for correct identifications can encourage a more liberal response bias. | Incentives can significantly shift response biases towards maximizing rewards. |
Cognitive Factors (e.g., cognitive load) | Mental workload affects the capacity for careful signal processing. | A fatigued air traffic controller might adopt a more liberal bias, increasing false alarms to avoid missing potential conflicts. | High cognitive load can impair judgment and lead to simplified decision-making strategies, often resulting in increased bias. |
Measuring and Controlling for Response Bias
Several methods exist to measure response bias. Calculating d’ (sensitivity) and β (criterion) in signal detection theory provides a quantitative measure of both the ability to discriminate between signal and noise and the response bias. d’ reflects the strength of the signal, while β reflects the decision criterion. Analyzing the hit rate and false alarm rate allows for the calculation of these parameters.
Signal detection theory analyzes the ability to discern a signal from noise. Understanding the factors influencing this discrimination, such as biases and sensitivity, is crucial in various fields. A related concept involves the complexities of social interactions, prompting the question of whether hybridity constitutes a sociological theory, as explored in this insightful article: is hybridity a sociolgical theory.
Returning to signal detection, the application of this theory extends to interpreting social signals and understanding the challenges of accurate perception within complex social environments.
Another method involves analyzing response distributions, comparing the proportion of “yes” and “no” responses across different signal strengths. However, these methods require specific experimental designs.Controlling response bias requires careful experimental design. Strategies include using balanced instructions (avoiding leading questions), employing multiple observers to compare results, and using objective measures to assess performance. For example, in medical diagnosis, blinding the diagnostician to the patient’s history can reduce bias.
Failing to account for response bias can lead to inaccurate conclusions, flawed research findings, and unethical practices. For instance, in legal settings, ignoring response bias in eyewitness testimony can lead to wrongful convictions.
Case Study Analysis
The wrongful conviction of Steven Avery, featured in the Netflix documentary “Making a Murderer,” exemplifies the significant role of response bias in a real-world scenario. The investigation was heavily influenced by confirmation bias, a type of response bias where evidence supporting pre-existing beliefs is favored. Investigators focused on Avery, neglecting other potential suspects and interpreting ambiguous evidence to support their preconceived notions.
This bias could have been mitigated through a more thorough and impartial investigation, involving independent review of evidence and considering alternative hypotheses. The consequences included a wrongful conviction and years of imprisonment for Avery.
Future Directions
Research on response bias continues to evolve, exploring its neural mechanisms and developing advanced statistical techniques for its detection and correction. Areas needing further investigation include the interplay between different types of bias and the development of effective interventions for various contexts. AI-powered decision support systems hold potential for mitigating bias by providing objective analyses of data and flagging potential biases in decision-making processes.
These systems could be trained on large datasets to identify patterns associated with biased responses, assisting human decision-makers in making more accurate judgments.
Applications of Signal Detection Theory
Signal detection theory (SDT) finds widespread application across diverse fields, offering a powerful framework for analyzing decision-making in the presence of uncertainty. Its ability to separate the sensitivity of a system from its response bias makes it invaluable in understanding performance in various contexts, from medical diagnosis to radar detection. This section explores some key applications, illustrating the versatility and impact of SDT.
Applications of Signal Detection Theory in Medical Diagnosis
The application of SDT in medical diagnosis provides a robust method for evaluating the accuracy of diagnostic tests and comparing the performance of different diagnostic tools. By framing medical decisions as a process of distinguishing between the presence or absence of a disease (signal) amidst background noise (non-disease related factors), SDT offers a more nuanced understanding than simply relying on raw accuracy rates.
Three distinct examples of SDT applications in medical diagnosis are presented below:
Example | Signal | Noise | Decision Criteria | Potential Consequences of Errors |
---|---|---|---|---|
Mammography for Breast Cancer Detection | Presence of a cancerous tumor | Benign lumps, dense breast tissue, imaging artifacts | Threshold of suspiciousness for radiologist interpretation (e.g., size, shape, density of abnormality) | False Positive: Unnecessary biopsy, anxiety; False Negative: Delayed treatment, poorer prognosis |
Blood Test for HIV | Presence of HIV antibodies | Variations in antibody levels due to other infections, test error | Threshold level of antibody concentration | False Positive: Unnecessary anxiety, further testing; False Negative: Delayed treatment, potential infection spread |
Electrocardiogram (ECG) for Heart Attack Detection | Characteristic ECG changes indicative of myocardial infarction | Normal heart rhythm variations, electrical interference | Deviation from established normal ECG patterns | False Positive: Unnecessary hospitalization, stress; False Negative: Delayed treatment, increased risk of complications |
In mammography, for instance, a more liberal decision criterion (lower threshold for classifying a finding as suspicious) would increase the number of false positives (calling benign findings cancerous) while decreasing false negatives (missing actual cancers). Conversely, a more conservative criterion (higher threshold) would reduce false positives but increase false negatives. This trade-off is visually represented by the ROC curve.
The ROC curve plots the true positive rate (sensitivity) against the false positive rate (1-specificity) for various decision criteria. A curve closer to the upper left corner indicates superior diagnostic accuracy.
Signal detection theory facilitates the comparison of different diagnostic tools by quantifying their performance using metrics like d’ (sensitivity index) and β (bias). For example, comparing MRI and CT scans for brain tumor detection, we could calculate d’ for each modality. A higher d’ value for one modality indicates better ability to discriminate between the presence and absence of a tumor, irrespective of response bias.
The β value provides information about the decision-making strategy of the clinician (or the algorithm used) in each case. For instance, a higher β suggests a more conservative approach.
Applications of Signal Detection Theory in Psychology and Psychophysics
SDT provides a rigorous framework for understanding sensory perception and cognitive processes, moving beyond simple accuracy measures to disentangle sensitivity and response bias.
Two experiments in psychophysics illustrating the use of SDT to measure sensory thresholds are:
- Experiment 1: Auditory Detection Threshold. Participants are presented with a series of trials, each containing either a faint tone (signal) or silence (noise). They indicate whether they heard a tone. The response data (hits, misses, false alarms, correct rejections) are used to calculate d’ (sensitivity to the tone) and β (response bias – tendency to say “yes” or “no”). A higher d’ indicates a lower detection threshold.
- Experiment 2: Visual Discrimination Threshold. Participants are presented with pairs of stimuli that differ slightly in brightness. They indicate which stimulus is brighter. The data are again used to calculate d’ (sensitivity to brightness differences) and β (response bias). A higher d’ indicates better ability to discriminate brightness levels.
In visual search tasks, SDT helps analyze the impact of set size (number of distractors) and target salience (how visually distinct the target is) on search performance. Increased set size or reduced target salience would decrease d’, indicating reduced sensitivity in detecting the target. β could reflect a participant’s tendency to respond more cautiously (higher β) with increased uncertainty.
