Waters knowledge base represents a significant advancement in water resource management. It transcends simple hydrological databases by integrating diverse data types – from hydrological modeling outputs and water quality measurements to socio-economic factors and policy documents – into a unified, searchable repository. This holistic approach aims to empower stakeholders with the information needed for informed decision-making, fostering better water resource management and scientific understanding.
The success of such a system, however, hinges on careful consideration of data quality, accessibility, and ethical implications.
The effective implementation of a waters knowledge base requires a robust data model capable of handling the complexity and volume of water-related data. This includes not only the technical aspects of database selection and data integration, but also the critical need for standardized data formats, rigorous quality control procedures, and clear data ownership policies. Furthermore, the user interface must be intuitive and accessible to a diverse range of stakeholders, from scientists and policymakers to the general public.
Defining “Waters Knowledge Base”
A Waters Knowledge Base is a centralized repository of information related to all aspects of water. It’s more than just a database; it’s a curated collection of data, models, and expert knowledge designed to support informed decision-making and action related to water resources. This encompasses scientific understanding, managerial strategies, and public awareness.
Comprehensive Definition of a Waters Knowledge Base
A Waters Knowledge Base integrates diverse data sources and knowledge types to provide a holistic understanding of water resources. It differs from hydrological databases by incorporating not only raw data but also interpreted information, models, and expert insights. Unlike water resource management information systems (WRMIS), which focus primarily on operational management, a Waters Knowledge Base serves a broader audience with diverse needs, including researchers, policymakers, and the public.
The boundaries of a Waters Knowledge Base are defined by its scope, which may range from a local watershed to a global scale, and by the types of water-related information it contains.
Knowledge Types Included in a Waters Knowledge Base
The following table categorizes the types of knowledge included in a Waters Knowledge Base:
Knowledge Category | Description | Examples | Data Format | Source |
---|---|---|---|---|
Hydrological Data | Measurements of water quantity and quality. | Streamflow, precipitation, water temperature, dissolved oxygen, nutrient levels. | Time series, spatial grids, point data | Monitoring stations, weather stations, remote sensing |
Water Resource Management Plans | Policies, strategies, and actions for managing water resources. | Water allocation plans, drought management plans, flood control plans. | Documents, maps, databases | Government agencies, NGOs, research institutions |
Water Quality Models | Mathematical models used to simulate and predict water quality. | WQ models predicting the impact of pollution on water bodies. | Software code, model parameters, simulation results | Research institutions, environmental consulting firms |
Geographic Information System (GIS) Data | Spatial data representing water-related features. | River networks, lakes, reservoirs, watersheds, land use. | Shapefiles, GeoTIFFs, geodatabases | Surveys, remote sensing, GIS databases |
Stakeholder Knowledge and Perspectives | Information gathered from various stakeholders regarding their experiences and knowledge related to water. | Interviews, focus groups, surveys, community reports regarding water use and management. | Text documents, audio/video recordings, survey data | Community members, local governments, NGOs |
User and Stakeholder Analysis
A Waters Knowledge Base benefits various user groups. Effective access management is crucial, balancing open access with the need to protect sensitive data.
- Scientists and Researchers: Need access to raw data, models, and publications for research and analysis. Access would be managed through user accounts and permissions based on affiliation and project needs.
- Water Resource Managers: Require access to real-time data, forecasts, and management plans for operational decision-making. Access is controlled through roles and responsibilities within their organizations.
- Policymakers: Need access to synthesized information, reports, and analyses to inform policy decisions. Access would be public for general information but restricted for sensitive data related to policy formulation.
- Public: Need access to information about water quality, availability, and management practices to raise awareness and promote responsible water use. Public access would be granted to a subset of the data, ensuring ease of understanding and interpretation.
- Environmental NGOs: Require access to data and information to monitor water quality, advocate for improved water management, and conduct their own research and analysis. Access is granted based on the NGO’s mission and established collaborations.
Data Sources for a Waters Knowledge Base
A comprehensive waters knowledge base requires diverse data sources to paint a complete picture of our precious water resources. This section details the identification, assessment, and integration of key data sources, emphasizing spatiotemporal resolution and addressing ethical considerations. Imagine it like building a magnificent Palembang rumah limas – you need strong, varied materials to create a sturdy and beautiful structure!
Data Source Identification and Description
Identifying reliable and relevant data sources is crucial for building a robust waters knowledge base. Below are five diverse data sources, each offering unique perspectives on water resources.
