What is knowledge based theory pdf – What is knowledge-based theory PDF? This question introduces a field exploring how knowledge is structured, represented, and utilized, particularly within artificial intelligence and expert systems. Understanding knowledge-based theory requires examining diverse knowledge types—from explicit facts to tacit skills—and how these interact in decision-making. This exploration delves into the historical development, key principles, and practical applications of this influential theoretical framework.
The core of knowledge-based theory lies in its attempt to formalize and represent human expertise in a way that can be used by computers. This involves identifying, classifying, and representing different types of knowledge (e.g., declarative, procedural, tacit), exploring methods for acquiring this knowledge (e.g., knowledge engineering, machine learning), and developing systems (e.g., expert systems) capable of using this knowledge to solve problems and make decisions.
The implications extend far beyond theoretical computer science, influencing fields like organizational learning, knowledge management, and even the design of ethical AI systems.
Defining Knowledge-Based Theory
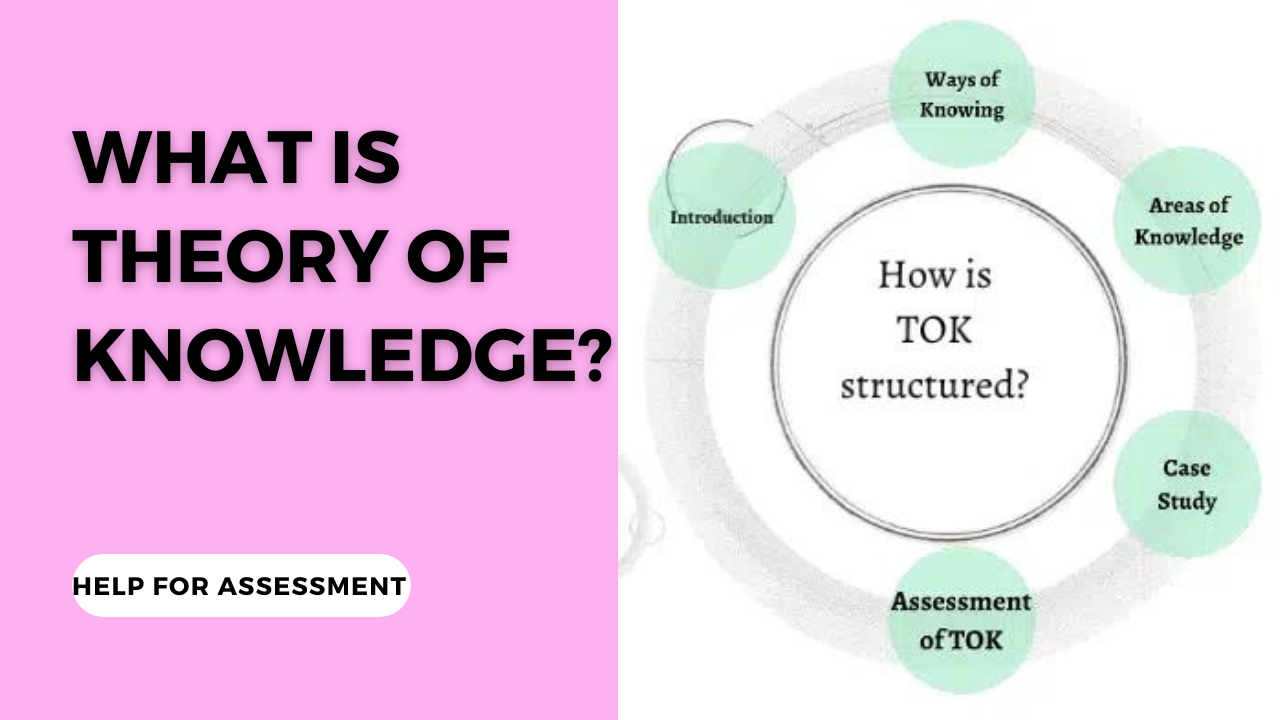
Knowledge-based theory, in its simplest form, posits that knowledge is the fundamental building block of human action and social structures. It emphasizes the role of knowledge in shaping individual beliefs, influencing decision-making processes, and ultimately driving societal change. This theory moves beyond simply acknowledging the existence of knowledge to exploring its creation, dissemination, and impact on various aspects of human life.Knowledge-based theory rests on several core principles.
Firstly, it highlights the active and constructive nature of knowledge acquisition. Knowledge is not passively received but actively built through experience, interpretation, and interaction with the environment. Secondly, the theory underscores the social dimension of knowledge. Knowledge is not solely an individual possession but is co-created, shared, and negotiated within social contexts. Thirdly, knowledge is seen as contextual and situated, meaning its meaning and value depend heavily on the specific circumstances in which it is used.
Finally, the theory acknowledges the inherent limitations and potential biases within knowledge systems. Knowledge is not objective truth but a reflection of the perspectives and experiences of those who create and utilize it.
Core Principles of Knowledge-Based Theory
The core principles of knowledge-based theory provide a framework for understanding how knowledge shapes human behavior and social systems. The active construction of knowledge, for example, challenges the notion of knowledge as a static body of facts. Instead, it emphasizes the dynamic process of learning and knowledge creation, highlighting the role of individual interpretation and experience. The social dimension of knowledge highlights the collaborative nature of knowledge production and dissemination.
This principle emphasizes the importance of communication, interaction, and shared understanding in building collective knowledge. The contextual and situated nature of knowledge acknowledges that knowledge is not universally applicable but rather its meaning and relevance are shaped by the specific context in which it is used. Finally, the recognition of inherent limitations and biases in knowledge systems encourages critical evaluation and a nuanced understanding of the knowledge we use to make decisions and shape our understanding of the world.
Historical Development of Knowledge-Based Theory
The development of knowledge-based theory can be traced through several key intellectual movements. Early philosophical inquiries into epistemology, the study of knowledge, laid the groundwork for exploring the nature and origins of knowledge. The Enlightenment, with its emphasis on reason and empirical observation, contributed to a focus on the verifiable and systematic acquisition of knowledge. The rise of sociology and anthropology in the 19th and 20th centuries further enriched the understanding of knowledge as a social construct, shaped by cultural norms and social interactions.
The development of cognitive science and information science in the latter half of the 20th century provided new tools and perspectives for analyzing the processes of knowledge acquisition, representation, and use. More recently, the rise of social constructivism and situated cognition has further emphasized the social and contextual nature of knowledge. The evolution of knowledge-based theory reflects a gradual shift from viewing knowledge as an objective, universal entity to understanding it as a dynamic, socially constructed, and contextually situated phenomenon.
Types of Knowledge in Knowledge-Based Theory
Knowledge-based theory posits that knowledge is a crucial element driving individual and organizational success. Understanding the different types of knowledge and their characteristics is essential for effectively managing, leveraging, and creating knowledge within any context. This section will delve into the various classifications of knowledge, examining their properties and roles in decision-making processes.
Categorization of Different Knowledge Types
The following Artikels five distinct types of knowledge within the Knowledge-Based Theory framework, each with its own unique characteristics and applications. The categorization presented here is not exhaustive, as the field of knowledge management continues to evolve, but it provides a solid foundation for understanding the diverse forms knowledge can take.
- Tacit Knowledge: This refers to the implicit, subjective, and often difficult-to-articulate knowledge embedded in individual experience and intuition. It’s often described as “knowing-how” rather than “knowing-that.” It’s highly personal and context-dependent.
- Explicit Knowledge: This is the opposite of tacit knowledge; it’s readily articulated, formalized, and easily communicated and shared. It’s often documented in manuals, databases, or other formal systems. It’s objective and easily transferable.
- Procedural Knowledge: This refers to knowledge of how to perform a task or process. It involves a sequence of steps or actions, often learned through practice and repetition. It’s closely related to skills and abilities.
- Declarative Knowledge: This is factual knowledge, often expressed as “knowing-that.” It encompasses facts, concepts, and principles. It’s typically easy to represent and communicate.
- Embodied Knowledge: This type of knowledge is deeply intertwined with the physical body and its experiences. It involves skills and understanding developed through physical practice and sensory experience. It’s often difficult to separate from the individual’s physical capabilities.