In eyewitness testimony research, SDT can assess the accuracy of eyewitness identifications. Witness confidence is often treated as a response criterion (β). High confidence does not necessarily indicate accurate identification; a biased witness (high β) might show high confidence even with low sensitivity (d’). Lineup composition (signal-to-noise ratio) also influences d’ and β. A lineup with highly similar distractors (high noise) could reduce d’, even for a sensitive witness.
Applications of Signal Detection Theory in Radar Detection and Other Engineering Fields
SDT forms the foundation of many signal processing techniques in engineering, enabling the reliable detection of signals in noisy environments.
In radar systems, the signal represents the reflection from a target, while noise includes thermal noise, clutter (unwanted reflections from the environment), and interference. Signal processing techniques, such as matched filtering, enhance the signal-to-noise ratio, improving d’. Different types of noise are addressed by employing specialized filters and algorithms tailored to their characteristics.
In communication systems, SDT is used to improve data transmission reliability. The signal represents the transmitted data, and the noise is channel noise (interference, attenuation). Error correction codes and modulation schemes are designed to minimize the impact of noise, maximizing d’.
In industrial process fault detection, SDT can analyze sensor data to identify anomalies. For example, in a manufacturing process, the signal represents deviations from expected operating parameters (e.g., temperature, pressure), and noise includes sensor errors and normal process variations. A decision-making process, potentially implemented as a control chart, would compare the measured signal to a threshold (decision criterion) to trigger an alarm if a fault is detected.
A flowchart would illustrate the steps: data acquisition, signal processing, comparison to threshold, and action (e.g., halt production, initiate maintenance).
Mathematical Models in Signal Detection Theory
Signal Detection Theory (SDT) provides a powerful framework for understanding decision-making in the presence of uncertainty. Its mathematical models allow us to quantify the sensitivity of an observer to a signal and the bias in their decision-making process. These models are crucial for interpreting data and making predictions across a wide range of applications.
SDT Models: Receiver Operating Characteristic (ROC) Curve
The Receiver Operating Characteristic (ROC) curve is a graphical representation of the trade-off between hit rate and false alarm rate at various decision criteria. It’s derived by plotting the hit rate (proportion of signals correctly identified) against the false alarm rate (proportion of noise incorrectly identified as signals) for different decision thresholds. The curve’s shape reflects the observer’s sensitivity (d’): a steeper curve indicates higher sensitivity, meaning the observer can better distinguish signal from noise.
The point on the curve closest to the upper left corner represents the optimal balance between minimizing misses and false alarms. For instance, an ROC curve close to the diagonal represents chance performance, while a curve that bows sharply towards the upper left corner indicates excellent discrimination. The area under the ROC curve (AUC) provides a quantitative measure of sensitivity, ranging from 0.5 (chance) to 1.0 (perfect discrimination).
The criterion (β) is reflected in the specific point on the ROC curve that the observer selects; a more liberal criterion (lower β) shifts the operating point towards higher hit and false alarm rates, while a conservative criterion (higher β) shifts it towards lower hit and false alarm rates.
d’ (Sensitivity) Calculation
The sensitivity index, d’, quantifies the observer’s ability to discriminate between signal and noise. It’s calculated as the difference between the Z-scores of the hit rate (H) and the false alarm rate (FA): d’ = Z(H)Z(FA). This calculation assumes that the underlying distributions of sensory evidence for signal and noise are normally distributed with equal variances. For example, if the hit rate is 0.84 and the false alarm rate is 0.16, their corresponding Z-scores are approximately 1 and -1, respectively, yielding a d’ of 2.
A higher d’ value indicates better discrimination. Conversely, a d’ of 0 suggests no ability to distinguish signal from noise. The assumption of normality is crucial; deviations from normality can affect the accuracy of d’ estimates.
β (Criterion/Bias) Calculation
The criterion, β, reflects the observer’s decision bias. It’s calculated as the ratio of the Z-scores of the false alarm rate and the hit rate: β = Z(FA) / Z(H). A β value of 1 indicates a neutral criterion; values less than 1 indicate a liberal criterion (more willing to say “signal”), and values greater than 1 indicate a conservative criterion (more cautious, less willing to report a signal).
Consider a scenario where a radiologist is screening mammograms. A liberal criterion might lead to more false positives (calling benign lesions cancerous), while a conservative criterion might lead to more false negatives (missing actual cancerous lesions). The optimal criterion depends on the costs associated with different types of errors.
Comparison of Models: High-Threshold vs. Low-Threshold Models
High-threshold models assume that a single criterion must be exceeded for a response to occur, representing a simple decision process. Low-threshold models, on the other hand, allow for multiple criteria and responses, offering a more nuanced approach to modeling decision-making. High-threshold models are simpler and easier to implement but may not capture the complexity of real-world decision-making processes. Low-threshold models provide a richer representation but are more complex mathematically.
The choice of model depends on the complexity of the task and the level of detail required.
Underlying Assumptions: Normality Assumption
The assumption of normally distributed sensory evidence is crucial for the accurate calculation of d’ and β. If this assumption is violated (e.g., due to skewed distributions), the calculated values might be inaccurate or misleading. Non-normal distributions can lead to underestimation or overestimation of sensitivity depending on the nature of the deviation. Robust alternatives to the standard SDT model exist for handling non-normal data.
Underlying Assumptions: Equal Variance Assumption
The assumption of equal variance in the signal and noise distributions simplifies the calculations of d’ and β. However, if the variances are unequal, the standard d’ calculation becomes inaccurate. Methods exist to adjust for unequal variances, but these methods add complexity to the analysis. Unequal variances can lead to biased estimates of sensitivity.
Underlying Assumptions: Independence Assumption
The standard SDT model assumes that observations are independent. If observations are correlated (e.g., due to temporal or spatial dependencies), the standard calculations of d’ and β might not be valid. Corrections for non-independence are possible but require more sophisticated statistical techniques. Ignoring dependence can lead to inflated estimates of sensitivity.
Key Equations and Formulas
Equation | Description | Variables |
---|---|---|
d’ = Z(H)
| Sensitivity (d-prime) calculation | Z(H) = Z-score of hit rate; Z(FA) = Z-score of false alarm rate |
β = Z(FA) / Z(H) | Criterion (beta) calculation | Z(H) = Z-score of hit rate; Z(FA) = Z-score of false alarm rate |
H = Φ(Z(signal) – β) | Hit Rate | Φ is the cumulative distribution function of the standard normal distribution, Z(signal) is the z-score corresponding to the signal distribution’s mean, β is the decision criterion. |
FA = Φ(-β) | False Alarm Rate | Φ is the cumulative distribution function of the standard normal distribution, β is the decision criterion. |
Application & Examples: Medical Diagnosis
In medical diagnosis, the signal is the presence of a disease, and the noise is the variability in test results or symptoms. A high d’ indicates a highly sensitive and specific diagnostic test. A liberal criterion (low β) might lead to more false positives (unnecessary treatments), while a conservative criterion (high β) might lead to more false negatives (missed diagnoses).