- Source 1: Global Surface Water Explorer (GSWE): This dataset provides global surface water extent and change information derived from satellite imagery. It boasts high spatiotemporal resolution, covering the entire globe with frequent updates. Data is available as raster data (GeoTIFF) and offers valuable insights into water body dynamics, but its accuracy can be affected by cloud cover and image resolution limitations. The temporal resolution is typically annual or seasonal.
- Source 2: Water Quality Monitoring Network Data (e.g., USGS Water Quality Data): Many national and international agencies collect water quality data from various monitoring stations. These datasets usually consist of tabular data (CSV) containing various parameters (e.g., temperature, pH, dissolved oxygen). Geographic coverage varies depending on the network’s density, and temporal resolution can range from daily to yearly. Data quality depends on the consistency of monitoring protocols and instrument calibration.
- Source 3: Global Biodiversity Information Facility (GBIF): GBIF aggregates biodiversity occurrence data from various sources, including museum collections, citizen science projects, and research studies. This includes aquatic species. Data is primarily presented as point data (CSV or Shapefile), with varying spatial and temporal resolution depending on the contributing datasets. Data quality varies, depending on the methods used for species identification and data collection.
- Source 4: Hydrological Modeling Outputs (e.g., outputs from the SWAT model): Hydrological models simulate water flow and storage within catchments. These models produce raster or vector data (GeoTIFF, Shapefile) representing various hydrological variables (e.g., runoff, evapotranspiration). Spatial and temporal resolution depend on the model parameters and input data, and the accuracy is influenced by the model’s assumptions and calibration process.
- Source 5: Socio-economic Census Data: National censuses and surveys collect socio-economic data, including information on water access, sanitation, and household income. Data is typically tabular (CSV) and is often aggregated at administrative units. Spatial resolution is usually coarse (e.g., at the district or province level), while temporal resolution depends on the frequency of the census. Data quality relies on the accuracy of the survey methodology and response rates.
Data Source Comparison and Reliability Assessment
Each data source possesses unique strengths and weaknesses regarding reliability and accuracy. A nuanced understanding of these factors is crucial for effective data integration and interpretation.
- GSWE: High reliability due to consistent satellite imagery processing, but susceptible to cloud cover and resolution limitations.
- Water Quality Monitoring Network Data: Reliability varies depending on data collection protocols and instrument calibration; potential biases due to uneven spatial distribution of monitoring stations.
- GBIF: Reliability varies greatly among datasets; potential biases related to observer bias and taxonomic expertise.
- Hydrological Modeling Outputs: Reliability depends on model accuracy and calibration; uncertainties associated with model parameters and input data.
- Socio-economic Census Data: High reliability for aggregated data, but subject to sampling errors and potential biases in survey responses.
To mitigate uncertainties and errors, data validation, quality control procedures, and data fusion techniques (combining data from multiple sources) are essential. For example, comparing water quality data with hydrological model outputs can help identify inconsistencies and improve model accuracy.
Data Source Organization and Presentation
Source Name | Source Type | Data Format | Accessibility | Spatial Resolution | Temporal Resolution | Reliability Assessment | Description |
---|---|---|---|---|---|---|---|
Global Surface Water Explorer (GSWE) | Remote Sensing | GeoTIFF | Public | Variable | Annual/Seasonal | High | Surface water extent |
USGS Water Quality Data | Monitoring Network | CSV | Public | Point | Variable | Medium | Water quality parameters |
Global Biodiversity Information Facility (GBIF) | Biodiversity Data | CSV, Shapefile | Public | Variable | Variable | Medium | Aquatic species occurrences |
SWAT Model Outputs | Hydrological Modeling | GeoTIFF, Shapefile | Variable | Variable | Variable | Medium | Hydrological variables |
Socio-economic Census Data | Socio-economic Data | CSV | Public | Coarse | Variable | High | Water access, sanitation |
Hyperlinks to Data Sources
- Global Surface Water Explorer (GSWE)
- USGS Water Data
- Global Biodiversity Information Facility (GBIF)
Data Integration and Harmonization Considerations
Integrating data from these diverse sources presents challenges due to differences in data formats, spatial resolutions, coordinate systems, and temporal coverage. Data transformation and standardization are necessary to ensure consistency. Geospatial data needs to be projected to a common coordinate system. Data fusion techniques, such as data assimilation, can combine data from different sources to improve accuracy and fill data gaps.
Ethical Considerations
Accessing, using, and sharing these data sources requires careful consideration of ethical implications. Data privacy concerns arise with socio-economic data, requiring anonymization or aggregation to protect individual identities. Intellectual property rights related to hydrological models and biodiversity datasets need to be respected. Data sovereignty principles should be considered, ensuring data is used and shared in a way that respects national and local laws and regulations.