Real-World Examples of Knowledge Types
The following table provides real-world examples of each knowledge type in professional settings:
Knowledge Type | Example | Professional Setting |
---|---|---|
Tacit Knowledge | A surgeon’s intuitive understanding of how to perform a complex operation based on years of experience. | Healthcare |
Tacit Knowledge | A software engineer’s instinctive ability to debug complex code quickly and efficiently. | Software Engineering |
Explicit Knowledge | A company’s documented safety procedures. | Manufacturing |
Explicit Knowledge | A medical textbook detailing the symptoms and treatments for various diseases. | Healthcare |
Procedural Knowledge | A pilot’s knowledge of how to fly a plane, encompassing a series of steps and procedures. | Aviation |
Procedural Knowledge | An accountant’s ability to prepare tax returns accurately and efficiently. | Accounting |
Declarative Knowledge | The principles of thermodynamics. | Engineering |
Declarative Knowledge | Knowledge of marketing principles and strategies. | Business |
Embodied Knowledge | A musician’s ability to play an instrument skillfully. | Music |
Embodied Knowledge | An athlete’s refined physical skills and coordination. | Sports |
Comparison of Knowledge Type Characteristics
This table compares three key knowledge types across several characteristics:
Knowledge Type | Accessibility | Transferability | Formalization | Representation |
---|---|---|---|---|
Tacit Knowledge | Difficult | Difficult | Low | Implicit, experiential |
Explicit Knowledge | Easy | Easy | High | Formal documents, databases |
Procedural Knowledge | Moderate | Moderate | Moderate | Step-by-step instructions, simulations |
Advantages and Disadvantages of Knowledge Types
- Tacit Knowledge:
- Advantages: Highly nuanced, context-specific, allows for quick, intuitive responses.
- Disadvantages: Difficult to articulate, share, and codify; vulnerable to loss upon departure of knowledge holders.
- Explicit Knowledge:
- Advantages: Easily shared, stored, and retrieved; facilitates standardization and consistency.
- Disadvantages: Can be inflexible, out-of-date, and may not capture the full richness of experience.
- Procedural Knowledge:
- Advantages: Allows for efficient task performance; can be easily trained and replicated.
- Disadvantages: May not adapt well to changing circumstances; can be overly rigid.
Influence of Knowledge Types on Decision-Making
A case study of a pharmaceutical company developing a new drug will be used to illustrate how tacit and explicit knowledge influence decision-making.
- Problem Identification: The initial identification of a need for a new drug relies heavily on explicit knowledge (e.g., epidemiological data, scientific literature) and tacit knowledge (e.g., researchers’ intuition about potential drug targets).
- Solution Generation: The design and development of the drug itself involves a complex interplay of both types of knowledge. Explicit knowledge (e.g., chemical formulas, pharmacological principles) guides the process, while tacit knowledge (e.g., researchers’ experience in drug design) is crucial for innovation and problem-solving.
- Evaluation: The evaluation of the drug’s efficacy and safety involves both explicit knowledge (e.g., clinical trial data, regulatory guidelines) and tacit knowledge (e.g., clinicians’ interpretation of results).
Interaction Between Knowledge Types in Decision-Making
The interaction between tacit and explicit knowledge is crucial for effective decision-making. Nonaka and Takeuchi’s SECI model (Socialization, Externalization, Combination, Internalization) (Nonaka & Takeuchi, 1995) highlights how tacit knowledge can be converted into explicit knowledge (externalization) and then re-embedded into practice (internalization). A successful integration of these knowledge types enhances decision-making by combining the richness of experience with the clarity and shareability of formal knowledge.
Conversely, a lack of effective knowledge conversion can hinder decision-making, leading to suboptimal choices or missed opportunities.
Summary of Key Differences and Interrelationships
The various types of knowledge—tacit, explicit, procedural, declarative, and embodied—possess unique characteristics impacting their accessibility, transferability, and role in decision-making. While explicit knowledge provides a foundation for structured approaches, tacit knowledge offers crucial intuition and expertise. Effective decision-making requires a synergistic integration of these diverse knowledge types, facilitated by processes that bridge the gap between implicit understanding and explicit articulation.
Knowledge Representation and Acquisition
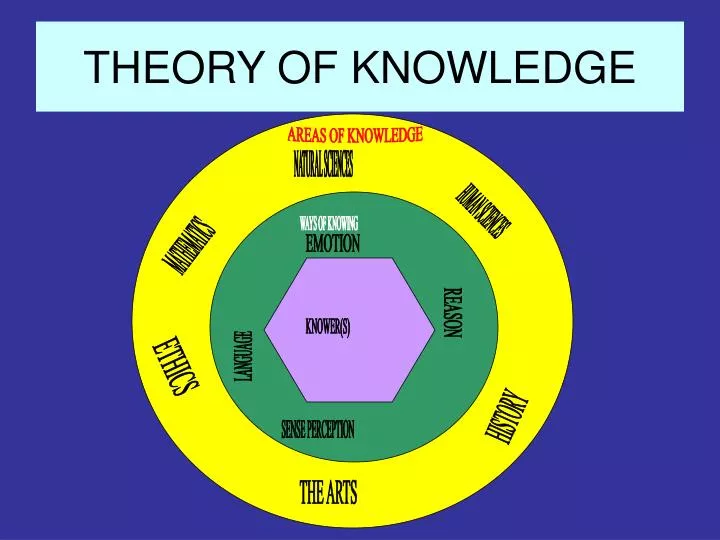
Knowledge representation and acquisition are crucial aspects of knowledge-based systems. Effective representation allows for efficient storage and retrieval of knowledge, while robust acquisition methods ensure the system’s knowledge base remains accurate and up-to-date. This section explores various techniques for representing and acquiring knowledge within a knowledge-based system.
Semantic Networks
Semantic networks represent knowledge as a graph of interconnected nodes and relationships. This approach is particularly useful for representing hierarchical relationships and taxonomic classifications. The following table illustrates a semantic network representing various fruits, their attributes, and relationships.
Node | Relationship | Target Node |
---|---|---|
Apple | is a | Fruit |
Apple | has color | Red |
Apple | has taste | Sweet |
Apple | originates in | Central Asia |
Banana | is a | Fruit |
Banana | has color | Yellow |
Banana | has taste | Sweet |
Banana | originates in | Southeast Asia |
Orange | is a | Fruit |
Orange | has color | Orange |
Orange | has taste | Sweet and Tart |
Orange | originates in | Southeast Asia |
Frames
Frames represent knowledge as structured data objects with slots for attributes and values. This approach is well-suited for representing objects with well-defined properties and relationships. The following example shows a frame representation for a “Car” object.“`Car: make: “Toyota” model: “Camry” year: 2023 color: “Silver” engineType: “4-cylinder”“`
Knowledge Acquisition – Rule-Based Systems
Rule-based systems acquire knowledge through the creation of production rules, which are IF-THEN statements that map conditions to actions. These rules form the core of the expert system’s reasoning engine. The following are three production rules for a car engine diagnosis expert system:
- IF engine won’t start AND battery is dead THEN replace battery
- IF engine is overheating AND coolant level is low THEN add coolant
- IF engine is making strange noises AND oil level is low THEN check oil level and add oil if needed
Knowledge Acquisition – Case-Based Reasoning
Case-based reasoning (CBR) is a knowledge acquisition technique that uses past experiences (cases) to solve new problems. A scenario where CBR would be suitable is diagnosing medical conditions. A new patient’s symptoms would be compared to existing cases in a database of similar cases. The system would then retrieve the most similar cases, adapt their solutions to the new situation, and propose a diagnosis and treatment plan.
The similarity is usually determined by a metric comparing symptoms and other relevant patient data.
Knowledge Integration
Integrating knowledge from multiple sources requires a systematic approach to ensure consistency and avoid conflicts. A strategy for integrating knowledge from a database, a text document, and a set of rules could involve the following steps:
- Data Extraction: Extract relevant information from each source. For the database, this involves querying; for the text document, it involves natural language processing (NLP) techniques; for the rules, it involves parsing.
- Data Transformation: Transform the extracted data into a unified format suitable for the knowledge base. This may involve data cleaning, normalization, and ontology mapping.
- Conflict Resolution: Develop mechanisms to resolve inconsistencies or conflicts between the sources. This might involve prioritizing information based on source reliability or using weighted averaging techniques.
- Knowledge Base Population: Populate the knowledge base with the integrated information, ensuring data integrity and consistency.
- Validation and Refinement: Validate the integrated knowledge base through testing and refine it based on feedback.
Specific Domain Knowledge Representation
A library catalog system can be effectively represented using a frame-based approach. The following table Artikels the structure:
Frame | Slot | Value Type | Example |
---|---|---|---|
Book | title | String | “The Lord of the Rings” |
Book | author | String | “J.R.R. Tolkien” |
Book | ISBN | String | “978-0618002255” |
Book | genre | String | “Fantasy” |
Member | memberID | Integer | 12345 |
Member | name | String | “John Doe” |
Member | address | String | “123 Main St” |
Loan | bookID | Integer | 67890 |
Loan | memberID | Integer | 12345 |
Loan | loanDate | Date | 2024-03-08 |
Loan | dueDate | Date | 2024-03-22 |
Knowledge Acquisition Techniques Comparison
The following table compares three knowledge acquisition techniques:
Technique | Description | Advantages | Disadvantages |
---|---|---|---|
Knowledge Engineering | Eliciting knowledge from human experts through interviews and observations. | High accuracy, deep understanding of domain. | Time-consuming, expensive, relies on expert availability. |
Machine Learning | Automatically learning knowledge from data using algorithms. | Scalable, can handle large datasets, automates knowledge acquisition. | Requires large amounts of data, can be prone to errors, difficult to interpret results. |
Ontology Engineering | Developing formal representations of knowledge using ontologies. | Facilitates knowledge sharing and reuse, improves interoperability. | Requires expertise in ontology design and development, can be complex. |
Knowledge-Based Systems and Applications

Knowledge-based systems (KBS) represent a significant advancement in artificial intelligence, leveraging explicitly encoded knowledge to solve complex problems and provide expert-level reasoning capabilities. These systems move beyond simple rule-based systems by incorporating diverse forms of knowledge, including facts, rules, heuristics, and case-based reasoning, enabling them to tackle a wider range of applications across various domains. Their effectiveness hinges on the quality and organization of the knowledge base, as well as the inference engine used to process this information.Knowledge-based systems find practical applications in diverse fields, demonstrating their versatility and impact on numerous industries.