The limitations include the complexities of human disease, where symptoms may overlap and test results can be ambiguous.
Application & Examples: Radar Detection
In radar detection, the signal is a target’s reflection, and the noise is background clutter. A high d’ indicates a radar system’s ability to distinguish targets from noise. A liberal criterion might lead to more false alarms (detecting non-targets), while a conservative criterion might lead to more misses (failing to detect actual targets). Limitations include environmental factors (weather, terrain) that can affect signal strength and introduce additional noise.
Application & Examples: Eyewitness Testimony
In eyewitness testimony, the signal is the memory of an event, and the noise is the influence of other factors (stress, suggestion). A high d’ indicates a witness’s ability to accurately recall details. A liberal criterion might lead to more false identifications, while a conservative criterion might lead to more missed identifications. Limitations include the inherent fallibility of human memory and the susceptibility to suggestion.
Limitations and Extensions
While SDT is a powerful framework, its limitations include the assumptions of normality and equal variance, which may not always hold in real-world scenarios. Extensions and alternative models, such as those that accommodate non-normal distributions or correlated observations, are constantly being developed to address these limitations. Furthermore, SDT primarily focuses on the discrimination between two alternatives (signal vs.
noise), and extensions are needed for more complex scenarios involving multiple alternatives.
Limitations of Signal Detection Theory
Signal Detection Theory (SDT) is a powerful framework for understanding decision-making under uncertainty, but like any model, it possesses inherent limitations and assumptions that restrict its applicability. Understanding these limitations is crucial for appropriately applying SDT and interpreting its results. It’s important to remember that SDT provides a valuable lens through which to view decision-making, but it’s not a universally applicable solution.While SDT elegantly models the process of distinguishing signals from noise, its effectiveness hinges on several key assumptions.
Failure to meet these assumptions can lead to inaccurate or misleading conclusions. Furthermore, certain real-world scenarios simply don’t align with the core principles of SDT, rendering it an inappropriate analytical tool in those instances. Exploring these limitations helps us appreciate the breadth and boundaries of SDT’s power.
Assumptions of Signal Detection Theory
SDT rests on several core assumptions, the violation of which can significantly impact the validity of its predictions. A primary assumption is that the sensory information follows a normal distribution. This means the distribution of sensory inputs, both in the presence and absence of a signal, is bell-shaped and symmetrical. Deviations from this normality, such as skewed distributions, can compromise the accuracy of SDT’s analyses.
Another key assumption is the independence of observations. SDT assumes that each decision is made independently of previous decisions, ignoring potential effects of learning, fatigue, or context. This independence assumption is frequently violated in real-world tasks involving extended observation periods. Finally, SDT assumes that the observer’s criterion remains constant across trials. However, in many situations, the observer’s response bias might shift due to factors such as changes in motivation or the perceived consequences of making different types of errors.
For example, a radiologist might become more cautious (shifting their criterion) after missing a serious diagnosis in a previous case.
Situations Where Signal Detection Theory May Not Be Applicable
There are several scenarios where the application of SDT might be problematic or even inappropriate. One such scenario is when the decision-making process involves complex cognitive operations beyond simple signal detection. For example, diagnosing a complex medical condition requires integrating multiple pieces of information, some of which might be qualitative rather than purely quantitative. SDT’s focus on a single sensory dimension may not adequately capture the complexity of such decision-making.
Similarly, SDT might not be suitable for analyzing situations where the observer’s response is influenced by factors outside the sensory input, such as social pressure, emotional state, or moral considerations. A juror’s decision in a court case, for example, is influenced by many factors beyond the simple detection of evidence. Finally, SDT’s assumption of a stable criterion might not hold in dynamic environments where the costs and benefits of different response types are constantly changing.
In a rapidly evolving situation, such as a military operation, an observer’s criterion might fluctuate significantly, rendering the application of a constant-criterion model inappropriate.
Comparison with Alternative Decision-Making Models
While SDT offers a valuable framework, other models provide alternative perspectives on decision-making. For instance, Bayesian models explicitly incorporate prior probabilities and the costs and benefits of different decisions, which are not explicitly incorporated in the basic SDT framework. These models allow for more nuanced analyses of decision-making in situations where prior information is available or where the consequences of different errors vary significantly.
Another alternative is the use of connectionist models, which utilize neural networks to simulate decision-making processes. These models can handle complex, non-linear relationships between inputs and outputs, providing a more flexible approach to modelling human decision-making than SDT, although they can be more difficult to interpret. The choice of model depends on the specific research question and the nature of the decision-making task under investigation.
The strengths of SDT lie in its simplicity and its focus on separating sensitivity from bias, making it a useful tool in many contexts. However, researchers should carefully consider the limitations of SDT and the potential advantages of alternative models when designing and interpreting their studies.
Signal Detection and Decision Making
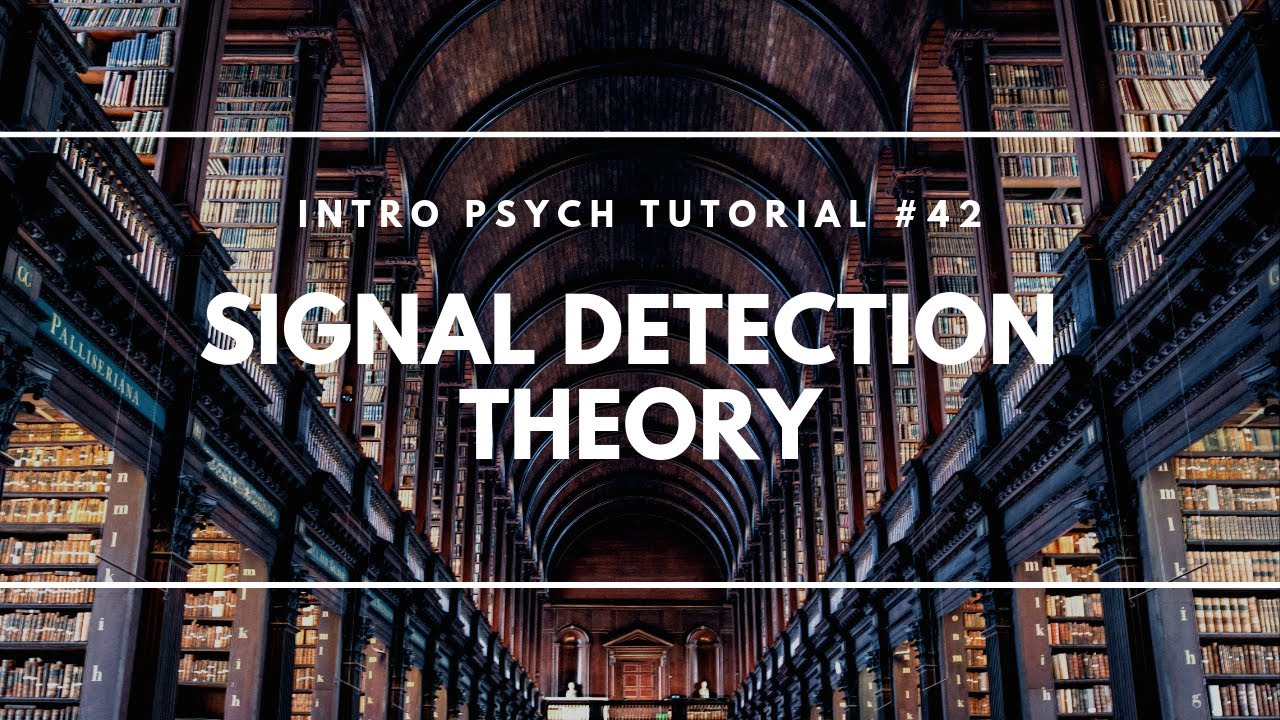
Signal detection theory elegantly bridges the gap between sensory perception and the complex world of human decision-making. It reveals how our brains actively process sensory information, weighing the evidence for and against a signal’s presence, ultimately leading to a decision. This process isn’t simply a passive reception of stimuli; rather, it’s a dynamic interplay of internal criteria and external context.