Structure and Organization of a Waters Knowledge Base
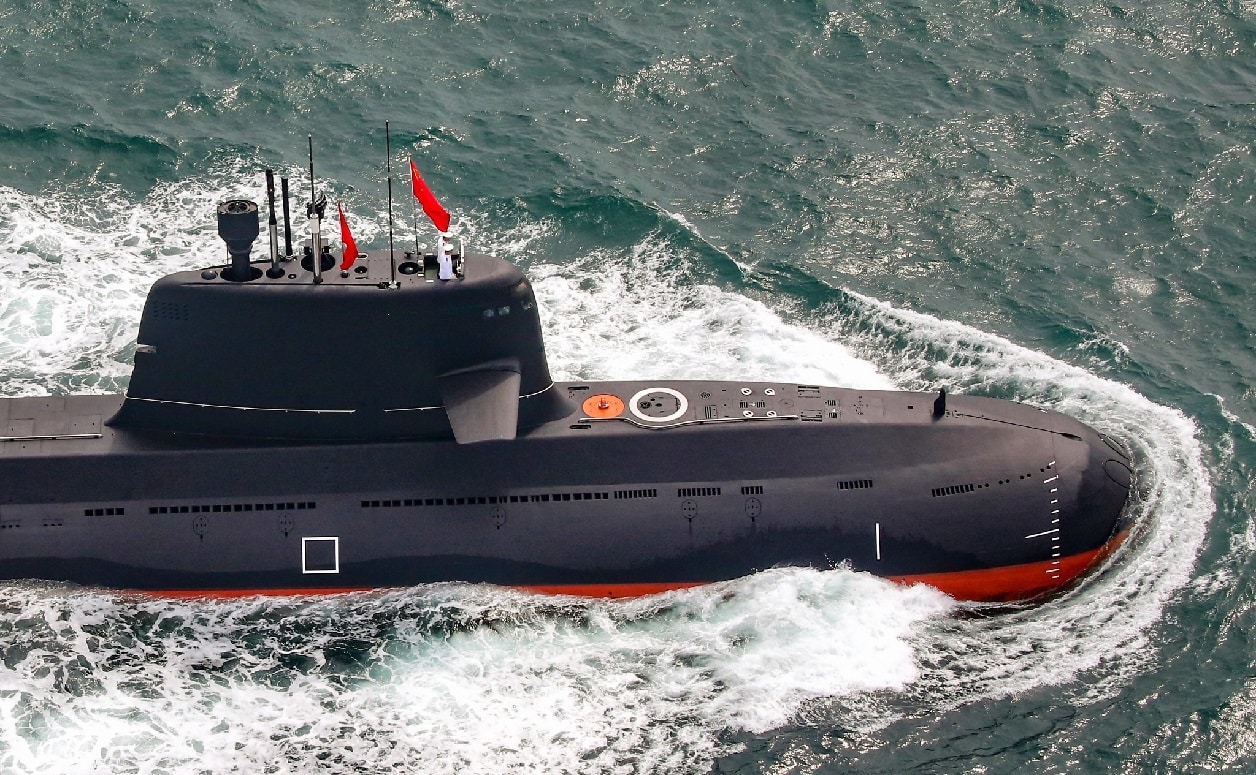
A robust waters knowledge base, especially one focused on freshwater ecosystems, requires careful consideration of its structure and organization to ensure efficient data storage, retrieval, and analysis. The design should prioritize accessibility for researchers and facilitate insightful discoveries about the complex dynamics of these vital environments. This section details a proposed structure, considering various organizational approaches and their implications for a freshwater ecosystem knowledge base.
Conceptual Model Design
A conceptual model for organizing information within a freshwater ecosystem knowledge base needs to accommodate diverse data types. We propose a relational model, capturing the interconnectedness of hydrological, water quality, biological, and geographical data. This model facilitates complex queries and analysis, reflecting the intricate relationships within freshwater ecosystems. The following table Artikels the entities and their key attributes:
Entity | Attribute | Data Type |
---|---|---|
WaterBody | Name | String |
WaterBody | Latitude | Float |
WaterBody | Longitude | Float |
WaterBody | Elevation | Float |
WaterBody | WaterBodyType | String (e.g., River, Lake, Wetland) |
WaterQualityData | WaterBodyID | Integer |
WaterQualityData | Date | Date |
WaterQualityData | Temperature | Float |
WaterQualityData | pH | Float |
WaterQualityData | DissolvedOxygen | Float |
WaterQualityData | Nitrate | Float |
SpeciesData | WaterBodyID | Integer |
SpeciesData | SpeciesID | Integer |
SpeciesData | Abundance | Integer |
SpeciesData | Date | Date |
HydrologicalData | WaterBodyID | Integer |
HydrologicalData | Date | Date |
HydrologicalData | FlowRate | Float |
HydrologicalData | WaterLevel | Float |
Species | SpeciesID | Integer |
Species | ScientificName | String |
Species | CommonName | String |
Organizational Structures
Three organizational structures are compared: hierarchical, relational, and network. A relational database structure is best suited for a waters knowledge base due to its ability to manage complex relationships between different data types efficiently and scalably. A hierarchical structure, while simpler to visualize, would struggle with the many-to-many relationships inherent in ecological data. A network structure, while flexible, could become unwieldy and difficult to query with large datasets.