The successful deployment of a KBS requires careful consideration of the specific domain, the nature of the knowledge required, and the appropriate knowledge representation techniques. The following examples illustrate the breadth of KBS applications and their potential benefits.
Unlocking the power of knowledge starts with understanding what is knowledge based theory pdf, its core principles, and practical applications. To truly master this, consider how a robust system enhances your learning; explore the innovative design of a smart knowledge base to see how technology can amplify your knowledge base theory understanding. Ultimately, grasping what is knowledge based theory pdf empowers you to build and leverage efficient, effective knowledge systems.
Real-World Applications of Knowledge-Based Systems
The application of knowledge-based systems spans a wide array of domains. The table below provides a structured overview of several key examples, highlighting the application area, the specific domain, and the type of knowledge utilized.
Application | Domain | Knowledge Type | Example |
---|---|---|---|
Medical Diagnosis | Healthcare | Rules, Case-based reasoning, Probabilistic knowledge | MYCIN (an early expert system for diagnosing bacterial infections) utilized rules and probabilistic reasoning based on patient symptoms and lab results. Modern systems incorporate machine learning to refine diagnostic accuracy. |
Financial Risk Assessment | Finance | Statistical data, Financial models, Rules | Credit scoring systems use knowledge-based approaches to assess the creditworthiness of loan applicants, combining financial data with rules and statistical models to predict the likelihood of default. |
Chemical Compound Identification | Chemistry | Structural formulas, Chemical properties, Reaction mechanisms | Systems can identify unknown compounds based on spectral data (e.g., mass spectrometry, NMR) by comparing the data to a knowledge base of known compounds and their properties. |
Fault Diagnosis in Manufacturing | Manufacturing | Sensor data, Process models, Expert rules | Systems monitor sensor data from machinery and use knowledge of the manufacturing process to diagnose potential faults and suggest corrective actions, minimizing downtime and improving efficiency. |
Legal Case Analysis | Law | Legal precedents, Statutes, Case summaries | Systems can assist legal professionals by analyzing case facts and comparing them to relevant legal precedents and statutes, aiding in legal research and strategy development. |
Advantages and Limitations of Knowledge-Based Systems
Knowledge-based systems offer several advantages, but also present certain limitations that must be carefully considered.Advantages include:* Improved Decision-Making: KBS can provide expert-level advice and support, leading to better and more consistent decisions, especially in complex situations where human expertise might be limited or unavailable.
Increased Efficiency and Productivity
Automating tasks and processes through KBS can significantly improve efficiency and productivity, freeing up human experts to focus on more strategic activities.
Enhanced Consistency and Accuracy
KBS eliminate inconsistencies and errors associated with human judgment, ensuring consistent application of knowledge and improved accuracy in decision-making.
Knowledge Preservation and Transfer
KBS help preserve and transfer valuable expert knowledge, especially in situations where experienced professionals are retiring or leaving the organization.
Accessibility to Expertise
KBS can make expert knowledge readily accessible to a wider audience, even those without specialized training.Limitations include:* Knowledge Acquisition Bottleneck: Eliciting and representing expert knowledge can be a time-consuming and challenging process, often requiring significant interaction with domain experts.
Knowledge Maintenance
Keeping the knowledge base up-to-date and accurate requires ongoing maintenance and updates, as new information becomes available or existing knowledge becomes obsolete.
Brittleness
KBS can be brittle, meaning they may perform poorly or fail completely when presented with inputs that are outside the scope of their knowledge base.
Explanation Difficulty
Understanding the reasoning process of some KBS can be difficult, making it challenging to explain the system’s conclusions and gain user trust.
Cost of Development and Implementation
Developing and implementing KBS can be expensive, requiring specialized expertise and significant investment in software and hardware.
Knowledge Management and Organizational Learning
Knowledge-based theory posits that organizational success hinges on the effective creation, dissemination, and application of knowledge. This inherently links it to organizational learning, a process by which organizations acquire, share, and use knowledge to improve their performance and adapt to change. Essentially, a strong knowledge management system is the engine driving organizational learning.The relationship between knowledge-based theory and organizational learning is symbiotic.
Knowledge-based theory provides the framework for understanding how knowledge is created, stored, and used within an organization. Organizational learning, in turn, provides the context for applying this knowledge to achieve strategic goals and enhance organizational capabilities. Effective knowledge management practices are crucial for fostering a learning organization, enabling it to continuously adapt and innovate. Without effective knowledge management, valuable knowledge may remain siloed, untapped, or even lost, hindering the organization’s learning process and competitive advantage.
Strategies for Managing Knowledge Within an Organization
Effective knowledge management requires a multifaceted approach encompassing several key strategies. These strategies aim to capture, codify, disseminate, and utilize knowledge effectively throughout the organization. A holistic strategy addresses both explicit and tacit knowledge, recognizing the importance of both in organizational success.
- Knowledge Creation: This involves actively encouraging the generation of new knowledge through initiatives like brainstorming sessions, research and development projects, and employee training programs. The organization should establish a culture that values knowledge creation and rewards innovative thinking. Examples include establishing dedicated innovation labs or implementing suggestion schemes with clear reward mechanisms.
- Knowledge Capture: This focuses on systematically collecting and documenting existing knowledge. Methods include creating knowledge repositories (databases, wikis), conducting knowledge interviews with experts, and using content management systems to archive crucial documents and projects. A well-defined process for capturing lessons learned from projects is also critical.
- Knowledge Codification: This involves transforming tacit knowledge (often implicit and difficult to articulate) into explicit knowledge (easily documented and shared). This can involve creating manuals, tutorials, or standard operating procedures. Mentorship programs and knowledge-sharing platforms are vital tools for codifying tacit knowledge.
- Knowledge Dissemination: This ensures that knowledge is readily accessible to those who need it. Effective strategies include establishing internal knowledge networks, utilizing collaborative tools, and implementing knowledge sharing platforms. Regular training sessions and workshops also contribute to knowledge dissemination.
- Knowledge Application: This involves ensuring that captured and codified knowledge is actually used to improve organizational processes and decision-making. This requires creating a culture of learning and continuous improvement, where employees are encouraged to apply new knowledge and share their experiences.
Knowledge Management Process Flowchart
The knowledge management process can be visualized as a cyclical flow, continuously improving and adapting.
A simplified representation: Knowledge Creation → Knowledge Capture → Knowledge Codification → Knowledge Dissemination → Knowledge Application → Knowledge Evaluation → (repeat)
Imagine a flowchart where each stage above is a box connected by arrows indicating the flow. The “Knowledge Evaluation” box would feed back into “Knowledge Creation,” showing the iterative nature of the process. For instance, evaluation might reveal gaps in knowledge or suggest improvements to existing processes, leading to new knowledge creation and the cycle restarting. The flowchart visually depicts the continuous feedback loop inherent in effective knowledge management, ensuring continuous improvement and adaptation.