Understanding this relationship unlocks insights into diverse fields, from medical diagnosis to eyewitness testimony.Signal detection theory posits that every decision involves a trade-off between correctly identifying a signal (a “hit”) and incorrectly identifying noise as a signal (a “false alarm”). This trade-off is influenced by both the strength of the signal itself and the decision-maker’s internal criteria, shaped by factors such as experience, expectations, and the perceived costs and benefits associated with different outcomes.
For instance, a radiologist interpreting a medical scan will employ a different decision criterion than a security guard monitoring a surveillance camera; the consequences of missing a cancerous tumor are far greater than missing a minor anomaly on a security feed.
The Role of Context and Experience in Signal Detection
Context and experience profoundly shape our signal detection abilities. Prior knowledge, expectations, and even the surrounding environment can significantly alter our sensitivity to a signal and our willingness to report its presence. Imagine a seasoned birdwatcher identifying a rare bird in a dense forest. Their extensive experience with bird identification and knowledge of the forest’s ecosystem will significantly enhance their ability to detect subtle visual and auditory cues compared to a novice observer.
Similarly, a doctor who has seen many cases of a particular disease will be more adept at detecting subtle symptoms in a patient than a less experienced colleague. These contextual factors effectively change the internal criterion used in the decision-making process, influencing the balance between hits and false alarms.
A Hypothetical Experiment on Contextual Effects in Signal Detection
To investigate the impact of context on signal detection, we could design an experiment focusing on auditory signal detection. Participants would be presented with a series of faint tones embedded within background noise. The experimental manipulation would involve varying the context provided to participantsbefore* the tone detection task. One group (the “high-expectation” group) would be told that the tones are likely to be present and easily discernible, while another group (the “low-expectation” group) would be told that the tones are rare and difficult to detect.
A control group would receive no prior information. The dependent variable would be the number of hits and false alarms reported by each group. We would predict that the high-expectation group would show a higher rate of hits, but also a higher rate of false alarms, reflecting a lowered decision criterion. Conversely, the low-expectation group would likely demonstrate fewer hits and fewer false alarms, indicating a more conservative decision criterion.
This experiment would provide quantitative evidence supporting the significant role context plays in influencing signal detection performance.
Noise and its impact on Signal Detection
Signal detection theory acknowledges that the detection of a signal is rarely a straightforward process. Instead, it’s often clouded by noise – interfering stimuli that obscure the signal we’re trying to identify. Understanding the nature and impact of noise is crucial to improving the accuracy and reliability of any detection system, whether it’s a radar detecting an aircraft, a doctor diagnosing a disease, or a person discerning a friend’s voice in a crowded room.
This section explores the various types of noise, their influence on detection sensitivity, and strategies for mitigating their effects.
Types of Noise Affecting Signal Detection
Noise can manifest in many forms, significantly impacting the ability to accurately detect a target signal. These different types of noise possess unique characteristics that influence how they interfere with signal detection. Understanding these variations is vital for designing effective signal detection systems.
- Internal Noise: This refers to noise originating from within the detection system itself. For example, in a biological system like the human auditory system, internal noise might include spontaneous neural activity in the auditory nerve, creating a background hum that can mask faint sounds. Similarly, in electronic equipment, thermal noise (random fluctuations in electron movement due to heat) and shot noise (random variations in electron flow) are examples of internal noise.
The effects of internal noise are often difficult to eliminate entirely.
- External Noise: This encompasses any noise originating from sources outside the detection system. In a radar system, external noise might include atmospheric interference, reflections from other objects, or radio frequency emissions from other devices. In a medical imaging system, external noise could be caused by patient movement or ambient electromagnetic fields. External noise is often more readily controlled or minimized compared to internal noise.
- Environmental Noise: This category includes noise arising from the environment surrounding the detection system. For instance, in speech recognition, background conversations or traffic noise are examples of environmental noise. In a seismograph measuring earthquake activity, environmental noise might include vibrations from nearby construction or wind. Effective strategies for reducing the impact of environmental noise often involve careful placement of the detection system or the use of noise-canceling techniques.
Noise’s Effect on Detection Sensitivity (d’)
Noise directly impacts the sensitivity (d’) of a detection system, a measure of how well the system can discriminate between the presence and absence of a signal. A higher d’ value indicates greater sensitivity. Noise increases the overlap between the probability distributions representing the signal-present and signal-absent conditions. This overlap makes it harder to distinguish between the two conditions, resulting in a lower d’.
Consider a simple example: Imagine trying to detect a faint light (signal) against a dark background (noise). If the background is very noisy (e.g., many flickering lights), the faint light becomes harder to distinguish, thus reducing d’. Conversely, a quieter background (less noise) allows for easier detection and increases d’.
Strategies for Reducing Noise Impact
Minimizing the effects of noise is paramount for improving the performance of signal detection systems. Several strategies can be employed to achieve this goal. These techniques aim to either reduce the noise level or enhance the signal, effectively improving the signal-to-noise ratio.
- Signal Averaging: Repeated measurements of the signal can be averaged to reduce the impact of random noise. The noise, being random, tends to cancel itself out over multiple measurements, while the signal remains consistent, thus enhancing the signal-to-noise ratio.
- Filtering: Filters can be used to selectively remove or attenuate frequencies or components of the noise that are not part of the signal. This is a common technique in signal processing, with various filter types (e.g., low-pass, high-pass, band-pass) designed to target specific frequency ranges.
- Signal Enhancement Techniques: These techniques aim to amplify or otherwise improve the signal itself, making it more distinguishable from the background noise. Examples include wavelet transforms, which can decompose a signal into different frequency components, making it easier to separate the signal from noise.