Knowledge Sharing and Collaboration
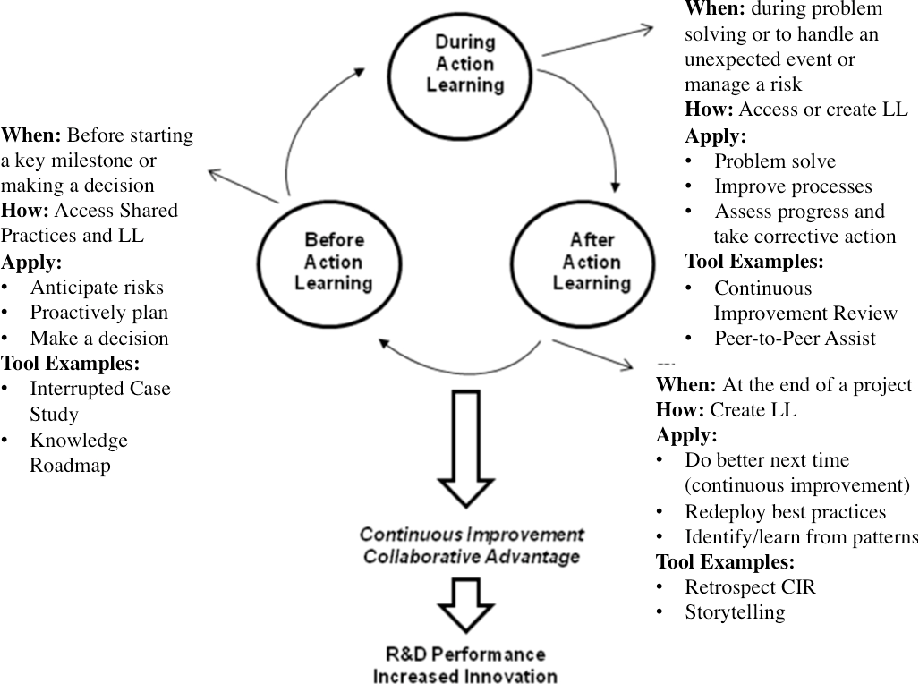
Effective knowledge sharing and collaboration are crucial for organizational success. A knowledge-based organization thrives on the seamless flow of information and expertise among its members, fostering innovation, problem-solving, and improved decision-making. However, several obstacles hinder this process, requiring careful consideration and strategic implementation of best practices.Knowledge sharing, while theoretically beneficial, faces significant challenges in many organizational contexts. These challenges stem from a variety of sources, including individual, group, and organizational factors.
Challenges of Knowledge Sharing in Organizations
Several factors impede the effective sharing of knowledge within organizations. These include issues related to individual attitudes and behaviors, such as the reluctance to share tacit knowledge (knowledge that is difficult to articulate or codify) due to perceived loss of competitive advantage or fear of being replaced. Group dynamics also play a role; established silos and departmental rivalries can create barriers to communication and collaboration.
Organizational culture, if not supportive of knowledge sharing, can actively discourage it through a lack of incentives or reward systems that recognize knowledge contribution. Furthermore, inadequate technology infrastructure or poorly designed knowledge management systems can make it difficult to access and share information effectively. Finally, the sheer volume of information within large organizations can overwhelm individuals and impede their ability to find and use relevant knowledge.
Best Practices for Facilitating Effective Knowledge Sharing
Overcoming the challenges of knowledge sharing requires a multi-faceted approach. This includes fostering a culture of trust and openness where employees feel comfortable sharing their knowledge without fear of reprisal. Incentivizing knowledge sharing through rewards and recognition programs is also crucial. Establishing clear channels for knowledge dissemination, such as communities of practice, mentoring programs, and regular knowledge-sharing sessions, can facilitate the flow of information.
Furthermore, providing training on effective communication and collaboration skills can empower employees to share their knowledge more effectively. Regularly evaluating and improving knowledge management systems is essential to ensure they are user-friendly and meet the needs of the organization.
The Role of Technology in Knowledge Sharing and Collaboration
Technology plays a vital role in facilitating knowledge sharing and collaboration. Knowledge management systems (KMS) provide a centralized repository for storing and accessing organizational knowledge. These systems can include databases, wikis, and document management systems. Collaboration platforms, such as enterprise social networks and project management tools, enable teams to work together on projects, share files, and communicate effectively.
These tools often integrate features such as instant messaging, video conferencing, and file sharing, streamlining the collaboration process. Furthermore, the use of learning management systems (LMS) allows for the dissemination of training materials and the tracking of employee learning progress. The implementation of these technologies, however, requires careful planning and integration with existing organizational systems to ensure their effective use.
For example, a poorly designed KMS can be more of a hindrance than a help, leading to information overload and difficulty in retrieving relevant knowledge. Effective training and ongoing support are essential to maximize the benefits of these technological tools.
Expert Systems and Knowledge Engineering
Expert systems represent a significant application of knowledge-based theory, embodying the principles of knowledge representation, inference, and reasoning to mimic the decision-making capabilities of human experts. This section delves into the development and application of expert systems, exploring the crucial roles of knowledge engineers and the challenges inherent in building and deploying these systems.
Expert System Development Principles
Expert system development involves a systematic process of knowledge acquisition, representation, and implementation. A core component is the inference engine, which uses the represented knowledge to deduce conclusions and provide solutions. Several types of inference engines exist, each with its own strengths and weaknesses.
- Forward Chaining: This data-driven approach starts with known facts and applies rules sequentially to reach a conclusion. It’s suitable for situations where the initial data is readily available and the goal is to determine all possible consequences. For example, a diagnostic system might use forward chaining to identify potential diseases based on a patient’s symptoms. Its strength lies in its efficiency in exploring all possible outcomes, while its weakness is its potential inefficiency when dealing with a large number of rules and facts, leading to combinatorial explosion.
- Backward Chaining: This goal-driven approach starts with a hypothesis (goal) and works backward, searching for rules and facts that support or refute it. It’s efficient when the number of possible goals is limited and the goal is clearly defined. For example, a medical diagnosis system might use backward chaining to determine if a patient has a specific disease based on a set of symptoms.
Its strength lies in its efficiency in focusing on relevant rules and facts, while its weakness is its inability to discover unexpected consequences or relationships not directly related to the initial goal.
- Model-Based Reasoning: This approach uses a model of the system or process being analyzed to simulate its behavior and make predictions. It is particularly useful when dealing with complex systems with multiple interacting components. For instance, a system monitoring a chemical plant might use model-based reasoning to predict the outcome of different operational scenarios. Its strength is its ability to handle uncertainty and incomplete information, but its weakness lies in the complexity of building and maintaining accurate models.
Rule-based systems and frame-based systems are two common approaches to knowledge representation in expert systems.
- Rule-based systems represent knowledge as a set of “IF-THEN” rules. They are relatively easy to understand and implement, making them suitable for problems with clearly defined rules and a limited number of exceptions. However, they can become difficult to manage and maintain as the number of rules increases.
- Frame-based systems represent knowledge as a collection of frames, each containing slots that represent attributes and values. They are better suited for representing complex, hierarchical knowledge structures with many interrelationships. They are more flexible than rule-based systems but can be more complex to design and implement.
Feature | Rule-Based Systems | Frame-Based Systems |
---|---|---|
Knowledge Representation | Production rules (IF-THEN) | Frames with slots and values |
Structure | Linear, modular | Hierarchical, network-like |
Suitability | Problems with clear rules | Complex, hierarchical problems |
Maintainability | Can become difficult with many rules | More flexible, but complex to design |
Explainability | Relatively easy to explain | Can be more difficult to explain |
The choice of knowledge representation significantly impacts system performance and explainability. Semantic networks, production rules, and decision trees are common representations. Semantic networks represent knowledge as a graph of interconnected concepts. Production rules, as mentioned earlier, represent knowledge as IF-THEN statements. Decision trees represent knowledge as a tree-like structure, facilitating decision-making through a series of branching choices.
The choice of representation should be tailored to the specific problem and the available data.
The Role of Knowledge Engineers
Knowledge engineers play a pivotal role in building expert systems. Their responsibilities encompass various stages of the development process.
- Knowledge Acquisition: This involves eliciting knowledge from domain experts, which can be challenging due to incomplete, inconsistent, or tacit knowledge. Experts may not always be able to articulate their knowledge explicitly.
- Knowledge Base Validation and Verification: Rigorous testing and validation are crucial to ensure the accuracy and reliability of the knowledge base. This involves checking for inconsistencies, completeness, and correctness of the knowledge represented.
- Iterative Development: Knowledge engineering is an iterative process, requiring feedback loops to refine the knowledge base and improve system performance. Constant refinement based on testing and expert feedback is essential.
- Required Skills: Successful knowledge engineers need strong communication skills to interact with domain experts, a deep understanding of the problem domain, and programming skills to implement the system.
Knowledge Elicitation from Domain Experts
Effective knowledge elicitation is crucial for building accurate and reliable expert systems. Various techniques can be employed, each with its own advantages and disadvantages.
Technique | Advantages | Disadvantages |
---|---|---|
Interviews | Flexible, allows for in-depth exploration | Time-consuming, can be subjective |
Questionnaires | Efficient for collecting data from multiple experts | Limited flexibility, may miss important details |
Protocol Analysis | Provides detailed insights into expert reasoning | Requires significant effort to analyze protocols |
Observation | Captures tacit knowledge | Can be intrusive, may alter expert behavior |
Managing conflicts and inconsistencies in expert knowledge requires careful analysis and reconciliation. Techniques such as conflict resolution meetings and the use of multiple experts can help address these issues. The elicited knowledge must be represented in a structured format suitable for the chosen knowledge representation scheme (e.g., production rules, frames). Ethical considerations, including informed consent and data privacy, must be addressed throughout the knowledge elicitation process.
Case Study: MYCIN
A classic example of a successful expert system is MYCIN, developed in the 1970s to diagnose bacterial infections.
- Domain: Medical diagnosis of bacterial infections.
- Knowledge Representation: Production rules.
- Inference Engine: Backward chaining.
- Evaluation Metrics: Accuracy of diagnosis compared to human experts.
MYCIN demonstrated the potential of expert systems to assist medical professionals in diagnosis. Its rule-based approach, while effective for its time, also highlighted limitations. The knowledge base required constant updating as medical knowledge evolved. The system’s inability to handle uncertainty beyond its pre-programmed rules presented a significant challenge. The brittle nature of rule-based systems, prone to failure when encountering unexpected situations, is a key limitation. Moreover, the explainability, while improved through rule tracing, remained limited in addressing the complex nuances of medical diagnosis.
Limitations of Expert Systems
While expert systems have proven valuable in specific domains, they face limitations in the context of modern AI approaches. Their reliance on explicitly encoded knowledge makes them difficult to adapt to new situations or learn from new data. Modern AI techniques like deep learning excel at learning from vast amounts of data, surpassing expert systems in tasks requiring pattern recognition and generalization.
The knowledge acquisition bottleneck and the difficulty of maintaining and updating large knowledge bases remain significant challenges. The inherent brittleness of many expert systems, failing gracefully when presented with unexpected inputs, contrasts sharply with the robustness often found in deep learning models.
Knowledge-Based Theory and Artificial Intelligence
Knowledge-based theory forms the cornerstone of many artificial intelligence (AI) systems, providing a framework for representing and reasoning with information. The connection lies in the ability of knowledge-based systems to mimic human-like problem-solving by leveraging explicitly represented knowledge rather than relying solely on statistical methods. This allows for explainability and the handling of complex, symbolic information, crucial for many AI applications.
The Role of Knowledge Representation and Reasoning in AI
Knowledge representation and reasoning are fundamental to AI’s ability to perform intelligent tasks. Knowledge representation involves encoding information about the world in a structured format that a computer can understand and manipulate. Reasoning mechanisms then utilize this representation to draw inferences, solve problems, and make decisions. Without effective knowledge representation, AI systems would lack the necessary information to perform complex tasks; without effective reasoning, they couldn’t utilize that information effectively.
The choice of representation significantly impacts the system’s capabilities, efficiency, and scalability.
Influence of Different Knowledge Representation Schemes on AI System Design
Different knowledge representation schemes cater to different types of knowledge and problem-solving approaches. The choice of scheme significantly influences the design and capabilities of the resulting AI system.
- Semantic Networks: These represent knowledge as a graph of interconnected nodes and edges, where nodes represent concepts and edges represent relationships.
- Example 1: Representing family relationships (e.g., “John is the father of Mary,” represented by a node for “John,” a node for “Mary,” and an edge labeled “father of”).
- Example 2: Modeling the characteristics of different animal species (e.g., “mammal,” “has fur,” “lays eggs”).
- Example 3: Creating a knowledge base for a question-answering system, linking concepts and facts.
- Frames: These are data structures that represent stereotypical situations, objects, or events. They consist of slots that represent attributes and their values.
- Example 1: Representing a “bird” frame with slots like “has wings,” “can fly,” “lays eggs,” and specific values for each slot (e.g., “wings: two”).
- Example 2: Modeling a “car” frame with slots for “make,” “model,” “year,” “color,” and “engine type.”
- Example 3: Representing different types of medical diagnoses, including symptoms, causes, and treatments.
- Ontologies: These are formal representations of knowledge that define concepts, their properties, and relationships. They provide a shared understanding of a domain.
- Example 1: An ontology for medical terminology, defining concepts like “disease,” “symptom,” “treatment,” and their relationships.
- Example 2: An ontology for e-commerce, defining concepts like “product,” “customer,” “order,” and “payment.”
- Example 3: An ontology for geographical information, defining concepts like “country,” “city,” “river,” and their spatial relationships.
- Production Rules: These represent knowledge as a set of “if-then” rules, where the “if” part specifies a condition and the “then” part specifies an action to be taken if the condition is met.
- Example 1: A rule for a medical diagnosis system: “IF patient has fever AND cough THEN suspect influenza.”
- Example 2: A rule for a game-playing AI: “IF opponent has a winning move THEN block it.”
- Example 3: A rule for a financial trading system: “IF stock price drops below a certain threshold THEN sell the stock.”
Limitations of Current Knowledge-Based Approaches
Current knowledge-based approaches face limitations in handling uncertainty, incomplete information, and common-sense reasoning. Representing and reasoning with probabilistic information remains a challenge, as does dealing with situations where information is missing or ambiguous. Common-sense reasoning, which humans effortlessly perform, remains a significant hurdle for AI systems. These limitations often lead to brittle systems that fail in unexpected situations.
Comparison of AI Approaches Related to Knowledge Representation
The table provided offers a concise comparison of various AI approaches and their respective knowledge representation methods, highlighting their strengths and weaknesses. The choice of approach depends heavily on the specific application and the nature of the knowledge involved.
Examples of Knowledge-Based Theory in AI Applications
Knowledge-based theory finds application in numerous real-world scenarios.
- Medical Diagnosis: Expert systems utilizing rule-based systems and frames are used for preliminary diagnosis based on patient symptoms. Knowledge is acquired from medical textbooks, expert interviews, and clinical data. Performance varies depending on the complexity of the disease and the completeness of the knowledge base. Limitations include the inability to handle ambiguous cases and the risk of misdiagnosis due to incomplete or inaccurate knowledge.
- Financial Forecasting: Bayesian networks are employed to model financial markets and predict stock prices based on various economic indicators. Knowledge is acquired from historical data and expert opinions. Performance is influenced by the accuracy of the data and the ability of the model to capture the complexities of the market. Limitations include the difficulty of accurately modeling complex relationships and the inherent uncertainty in financial markets.
- Manufacturing Process Control: Rule-based systems are used to monitor and control manufacturing processes, ensuring quality and efficiency. Knowledge is acquired from engineering specifications, expert knowledge, and process data. Performance is measured by the reduction in defects and the improvement in production efficiency. Limitations include the difficulty of handling unexpected events and the need for continuous updates to the knowledge base as processes evolve.
Case Study: Medical Diagnosis System
One example is a medical diagnosis system using a Bayesian network to diagnose heart conditions. The system’s knowledge base comprises probabilistic relationships between symptoms (e.g., chest pain, shortness of breath) and diseases (e.g., heart attack, angina). Knowledge is acquired from medical literature and expert cardiologists. The system uses Bayesian inference to calculate the probability of each disease given the patient’s symptoms.
The development of this medical diagnosis system faced challenges in acquiring accurate and complete data, handling uncertainty in symptom presentation, and ensuring the system’s explainability to medical professionals. However, the successful implementation demonstrated the effectiveness of Bayesian networks in improving diagnostic accuracy, leading to earlier and more effective treatment for patients.
Ethical Implications of Knowledge-Based Systems in High-Stakes Decision-Making, What is knowledge based theory pdf
The use of knowledge-based systems in high-stakes decision-making scenarios raises significant ethical concerns. Bias in the data used to train these systems can lead to unfair or discriminatory outcomes. Lack of transparency in the reasoning process can make it difficult to understand why a system made a particular decision, hindering accountability. Ensuring fairness, transparency, and accountability is crucial to mitigate these risks and build trustworthy AI systems.
Limitations and Criticisms of Knowledge-Based Theory
Knowledge-based theory, while offering a powerful framework for understanding and utilizing knowledge, is not without its limitations and criticisms. These limitations stem from both inherent challenges in representing and managing knowledge and from broader philosophical debates surrounding the nature of knowledge itself. A critical examination of these aspects reveals areas where the theory falls short and highlights ongoing challenges in its practical application.
Knowledge Representation Limitations
One significant limitation lies in the difficulty of accurately and comprehensively representing knowledge. Knowledge is complex, often tacit, and context-dependent. Formal knowledge representation schemes, such as semantic networks or production rules, struggle to capture the nuances of human understanding, particularly the tacit knowledge that is often crucial for expertise. For instance, a skilled surgeon’s knowledge isn’t solely codified in medical textbooks; it involves intuition, experience-based judgment, and an ability to adapt to unexpected situations—aspects that are difficult to translate into a formal knowledge base.
The inherent limitations of symbolic representations in capturing the richness and ambiguity of human cognition remain a central challenge. Furthermore, the process of knowledge elicitation from experts can be time-consuming, expensive, and prone to error, leading to incomplete or inaccurate knowledge bases.
The Problem of Context and Ambiguity
Knowledge is not static; its meaning and applicability depend heavily on context. Knowledge-based systems often struggle to handle ambiguous or contradictory information, leading to incorrect inferences or decisions. For example, a system designed to diagnose medical conditions might misinterpret symptoms if it lacks sufficient contextual information about the patient’s medical history or lifestyle. The inability to effectively manage context and ambiguity limits the robustness and reliability of knowledge-based systems, particularly in complex and dynamic environments.