- Optimized System Design: Careful design of the detection system can minimize the introduction of internal noise. This involves selecting appropriate components, shielding the system from external interference, and employing robust signal processing algorithms.
Bayesian Approach to Signal Detection
The Bayesian approach offers a powerful and flexible framework for signal detection, moving beyond the limitations of classical methods by explicitly incorporating prior knowledge about the probability of signal presence. This approach allows for a more nuanced and informed decision-making process, particularly in situations where prior information is readily available or can be reasonably estimated.
Mathematical Formulation using Bayes’ Theorem
The core of the Bayesian approach lies in Bayes’ theorem, which elegantly connects prior beliefs with observed evidence to update our understanding of the likelihood of a signal. The theorem is expressed as:
P(Signal|Data) = [P(Data|Signal)
P(Signal)] / P(Data)
Where:* P(Signal|Data) is the posterior probability – the probability of a signal being present given the observed data. This is what we want to determine.
- P(Data|Signal) is the likelihood – the probability of observing the data given that a signal is present.
- P(Signal) is the prior probability – the probability of a signal being present before observing any data.
- P(Data) is the evidence – the probability of observing the data, regardless of whether a signal is present. This acts as a normalizing constant.
Let’s illustrate with a numerical example: Imagine a medical test for a rare disease. The prior probability of having the disease (P(Signal)) is 0.01 (1%). The test has a 90% accuracy rate in detecting the disease when it’s present (P(Data|Signal) = 0.9) and a 95% accuracy rate in correctly identifying those without the disease (P(Data|¬Signal) = 0.95). If a person tests positive (Data), what is the posterior probability of them having the disease?
We need to calculate P(Data) using the law of total probability: P(Data) = P(Data|Signal)P(Signal) + P(Data|¬Signal)P(¬Signal) = (0.9
- 0.01) + (0.05
- 0.99) ≈ 0.
- Then, applying Bayes’ theorem: P(Signal|Data) ≈ (0.9
- 0.01) / 0.0585 ≈ 0.15. Even with a positive test result, the posterior probability of having the disease remains relatively low (15%), highlighting the importance of considering prior probabilities.
Comparison with the Neyman-Pearson Approach
The Bayesian and Neyman-Pearson approaches differ significantly in their philosophies and assumptions.
Aspect | Bayesian Approach | Neyman-Pearson Approach |
---|---|---|
Prior Probabilities | Explicitly incorporated into the decision process. | Ignored; only considers likelihood ratio. |
Decision Criterion | Maximizes expected utility or minimizes expected loss. | Controls Type I and Type II error rates. |
Error Rates | Implicitly balanced through the loss function. | Explicitly controlled through significance level (α) and power (1-β). |
Focus | Minimizing overall risk. | Controlling error rates. |
Assumptions | Requires knowledge or estimation of prior probabilities. | Assumes no prior knowledge of probabilities. |
Effect of Prior Probabilities on the ROC Curve
Changes in prior probabilities directly influence the optimal operating point on the Receiver Operating Characteristic (ROC) curve. Imagine an ROC curve with a diagonal line representing random guessing. A higher prior probability of the signal being present will shift the optimal operating point towards a higher sensitivity (true positive rate) even at the cost of a higher false positive rate.
Conversely, a lower prior probability will shift the operating point towards higher specificity (true negative rate). A graphical representation would show multiple ROC curves, each corresponding to a different prior probability, with the optimal operating point shifting along the curve. The curves would all originate from (0,0) and end at (1,1), but the steepness and the optimal point will vary.
Loss Functions in Bayesian Signal Detection, What is signal detection theory
The Bayesian framework incorporates a loss function, which quantifies the cost associated with different types of errors (false positives and false negatives). Different loss functions lead to different decision thresholds. For example, a loss function that heavily penalizes false negatives (e.g., missing a disease diagnosis) will result in a lower decision threshold, increasing sensitivity but also increasing the false positive rate.
Conversely, a loss function that emphasizes minimizing false positives (e.g., in spam filtering, where incorrectly labeling a legitimate email as spam is costly) will lead to a higher decision threshold, prioritizing specificity.
Limitations of the Bayesian Approach
The main limitation of the Bayesian approach is the requirement for accurate prior probabilities. Obtaining reliable prior probabilities can be challenging or even impossible in some situations. If the prior probabilities are inaccurate, the posterior probabilities derived from Bayes’ theorem will also be inaccurate, potentially leading to poor decisions. In cases where prior information is scarce or unreliable, the classical Neyman-Pearson approach might be more appropriate.
Applying the Bayesian Approach to Medical Diagnosis
Consider diagnosing a disease using a medical test. Let’s say:* P(Disease) = 0.02 (prior probability of having the disease)
- P(Positive Test | Disease) = 0.95 (sensitivity)
- P(Positive Test | No Disease) = 0.05 (false positive rate)
A patient receives a positive test result. We want to calculate P(Disease | Positive Test).
1. Calculate P(Positive Test)
P(Positive Test) = P(Positive Test | Disease)P(Disease) + P(Positive Test | No Disease)P(No Disease) = (0.95
- 0.02) + (0.05
- 0.98) = 0.068
- P(Disease)] / P(Positive Test) = (0.95
- 0.02) / 0.068 ≈ 0.28
2. Apply Bayes’ Theorem
P(Disease | Positive Test) = [P(Positive Test | Disease)
Therefore, even with a positive test result, the posterior probability of having the disease is only approximately 28%. This highlights the importance of considering prior probabilities and the test’s accuracy in making informed medical decisions. Further testing or clinical evaluation would likely be necessary.
Signal Detection in Sensory Systems

Signal detection theory (SDT) provides a powerful framework for understanding how we perceive the world around us, moving beyond simple stimulus-response models to encompass the complexities of decision-making under uncertainty. By considering both the sensory information and the decision criteria used to interpret that information, SDT offers valuable insights into the workings of our sensory systems, particularly in auditory and visual perception.The application of SDT to sensory systems reveals the intricate interplay between the physical stimuli, the neural processing of these stimuli, and the subjective experience of perception.
It highlights the fact that perception isn’t a passive reception of sensory input, but rather an active process of interpreting ambiguous information. This process is influenced by factors such as internal noise, attention, and prior expectations.
Auditory Perception and Signal Detection Theory
SDT elegantly explains how we discriminate between sounds in a noisy environment. For example, consider the task of detecting a faint whisper in a crowded room. The whisper itself is the signal, while the background chatter represents the noise. The listener must weigh the sensory evidence (the perceived sound) against the background noise to decide whether the signal is present or absent.
This decision involves a trade-off: sometimes, a listener might perceive a signal where there is only noise (a false alarm), while other times they might miss a real signal (a miss). SDT allows us to quantify these probabilities and understand how factors like the intensity of the whisper and the level of background noise affect the listener’s performance. A listener might adjust their sensitivity (criterion) depending on the context; for example, they might be more likely to report a whisper if they are expecting an important message.
Visual Perception and Signal Detection Theory
Similar principles apply to visual perception. Consider the task of detecting a dim star in a dark night sky. The faint light from the star is the signal, while the ambient light and noise from the observer’s own visual system constitute the noise. Again, the observer must weigh the sensory evidence (the perceived light) against the background noise to decide whether a star is present.
The observer’s decision criterion might be influenced by factors such as the duration of observation or their prior knowledge about the expected location of the star. SDT allows for a precise quantification of the observer’s performance, considering both the sensitivity to the signal and the response bias. For example, an observer might be more inclined to report seeing a star if they are familiar with the constellation it is supposedly part of.
Neural Processing in Signal Detection
Neural processing plays a crucial role in signal detection. Sensory receptors transduce physical stimuli into neural signals, which are then processed through a series of neural stages. Noise, present at every stage of this processing, can interfere with the detection of weak signals. SDT helps us understand how neural mechanisms contribute to the overall sensitivity and bias in signal detection.
For instance, neural adaptation, a phenomenon where the response of neurons decreases over time to a constant stimulus, can affect the detection of weak signals by reducing their salience relative to background noise. Furthermore, attentional mechanisms can modulate neural activity, enhancing the processing of relevant signals and filtering out irrelevant noise. This neural modulation influences the decision criterion and ultimately affects the observer’s performance in signal detection tasks.
The internal noise itself might be modeled as the variability in the neural response to a constant stimulus.
Neurobiological Basis of Signal Detection
Signal detection theory, while a powerful framework for understanding decision-making under uncertainty, gains significant depth when considered through the lens of its underlying neurobiological mechanisms. Understanding how the brain processes sensory information, allocates attention, and makes decisions is crucial for a complete appreciation of signal detection processes. This section delves into the neural underpinnings of signal detection, exploring the intricate interplay of brain regions and neural activity that allows us to discern signals from noise.
Sensory Processing
Sensory information, whether visual, auditory, or somatosensory, undergoes a series of complex transformations as it travels from sensory receptors to the cortex. For instance, in the visual system, light is initially transduced into electrical signals by photoreceptor cells in the retina. These signals are then processed through a series of retinal ganglion cells, relayed via the lateral geniculate nucleus (LGN) of the thalamus, and finally projected to the primary visual cortex (V1).
Within V1, feature extraction occurs, with specialized neurons responding selectively to specific orientations, spatial frequencies, and other visual features. This pre-attentive processing allows for the initial identification of potential signals, even before conscious awareness. Neurotransmitters such as glutamate, acting on AMPA and NMDA receptors, play a critical role in these excitatory processes. Inhibitory neurotransmitters like GABA also modulate activity, shaping the signal and refining its representation.
Similar pathways, albeit with different receptor types and neurotransmitters, exist for auditory and somatosensory processing.
Attentional Mechanisms
Attention plays a crucial role in filtering relevant information from the background noise. The dorsal attention network, primarily involving the intraparietal sulcus (IPS) and superior parietal lobule (SPL), mediates top-down, goal-directed attention, allowing us to selectively focus on specific stimuli. The ventral attention network, encompassing the temporoparietal junction (TPJ) and frontal eye fields (FEF), is more involved in bottom-up, stimulus-driven attention, orienting our attention to unexpected or salient events.
Neurotransmitters like acetylcholine and norepinephrine are heavily involved in modulating attentional networks, influencing the gain of sensory processing in relevant brain areas. For example, increased norepinephrine release enhances the signal-to-noise ratio, improving signal detection.
Decision-Making Processes
The prefrontal cortex (PFC), particularly the dorsolateral PFC (dlPFC) and ventrolateral PFC (vlPFC), plays a pivotal role in evaluating sensory evidence and formulating a response. The PFC integrates information from various sensory modalities and uses this evidence to make a decision. The parietal cortex, particularly the IPS, is involved in accumulating evidence over time and maintaining a representation of the current state of evidence.
Working memory, crucial for holding information relevant to the decision, is supported by prefrontal activity. Cognitive control mechanisms within the PFC ensure that the decision process is efficient and not unduly influenced by irrelevant information.
Brain Regions Involved in Signal Detection Processes
Brain Region | Role in Signal Detection | Specific Subregions/Networks | Supporting Evidence (e.g., studies, techniques) |
---|---|---|---|
Sensory Cortex (e.g., V1, A1, S1) | Initial sensory processing and feature extraction | Layer IV neurons, orientation columns, tonotopic maps, somatotopic maps | Electrophysiological recordings (single-unit and EEG), fMRI studies showing activity increases during stimulus presentation. Lesion studies demonstrating deficits in sensory processing after cortical damage. |
Parietal Cortex | Spatial attention, integration of sensory information | Intraparietal sulcus (IPS), superior parietal lobule (SPL) | fMRI studies demonstrating increased activity in IPS during spatial attention tasks. Lesion studies showing neglect syndromes after parietal damage. |
Prefrontal Cortex | Decision-making, response selection | Dorsolateral PFC (dlPFC), ventrolateral PFC (vlPFC) | fMRI studies showing increased activity in dlPFC during decision-making tasks. Lesion studies showing impaired decision-making after PFC damage. |
Amygdala | Processing of emotional salience in signal detection | Basolateral amygdala, central amygdala | Studies showing enhanced responses to emotionally salient stimuli. Amygdala lesions can impair detection of fear-relevant stimuli. |
Thalamus | Relay of sensory information | Specific thalamic nuclei (e.g., LGN for vision, MGN for audition) | Lesion studies demonstrating sensory deficits after thalamic damage. Electrophysiological recordings showing relay of sensory information through thalamus. |
Neural Activity and Signal Detection Theory Parameters
Sensitivity (d’)
Neural firing rates directly reflect the sensitivity (d’) in signal detection theory. A larger difference in firing rates between signal-present and signal-absent trials indicates higher sensitivity. Signal-to-noise ratio, a measure of the strength of the signal relative to the background noise in neural activity, is another key determinant of d’. Higher signal-to-noise ratios translate to better signal detection.
Variations in neural activity across different brain regions contribute to overall sensitivity. For example, stronger responses in sensory cortex to the signal and weaker responses to noise contribute to higher d’. Studies using electrophysiological recordings (e.g., EEG, single-unit recordings) have directly linked neural firing rates and signal-to-noise ratios to d’ in various sensory modalities.
Response Bias
Response bias, or the criterion, is influenced by neural activity in brain regions associated with reward processing, risk aversion, and other motivational factors. For example, increased activity in brain regions associated with reward (e.g., nucleus accumbens) might lower the criterion, leading to more liberal responses (more false alarms). Conversely, heightened activity in regions related to punishment or risk aversion (e.g., amygdala) might raise the criterion, resulting in more conservative responses (more misses).