This highlights the inherent difficulty in creating knowledge bases that can accurately represent the subtleties of real-world situations.
Critique from a Social Constructivist Perspective
Social constructivist perspectives challenge the assumption that knowledge is an objective entity that can be extracted and codified. These perspectives emphasize the social and collaborative nature of knowledge creation and emphasize the role of shared understanding and interpretation. Knowledge-based theory, in its focus on individual expertise and formal representation, often overlooks the distributed and socially constructed nature of knowledge within organizations and communities.
This critique highlights the limitations of applying a purely individualistic approach to understanding and managing knowledge. The emphasis on explicit knowledge within many knowledge management systems can neglect the vital role of tacit knowledge, leading to an incomplete understanding of organizational learning.
Challenges in Knowledge Acquisition and Maintenance
The process of acquiring, updating, and maintaining knowledge bases is an ongoing challenge. Knowledge becomes obsolete quickly in rapidly evolving fields, requiring continuous updates and revisions. Furthermore, the cost and effort involved in maintaining large and complex knowledge bases can be substantial. This dynamic nature of knowledge necessitates robust mechanisms for knowledge acquisition, validation, and refinement, which are not always readily available or easily implemented.
The scalability of knowledge-based systems, particularly in dealing with vast amounts of data and diverse knowledge sources, remains a significant technological hurdle.
The Limitations of Expert Systems
Expert systems, a prime example of knowledge-based systems, have faced limitations in their ability to handle uncertainty, incomplete information, and complex reasoning tasks. While successful in specific domains, their performance often degrades when faced with situations outside their predefined knowledge base. This brittleness limits their generalizability and applicability across diverse contexts. Furthermore, the reliance on expert knowledge can introduce biases and limitations reflecting the expertise of the individual providing the knowledge.
The lack of explainability in some expert systems also raises concerns about transparency and trust.
Future Trends in Knowledge-Based Theory
Knowledge-based theory is a dynamic field constantly evolving with advancements in computing and data science. This section explores emerging trends, predicts future developments, analyzes advancements in knowledge representation and management, and concludes with a summary of key findings regarding the future trajectory of the field.
Emerging Trends and Applications
The convergence of artificial intelligence, big data, and advanced computational techniques is driving significant transformations in knowledge-based systems. This section examines the impact of several key trends and their applications across diverse domains.
Explainable AI (XAI) Impact on Trust and Transparency in Knowledge-Based Systems
Explainable AI (XAI) is crucial for enhancing trust and transparency in knowledge-based systems. Opaque AI models, while potentially powerful, lack the ability to explain their decision-making processes, hindering adoption, especially in high-stakes applications like healthcare and finance. XAI aims to bridge this gap by providing insights into the reasoning behind AI-driven conclusions. For instance, in medical diagnosis, an XAI system could not only identify a disease but also explain the specific factors (e.g., symptoms, test results) that led to the diagnosis, increasing physician confidence and patient trust.
Similarly, in loan applications, an XAI system could explain why a loan was approved or denied, promoting fairness and transparency. This improved explainability fosters greater acceptance and wider deployment of knowledge-based systems.
Big Data Analytics Impact on Knowledge Representation and Reasoning
The exponential growth of big data presents both challenges and opportunities for knowledge-based systems. Big data analytics allows for the extraction of complex patterns and relationships from massive datasets, enriching knowledge representation and reasoning. For example, in customer relationship management (CRM), big data analytics can identify previously unknown customer segments and preferences, leading to more targeted marketing campaigns. A case study of Netflix’s recommendation system illustrates this point.
Netflix leverages big data analytics to analyze user viewing history, preferences, and demographics to provide personalized movie and TV show recommendations, improving user engagement and satisfaction. This highlights how big data analytics fuels the evolution of knowledge representation and reasoning by enabling the creation of more comprehensive and nuanced knowledge models.
Rise of Hybrid Knowledge Representation Models
Traditional knowledge representation models often fall short when dealing with complex, real-world problems. Hybrid models, combining symbolic and sub-symbolic approaches, offer a more robust and flexible solution. Symbolic approaches (e.g., logic-based systems) excel at representing explicit knowledge, while sub-symbolic approaches (e.g., neural networks) are adept at learning implicit patterns from data. A concrete example is a hybrid system combining a rule-based expert system (symbolic) with a deep learning model (sub-symbolic) for medical diagnosis.
The rule-based system provides a framework for reasoning based on established medical knowledge, while the deep learning model identifies patterns in medical images, potentially revealing subtle indicators missed by human experts. This synergistic combination enhances the accuracy and reliability of the diagnostic system. Another example is using a knowledge graph (symbolic) enriched with embeddings learned from text data (sub-symbolic) to improve the accuracy of information retrieval.
Knowledge-Based Theory Application in Personalized Medicine and Healthcare
Knowledge-based systems are revolutionizing personalized medicine by enabling the development of tailored treatment plans based on individual patient characteristics. Advancements include the use of ontologies to represent medical knowledge, enabling efficient querying and reasoning about patient data. For example, systems can integrate patient genetic information, medical history, and lifestyle factors to predict disease risk and recommend personalized interventions.
This personalized approach enhances treatment effectiveness and reduces adverse effects. Furthermore, knowledge-based systems facilitate drug discovery by analyzing vast amounts of biological data to identify potential drug targets and predict drug efficacy.
Knowledge-Based Theory Application in Smart Manufacturing and Industrial Automation
In smart manufacturing, knowledge-based systems optimize production processes, improve quality control, and enhance predictive maintenance. Specific use cases include intelligent scheduling systems that optimize resource allocation based on real-time data, predictive maintenance systems that anticipate equipment failures, and quality control systems that identify defects in products. For instance, a knowledge-based system can monitor sensor data from a manufacturing plant, identify anomalies, and predict potential equipment failures, allowing for proactive maintenance and preventing costly downtime.
This leads to increased efficiency, reduced waste, and improved product quality.
Knowledge-Based Theory Application in Financial Modeling and Risk Management
Knowledge-based systems are increasingly used in financial modeling and risk management to improve decision-making and mitigate financial risks. Applications include fraud detection systems that identify suspicious transactions, algorithmic trading systems that execute trades based on complex market analyses, and credit scoring systems that assess the creditworthiness of borrowers. For example, a knowledge-based system can analyze a vast amount of financial data to identify patterns indicative of fraudulent activities, such as unusual transaction amounts or locations.
This allows financial institutions to proactively prevent fraud and minimize losses. Similarly, knowledge-based systems can be used to model complex financial instruments and assess their risks, enabling better investment decisions.
Short-Term Predictions (Within 5 Years)
Several factors point to specific trends in the near future of knowledge-based systems.
Prevalence of Specific Knowledge Representation Languages
We predict a continued rise in the use of OWL (Web Ontology Language) and RDF (Resource Description Framework) for knowledge representation, driven by the growing adoption of semantic web technologies and the need for interoperability between different knowledge bases. The standardization and wide adoption of these languages will facilitate data sharing and integration across various applications.
Adoption Rate of Specific Knowledge-Based System Development Tools
We anticipate increased adoption of low-code/no-code platforms for developing knowledge-based systems. These tools lower the barrier to entry for developers, allowing for faster prototyping and deployment of applications. The user-friendly nature of these platforms will drive wider adoption across various sectors.
Impact of Specific Technological Advancements
Quantum computing, while still in its nascent stages, holds the potential to revolutionize knowledge-based systems by enabling the solution of complex computational problems currently intractable for classical computers. This could lead to significant advancements in areas such as drug discovery and materials science, where the search space is incredibly vast.
Long-Term Predictions (Beyond 10 Years)
Looking further into the future, several transformative changes are expected in the field of knowledge-based systems.
Role of Knowledge-Based Systems in Addressing Global Challenges
Knowledge-based systems will play a pivotal role in addressing global challenges like climate change and poverty. They can be used to optimize resource allocation, predict environmental changes, and develop sustainable solutions. For example, knowledge-based systems can model climate change impacts on various ecosystems, helping to inform policy decisions and mitigation strategies. Similarly, they can be used to optimize the distribution of aid resources in disaster relief efforts.
Potential for Truly Autonomous Knowledge-Based Systems
The emergence of truly autonomous knowledge-based systems is likely, but gradual. This will involve significant advancements in machine learning, reasoning, and natural language processing. However, ensuring safety, reliability, and ethical considerations will be paramount. The implications are far-reaching, potentially leading to automation of complex tasks across various industries.
Ethical Considerations Surrounding Widespread Use of Advanced Knowledge-Based Systems
The widespread use of advanced knowledge-based systems raises several ethical concerns, including bias in algorithms, data privacy, and job displacement. Addressing these concerns through robust regulatory frameworks and ethical guidelines will be crucial to ensure responsible development and deployment of these technologies. Transparency and accountability mechanisms are vital to build public trust and prevent misuse.
Advancements in Knowledge Representation and Management
Continuous advancements in knowledge representation and management are essential for the continued growth of knowledge-based systems.
Developments in Ontological Engineering Methodologies
Improved ontological engineering methodologies focus on automating ontology creation and maintenance, addressing the challenges of scalability and consistency. Techniques such as ontology learning from data and ontology merging are becoming increasingly sophisticated. For example, machine learning algorithms can be used to automatically extract ontologies from large text corpora, significantly reducing the manual effort required.
Knowledge Acquisition Techniques
Machine learning is playing a crucial role in knowledge acquisition, enabling the automated extraction of knowledge from various sources, including text, images, and sensor data. For instance, deep learning models can be used to extract knowledge from unstructured text data, such as medical reports or scientific publications. This automated approach significantly accelerates the knowledge acquisition process and enables the creation of larger and more comprehensive knowledge bases.
Knowledge Reasoning Mechanisms
Advancements in reasoning under uncertainty are crucial for dealing with real-world scenarios where information is incomplete or unreliable. Probabilistic reasoning methods, such as Bayesian networks and Markov logic networks, are increasingly used to handle uncertainty and provide robust reasoning capabilities. For example, Bayesian networks can be used to model the uncertainty associated with medical diagnoses, allowing for more informed decision-making.
Comparative Analysis of Knowledge Representation Formalisms
| Formalism | Expressiveness | Reasoning Capabilities | Scalability ||—————–|————————–|————————|—————–|| Semantic Networks | Represents concepts and relationships using nodes and edges. Limited expressiveness for complex relationships. | Simple reasoning based on graph traversal. Limited for complex inferences. | Relatively scalable, but can become computationally expensive for very large networks.
|| Frames | Represents knowledge as structured entities with attributes and values. Allows for inheritance and default values. More expressive than semantic networks. | Inference based on slot filling and inheritance. Can handle more complex reasoning than semantic networks.
| Moderately scalable, but performance can degrade with a large number of frames and complex inheritance hierarchies. || Description Logics| Formal language for representing knowledge with well-defined semantics. Highly expressive, capable of representing complex concepts and relationships. | Supports complex reasoning tasks such as classification, subsumption, and instance checking. Efficient reasoning algorithms exist.
| Reasoning complexity can be high for very expressive ontologies, but optimized reasoners exist to handle large-scale ontologies. |
Challenges and Opportunities Associated with Managing Large-Scale Knowledge Graphs and Ontologies
- Challenge: Scalability – Managing and querying extremely large knowledge graphs and ontologies efficiently.
- Challenge: Consistency – Maintaining consistency and avoiding contradictions in large knowledge bases.
- Challenge: Quality – Ensuring the quality and accuracy of the knowledge represented.
- Challenge: Interoperability – Enabling seamless integration and exchange of knowledge between different knowledge bases.
- Opportunity: Automated ontology learning – Developing methods for automatically learning ontologies from data.
- Opportunity: Ontology merging – Developing techniques for merging different ontologies into a unified knowledge base.
- Opportunity: Knowledge graph visualization – Developing effective methods for visualizing and exploring large knowledge graphs.
Case Studies of Knowledge-Based Systems
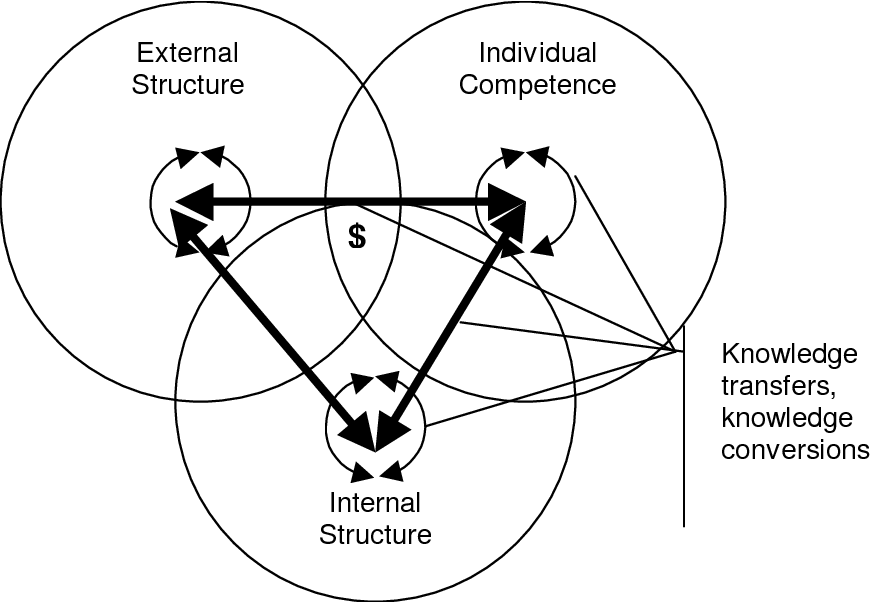
This section presents a detailed case study of a successful knowledge-based system in the medical diagnosis domain, illustrating the design, implementation, evaluation, and impact of such systems. Analyzing this case study provides valuable insights into the practical applications and challenges of knowledge-based theory.
MYCIN: A Medical Diagnosis System
MYCIN, developed in the 1970s at Stanford University, is a pioneering example of a knowledge-based system for diagnosing bacterial infections. Its initial deployment was in the mid-1970s, though it never received widespread clinical adoption. Despite this, its impact on the field of knowledge-based systems and artificial intelligence in medicine remains significant.
System Design
Knowledge Representation
MYCIN employed a rule-based expert system architecture. Its knowledge base consisted of a large number of production rules, each representing a piece of medical expertise in the form of an “IF-THEN” statement. These rules were elicited from expert physicians through interviews and careful analysis of medical literature. Uncertainty was handled using certainty factors, a numerical representation of the confidence associated with each rule’s conclusion.
Feature | Description |
---|---|
Representation Type | Rule-based |
Knowledge Source | Expert interviews, medical literature |
Reasoning Method | Backward chaining |
Uncertainty Handling | Certainty factors |
Inference Engine
MYCIN used a backward chaining inference engine. Starting with a hypothesis (e.g., a specific bacterial infection), the system worked backward, searching for rules that could support or refute the hypothesis. The certainty factors associated with each rule were combined to arrive at an overall certainty for the hypothesis. This process continued until a diagnosis was reached or no further rules were applicable.
User Interface
The user interface was primarily text-based, involving a series of questions and answers between the system and the physician. The system would ask questions about the patient’s symptoms, medical history, and laboratory results. Based on the responses, MYCIN would infer possible diagnoses and suggest appropriate treatments, along with associated certainty factors.
System Implementation
Programming Language
MYCIN was implemented primarily in Lisp, a language well-suited for symbolic reasoning and knowledge representation.
Development Environment
The development environment consisted of standard Lisp development tools available at the time.
Data Sources
The knowledge base was populated through extensive interviews with infectious disease specialists and a review of relevant medical literature.
Testing Methodology
MYCIN’s performance was evaluated using a combination of techniques, including testing against known cases, comparing its diagnoses to those of expert physicians, and evaluating its ability to handle incomplete or uncertain information.
System Evaluation
Evaluation Metrics
The evaluation of MYCIN focused on the accuracy of its diagnoses, the consistency of its reasoning, and the usability of its interface. Quantitative metrics, such as the percentage of correct diagnoses, were used to assess accuracy. Qualitative feedback from physicians was also gathered to assess usability and acceptance.
Results
While MYCIN didn’t achieve perfect accuracy, its performance was comparable to, and in some cases superior to, that of individual expert physicians in diagnosing bacterial infections. The system’s ability to handle uncertainty and incomplete information was also considered a significant achievement.
Comparative Analysis
MYCIN’s performance was compared to the diagnostic abilities of expert physicians. While a direct head-to-head comparison was not always possible, the results generally indicated comparable or superior performance in specific scenarios.
Impact of the System
Benefits
MYCIN demonstrated the feasibility of building knowledge-based systems for complex medical tasks. It highlighted the potential for such systems to assist physicians in making more accurate and consistent diagnoses, potentially improving patient care.
Limitations
MYCIN’s reliance on certainty factors for uncertainty management was a significant limitation. The system’s knowledge base was also limited in scope and required continuous updates to reflect the evolving state of medical knowledge. Furthermore, the lack of a graphical user interface and the complexity of the reasoning process limited its usability.
Future Improvements
Future improvements could include incorporating more sophisticated uncertainty management techniques, expanding the knowledge base to cover a broader range of medical conditions, and developing a more user-friendly interface. Integration with electronic health records would also enhance its practicality.
The MYCIN case study underscores the importance of robust knowledge representation, effective inference mechanisms, and rigorous testing in the development of knowledge-based systems. Careful consideration of uncertainty management and user interface design are crucial for ensuring system usability and acceptance. Furthermore, continuous knowledge base maintenance and updates are essential for maintaining system relevance and accuracy.