Neurotransmitters such as dopamine, involved in reward processing, and serotonin, linked to mood and impulsivity, play crucial roles in modulating response bias.
Comparative Neurobiology of Signal Detection
Signal detection mechanisms are conserved across many species, reflecting the fundamental importance of this ability for survival. However, the specific neural substrates and their efficiency vary across species. Primates, for example, exhibit more complex cortical processing compared to rodents, leading to potentially higher sensitivity and more refined decision-making. These differences in brain architecture and neural processing reflect evolutionary adaptations to different ecological niches and cognitive demands.
Clinical Implications
Impairments in the neural mechanisms underlying signal detection contribute to a range of neurological and psychiatric disorders. For instance, schizophrenia is often associated with deficits in sensory processing, attention, and decision-making, leading to difficulties in distinguishing relevant signals from background noise. Attention-deficit/hyperactivity disorder (ADHD) is characterized by difficulties in sustaining attention and inhibiting impulsive responses, which directly impact signal detection performance.
Similarly, autism spectrum disorder is often associated with atypical sensory processing and attentional biases. Therapeutic interventions targeting these neural mechanisms, such as cognitive training or pharmacological interventions, hold promise for improving signal detection abilities in these conditions.
Individual Differences in Signal Detection
Signal detection theory, while providing a robust framework for understanding how we discern signals from noise, doesn’t account for the remarkable variability observed in individuals’ performance. This variability highlights the crucial role of individual differences in shaping our ability to detect signals accurately and efficiently. Understanding these differences is essential for optimizing performance in various fields, from medical diagnosis to air traffic control.The performance of an individual in a signal detection task is not solely determined by the inherent properties of the signal and noise.
Instead, a complex interplay of factors contributes to the observed variability. These factors can be broadly categorized into biological predispositions, acquired skills, and cognitive characteristics. The intricate relationship between these factors makes predicting individual performance a challenging yet fascinating area of research.
Age-Related Differences in Signal Detection
Age significantly impacts signal detection capabilities. Studies consistently demonstrate a decline in sensory acuity with age, affecting various sensory modalities like vision and hearing. This decline translates directly into poorer signal detection performance. For example, older adults might experience difficulty distinguishing faint sounds or subtle visual cues compared to younger adults. This isn’t simply a matter of reduced sensitivity; it also reflects changes in neural processing efficiency and cognitive resources.
Older adults may require stronger signals or longer processing times to achieve comparable levels of accuracy.
Experience and Expertise
Experience and expertise dramatically shape signal detection performance. Professionals trained to detect specific signals, such as radiologists interpreting medical images or air traffic controllers monitoring radar screens, consistently outperform novices. This improvement isn’t solely due to enhanced sensory capabilities, but also reflects the development of sophisticated strategies, refined perceptual skills, and the ability to integrate contextual information effectively.
Experts learn to recognize subtle patterns and cues that are often missed by less experienced individuals, effectively enhancing their signal-to-noise ratio.
Cognitive Abilities and Signal Detection
Cognitive abilities, such as attention, working memory, and decision-making skills, significantly influence signal detection performance. Individuals with higher cognitive abilities tend to exhibit better performance, demonstrating superior accuracy and faster response times. For example, individuals with better working memory capacity are better able to maintain relevant information in mind, facilitating the comparison of sensory input with expected signals. Similarly, strong attentional control minimizes distractions and improves focus on relevant cues.
Conversely, cognitive impairments can significantly hinder signal detection performance.
Summary of Individual Differences Affecting Signal Detection
The following factors significantly influence individual differences in signal detection:
- Age: Sensory decline with age leads to reduced sensitivity and slower processing speed, impacting signal detection accuracy.
- Experience: Expertise and training enhance signal detection through the development of specialized strategies and refined perceptual skills.
- Cognitive Abilities: Stronger attention, working memory, and decision-making skills contribute to improved accuracy and faster response times.
- Motivation and Alertness: Individual differences in motivation and alertness can significantly impact performance, influencing both response bias and accuracy.
- Genetic Factors: Genetic predispositions might influence sensory sensitivity and neural processing efficiency, indirectly impacting signal detection.
Signal Detection and Attention
The intricate dance between signal detection and attention reveals how our cognitive resources shape our perception of the world. Understanding this relationship is crucial for comprehending how we filter information, prioritize stimuli, and make decisions in a constantly changing environment. Attention acts as a spotlight, focusing our cognitive resources on specific aspects of our sensory input, profoundly impacting our ability to detect relevant signals amidst background noise.Attention significantly enhances signal detection performance.
By selectively focusing on relevant stimuli, we improve our sensitivity to those signals and reduce the influence of distracting information. This selective filtering process allows us to efficiently process information, preventing sensory overload and enabling us to respond effectively to important events. The efficiency of this process can vary based on the individual’s level of alertness, the nature of the task, and the complexity of the environment.
Selective Attention’s Influence on Signal Detection
Selective attention acts as a filter, enhancing the detection of attended stimuli while suppressing the processing of unattended ones. This filtering process is not absolute; unattended stimuli can still exert some influence, particularly when they are highly salient or emotionally significant. Consider a cocktail party effect: While focusing on a conversation, we can still detect our name being mentioned from across the room.
This demonstrates that even when attention is focused elsewhere, important stimuli can break through the filter, highlighting the dynamic interplay between attention and signal detection. The strength of this filtering process can vary considerably depending on individual differences, task demands, and environmental factors. For instance, someone highly focused on a task might be less susceptible to distractions, resulting in improved signal detection performance.
Experimental Paradigms Investigating Attention and Signal Detection
Several experimental paradigms effectively probe the relationship between attention and signal detection. One such paradigm is the visual search task, where participants must locate a target stimulus amongst distractors. The difficulty of this task, and consequently the accuracy of signal detection, varies depending on the features of the target and the similarity to distractors. If the target is highly salient and dissimilar from distractors, detection is rapid and accurate.
Conversely, if the target is similar to distractors, search time increases and detection accuracy decreases, illustrating the role of attention in filtering relevant from irrelevant information.Another powerful paradigm is the dichotic listening task, where participants listen to different auditory messages presented simultaneously to each ear. They are instructed to attend to one message and ignore the other. While performance on the attended message is typically high, participants often fail to detect meaningful information in the unattended message, further supporting the selective nature of attention in signal detection.
Variations in this task, such as introducing a highly salient stimulus in the unattended ear (e.g., the participant’s name), allow researchers to study the limits of attentional filtering and the impact of unexpected or highly relevant stimuli on signal detection.
Future Directions in Signal Detection Theory
Signal detection theory (SDT), a powerful framework for understanding decision-making under uncertainty, continues to evolve and expand its reach into diverse fields. This review explores promising avenues for future research, focusing on advancements in Bayesian SDT, the development of robust models for high-dimensional data, the integration of cognitive factors, and the modeling of dynamic environments. These advancements promise to significantly enhance the applicability and predictive power of SDT across various disciplines.