Sources
[This section would include a bibliography with at least three sources using a consistent citation style (e.g., APA). Due to the limitations of this text-based response, I cannot provide specific bibliographic entries here. However, relevant sources would include publications on MYCIN’s development and evaluation, as well as general works on expert systems and knowledge-based systems in medicine.]
Ethical Considerations in Knowledge-Based Systems
Knowledge-based systems (KBS), while offering significant advantages in efficiency and decision-making, present complex ethical challenges. The potential for bias, lack of transparency, and unforeseen consequences necessitates careful consideration of ethical implications throughout the entire lifecycle of a KBS, from design and development to deployment and monitoring. Failing to address these concerns can lead to discriminatory outcomes and erode public trust.
Ethical Implications of Using Knowledge-Based Systems
The use of knowledge-based systems carries several ethical implications, primarily stemming from the potential for biased data to produce discriminatory outcomes. These systems, trained on historical data, can inadvertently perpetuate and even amplify existing societal biases related to gender, race, and socioeconomic status. This is particularly problematic in sensitive areas such as loan applications, hiring processes, and criminal justice risk assessments.For instance, a loan application system trained on data reflecting historical lending practices that disproportionately favored certain demographics might unfairly deny loans to individuals from underrepresented groups, even if those individuals are equally creditworthy.
Similarly, a hiring system trained on past hiring data could perpetuate gender bias by favoring male candidates over equally qualified female candidates. In criminal justice, risk assessment tools trained on data reflecting existing racial disparities in arrests and convictions could lead to biased predictions, potentially exacerbating existing inequalities.The opacity of some KBS, especially those employing complex machine learning algorithms, further complicates ethical considerations.
The difficulty in understanding how these systems arrive at their decisions makes it challenging to identify and address biases, hindering accountability and transparency. This “black box” nature undermines trust and makes it difficult to rectify unfair or discriminatory outcomes.
Potential Biases in Knowledge Representation and Application
Implicit biases within training data are a significant source of discriminatory outputs in knowledge-based systems. These biases can manifest in various ways, depending on the type of bias present in the data and the chosen knowledge representation technique.
Bias Type | Description | Example in Knowledge-Based System | Potential Impact |
---|---|---|---|
Confirmation Bias | Favoring information confirming existing beliefs. | A system trained on data reflecting only one viewpoint regarding a specific medical treatment, ignoring contradictory evidence. | Reinforces existing prejudices; limits perspectives, leading to potentially suboptimal or even harmful recommendations. |
Sampling Bias | Data not representative of the target population. | A facial recognition system trained primarily on images of individuals with light skin tones, resulting in poor performance for individuals with darker skin tones. | Inaccurate or biased results for underrepresented groups. |
Measurement Bias | Inconsistent or flawed data collection methods. | A system for assessing job applicant suitability relying on self-reported data prone to exaggeration or misrepresentation. | Erroneous conclusions and recommendations based on unreliable data. |
Attribution Bias | Attributing outcomes to individual characteristics rather than systemic factors. | A loan application system that unfairly penalizes applicants from low-income neighborhoods without considering broader economic factors. | Perpetuates societal inequalities by overlooking systemic issues. |
The choice of knowledge representation technique also influences the potential for bias. Rule-based systems, with their explicit rules, may be easier to audit for bias, but they can still reflect the biases of their creators. Neural networks, while powerful, are often opaque, making bias detection and mitigation more challenging.Human oversight is crucial in mitigating bias. Careful selection and preprocessing of training data, regular audits for bias, and ongoing monitoring of system outputs are essential.
Human experts should be involved in both the development and deployment phases to identify and correct potential biases.
Strategies for Ensuring Ethical and Responsible Development and Deployment of Knowledge-Based Systems
Developing and deploying ethical knowledge-based systems requires a multi-faceted approach encompassing data preprocessing, system design, and ongoing monitoring.Methods for detecting and mitigating bias in training data include data augmentation (adding synthetic data to balance representation), adversarial training (training the system to resist adversarial attacks designed to expose biases), and careful data cleaning and preprocessing to identify and remove biased or irrelevant information.Designing explainable and interpretable knowledge-based systems is crucial for enhancing transparency and accountability.
Techniques like LIME (Local Interpretable Model-agnostic Explanations) and SHAP (SHapley Additive exPlanations) can help explain individual predictions, making it easier to identify and address biases.A robust ethical review and approval framework is necessary before deploying any KBS. This framework should include guidelines for assessing potential risks and harms, involving diverse stakeholders in the review process, and ensuring that the system aligns with relevant ethical guidelines and regulations.
The framework should mandate impact assessments that specifically address potential biases and discriminatory outcomes.Ongoing monitoring and evaluation are vital for identifying and addressing emerging ethical concerns. Key performance indicators (KPIs) should be established to track the system’s performance across different demographic groups, ensuring fairness and equity. These KPIs might include:* Accuracy rates across different demographic groups.
- Error rates and types of errors made by the system.
- Frequency and nature of biased or discriminatory outcomes.
- User feedback regarding the system’s fairness and transparency.
- The system’s adherence to predefined ethical guidelines.
Regular audits and updates based on monitoring data are crucial to ensure the system remains ethical and effective over time.
Illustrative Example: A Knowledge Base for Medical Diagnosis
This section presents a simplified knowledge base for medical diagnosis, illustrating the core principles of knowledge representation and reasoning within a knowledge-based system. The example focuses on a limited set of symptoms and diseases to maintain clarity and simplicity, highlighting the fundamental structure and functionality. A more comprehensive system would necessitate a significantly larger and more complex knowledge base.
The knowledge base utilizes a rule-based approach, where rules are defined in the form of “IF-THEN” statements. Each rule connects a set of symptoms (the IF part) to a possible diagnosis (the THEN part). The system uses a forward-chaining inference engine, starting from observed symptoms and applying rules to deduce possible diagnoses. Certainty factors can be incorporated to reflect the strength of association between symptoms and diagnoses, allowing for probabilistic reasoning.
Knowledge Base Structure
The knowledge base is structured as a set of rules represented in a tabular format for ease of understanding. A more sophisticated system might use a more complex representation such as a semantic network or an ontology. This tabular representation allows for straightforward rule evaluation and diagnosis generation.
Rule ID | Symptoms | Diagnosis | Certainty Factor |
---|---|---|---|
R1 | High fever, cough, muscle aches | Influenza | 0.8 |
R2 | Sore throat, swollen glands, fatigue | Mononucleosis | 0.7 |
R3 | High fever, headache, stiff neck | Meningitis | 0.9 |
R4 | Cough, shortness of breath, chest pain | Pneumonia | 0.6 |
R5 | Runny nose, sneezing, congestion | Common Cold | 0.5 |
Diagnosis Process
Let’s consider a patient presenting with the following symptoms: high fever, cough, and muscle aches. The system would proceed as follows:
- Symptom Input: The system receives the input: high fever, cough, muscle aches.
- Rule Matching: The system searches the knowledge base for rules that match these symptoms. Rule R1 (High fever, cough, muscle aches → Influenza) is identified as a match.
- Diagnosis Generation: The system infers a diagnosis of Influenza with a certainty factor of 0.8 based on Rule R1.
- Output: The system outputs the diagnosis: “Based on your symptoms, you may have Influenza (Certainty: 0.8). Further medical evaluation is recommended.”
Example of Rule Application with Uncertainty
Consider a patient presenting with a cough and shortness of breath. Rules R4 (Cough, shortness of breath, chest pain → Pneumonia) and R5 (Runny nose, sneezing, congestion → Common Cold) are partially matched. The system would assess the certainty factors. If chest pain is absent, the certainty factor for Pneumonia would be reduced (e.g., to 0.4). The system would then compare the certainty factors for Pneumonia and Common Cold to suggest the most probable diagnosis, while emphasizing the need for further evaluation.
This illustrates how the system handles uncertainty inherent in medical diagnosis.
Q&A: What Is Knowledge Based Theory Pdf
What are the limitations of knowledge-based systems?
Knowledge-based systems can be brittle, struggling with unexpected inputs or situations outside their explicitly programmed knowledge. They can also be difficult and expensive to build and maintain, requiring significant expertise in knowledge engineering. Furthermore, they may struggle with uncertainty and common-sense reasoning.
How does knowledge-based theory relate to organizational learning?
Knowledge-based theory provides a framework for understanding how organizations acquire, share, and utilize knowledge. It informs strategies for knowledge management, emphasizing the importance of both explicit and tacit knowledge in organizational learning and performance improvement.
What is the role of knowledge engineers?
Knowledge engineers are specialists who elicit, represent, and integrate expert knowledge into knowledge-based systems. They bridge the gap between domain experts and computer systems, ensuring the system accurately reflects the expertise needed to solve problems.
What are some examples of real-world applications of knowledge-based systems beyond those mentioned in the Artikel?
Examples include fraud detection systems in finance, customer service chatbots, and intelligent tutoring systems in education.