Advancements in Bayesian Signal Detection Theory
Bayesian SDT, which incorporates prior knowledge and uncertainty into signal detection models, has witnessed significant progress. This approach allows for a more nuanced understanding of decision-making by accounting for individual differences in prior beliefs and the inherent uncertainty associated with sensory information. In neuroscience, Bayesian SDT has proven particularly valuable in modeling sensory perception and decision-making processes. For instance, studies have shown improved accuracy in predicting perceptual judgments by incorporating prior knowledge about stimulus probabilities.
In machine learning, Bayesian SDT has been successfully applied to anomaly detection and classification tasks, achieving improved accuracy rates and reduced false positive rates compared to traditional methods. For example, in medical imaging, Bayesian models can integrate prior knowledge about disease prevalence to improve the detection of subtle anomalies, leading to more accurate diagnoses. However, challenges remain, particularly in effectively incorporating complex prior knowledge and managing computational complexity in high-dimensional datasets.
The development of efficient algorithms and approximation techniques will be crucial for advancing the application of Bayesian SDT.
Potential Areas for Future Research and Development
Three key areas hold immense potential for future research in SDT: handling high-dimensional data, incorporating cognitive factors, and modeling dynamic environments.
Robust Signal Detection Models for High-Dimensional Data
High-dimensional datasets, such as those generated by fMRI or social network analysis, present unique challenges for signal detection. The “curse of dimensionality” – the exponential increase in computational complexity with increasing dimensionality – necessitates the development of novel model architectures and algorithms. Research questions include: How can dimensionality reduction techniques be optimally integrated into SDT models? What are the limitations of existing high-dimensional SDT models, and how can these be addressed through innovative approaches such as manifold learning or deep learning?
Potential methodologies include the development of novel dimensionality reduction techniques tailored to the specific characteristics of the data, the exploration of sparse modeling approaches to reduce the number of parameters, and the adaptation of deep learning architectures for signal detection in high-dimensional spaces.
Incorporation of Cognitive Factors into Signal Detection Models
Current SDT models often simplify the cognitive processes involved in signal detection. Integrating cognitive factors such as attention, working memory, and cognitive load into SDT models is crucial for creating more realistic and predictive models of human behavior. Research questions include: How do attentional mechanisms modulate the sensitivity and bias parameters in SDT? How does working memory capacity influence the ability to integrate information across multiple sensory modalities?
Potential methodologies include incorporating cognitive load measures into SDT models, using computational models to simulate the interaction between cognitive processes and signal detection, and conducting behavioral experiments to investigate the effects of cognitive factors on decision-making under uncertainty.
Signal Detection in Dynamic Environments
Many real-world scenarios involve signals and noise that change over time. Developing SDT models that can effectively handle dynamic environments is crucial for applications such as tracking moving objects, monitoring physiological signals, and analyzing time-series data. Research questions include: How can temporal dependencies be incorporated into SDT models? What are the optimal methods for adapting decision criteria in dynamic environments?
Potential methodologies include using state-space models to represent the temporal dynamics of signals and noise, applying recurrent neural networks to learn temporal patterns, and developing online learning algorithms that adapt to changing environmental conditions.
A Complex Signal Detection Scenario: Anomaly Detection in Medical Imaging
Consider the detection of a small cancerous tumor in a noisy medical image (e.g., a mammogram). The signal (tumor) is subtle, characterized by a slight increase in tissue density compared to the surrounding tissue (noise). The decision criterion involves setting a threshold for the pixel intensity values. A high threshold minimizes false positives (identifying benign tissue as cancerous), but increases false negatives (missing actual tumors).
A low threshold increases sensitivity (detecting more tumors), but at the cost of increased false positives. The trade-off between sensitivity and specificity is crucial. A false negative could lead to delayed treatment and reduced survival rates, while a false positive results in unnecessary biopsies, anxiety, and medical expenses. The optimal decision criterion balances the costs associated with false positives and false negatives, potentially incorporating prior probabilities of cancer based on patient risk factors and the costs of each type of error.
Quantifying this trade-off requires careful consideration of the relative costs of false positives and false negatives, which may vary depending on the specific clinical context.
Table of Current Research Trends
Research Trend | Description | Key Researchers/Groups | Open Questions |
---|---|---|---|
Bayesian Signal Detection Theory | Incorporates prior knowledge and uncertainty into signal detection models. | (This requires specific researchers and groups, which are beyond the scope of this response. A literature search would provide appropriate names.) | How to effectively incorporate complex and uncertain prior knowledge? How to efficiently handle high-dimensional prior distributions? |
High-Dimensional Signal Detection | Addresses challenges in detecting signals in high-dimensional datasets. | (This requires specific researchers and groups, which are beyond the scope of this response. A literature search would provide appropriate names.) | How to effectively mitigate the curse of dimensionality? How to develop robust feature selection methods? |
Signal Detection in Dynamic Systems | Models signal detection in environments where signals and noise change over time. | (This requires specific researchers and groups, which are beyond the scope of this response. A literature search would provide appropriate names.) | How to effectively model long-range temporal dependencies? How to handle non-stationarity in signals and noise? |
A Specific Research Question
How can we develop more robust signal detection models that are less susceptible to adversarial attacks, particularly in the context of cybersecurity applications where malicious actors may intentionally try to mask signals or introduce noise?
Potential Methodologies for Future Research
The development of robust and accurate signal detection models will benefit from a multi-faceted approach.
- Computational modeling using Bayesian networks allows for explicit representation of uncertainty and prior knowledge.
- Machine learning algorithms, such as Support Vector Machines and deep learning models, offer powerful tools for pattern recognition and classification in high-dimensional datasets.
- Behavioral experiments with human participants provide valuable insights into the cognitive processes underlying signal detection.
- Simulation studies allow for controlled investigation of the effects of different model parameters and environmental conditions.
Query Resolution: What Is Signal Detection Theory
Can signal detection theory be used to analyze decisions made by non-human systems?
Absolutely! Signal detection theory is applicable to any system that makes decisions based on noisy information, including automated systems like radar, spam filters, and even AI algorithms.
What are some ethical considerations when applying signal detection theory?
Understanding bias is crucial. A biased system might lead to unfair or discriminatory outcomes. For example, a medical diagnostic tool with a high false positive rate for a specific demographic could lead to unnecessary treatment and anxiety.
How does signal detection theory relate to Bayesian inference?
Both deal with making decisions under uncertainty. Bayesian inference incorporates prior probabilities into the decision-making process, while classical signal detection theory often focuses on the likelihood ratio. However, they are compatible and can be combined.
Is signal detection theory only relevant for sensory perception?
No, it applies to any situation where a decision is made based on noisy information, including cognitive tasks, financial decisions, and even social judgments.