Visual Representation
The chosen relational structure can be visualized as a series of interconnected tables. For instance, the `WaterBody` table is linked to `WaterQualityData`, `SpeciesData`, and `HydrologicalData` tables via foreign keys (`WaterBodyID`). The `SpeciesData` table is linked to the `Species` table via `SpeciesID`.* WaterBody Table: Contains information on individual water bodies (e.g., River Musi, Lake Ranau). Each entry has a unique `WaterBodyID`.
WaterQualityData Table
Contains water quality measurements for each `WaterBodyID`.
Each entry includes `Date`, `Temperature`, `pH`, `DissolvedOxygen`, and `Nitrate` levels.
SpeciesData Table
Contains data on species presence, abundance, and biomass within each `WaterBodyID`.
Each entry links to a `SpeciesID` and includes `Abundance` and `Date` of observation.
Species Table
Contains information on each species, with a unique `SpeciesID`, `ScientificName`, and `CommonName`.
HydrologicalData Table
Contains hydrological data such as `FlowRate` and `WaterLevel` for each `WaterBodyID` and `Date`.
Data Relationships
The relationships are primarily one-to-many. One `WaterBody` can have many `WaterQualityData` entries, many `SpeciesData` entries, and many `HydrologicalData` entries. The `SpeciesData` table has a one-to-many relationship with the `Species` table; one species can be present in many water bodies.
Querying the Knowledge Base (Example), Waters knowledge base
1. Query
Retrieve the average temperature and dissolved oxygen levels for River Musi in
2023. Expected Output
A table with average temperature and dissolved oxygen values for River Musi for each month in
2023. 2. Query
List all species observed in Lake Ranau with abundance greater than
100. Expected Output
A list of species names and their abundance in Lake Ranau exceeding
100. 3. Query
Find water bodies with nitrate levels consistently above 5 ppm in the last year. Expected Output: A list of water body names exceeding the nitrate threshold.
Scalability and Maintainability
The relational database structure is highly scalable. Adding new data points or water bodies simply involves adding new rows to the respective tables. Regular database maintenance, including data backups, indexing, and query optimization, will ensure efficient performance even with large datasets. Data integrity can be maintained through constraints and validation rules within the database schema. Error handling mechanisms, such as logging and exception handling, can be incorporated into the data processing pipelines to improve the robustness of the system.
Data Management and Maintenance
Maintaining the accuracy, completeness, and consistency of the Waters Knowledge Base is paramount to its utility and reliability. Effective data management ensures the information remains valuable and trustworthy for all users, from researchers to policymakers. This section details the procedures and strategies implemented to achieve and maintain high data quality within the knowledge base.
Data Accuracy, Completeness, and Consistency
Maintaining data accuracy, completeness, and consistency is crucial for the reliability of the Waters Knowledge Base. These aspects are addressed through a multi-faceted approach involving validation, imputation techniques, and data normalization.
Methods for Validating Data Accuracy
Three distinct methods are employed to validate the accuracy of newly ingested water data: cross-referencing, statistical analysis, and expert review. These methods leverage various data sources, including government agencies (e.g., the EPA), scientific publications (e.g., peer-reviewed journals), and sensor networks (e.g., hydrological monitoring stations).
Method | Data Sources | Validation Technique | Strengths | Weaknesses | Applicability |
---|---|---|---|---|---|
Cross-referencing | Government agencies, scientific publications | Comparing data against established values from multiple sources. | High reliability when multiple sources agree; relatively simple to implement. | Requires multiple reliable sources; may not detect subtle errors. | Water temperature, pH, flow rate |
Statistical Analysis | Sensor networks, historical data | Applying statistical tests (e.g., outlier detection, regression analysis) to identify inconsistencies. | Can detect subtle errors and outliers; objective and quantitative. | Requires sufficient data for statistical significance; may require specialized expertise. | All data types |
Expert Review | All sources | Subject matter experts review data for plausibility and consistency. | Can identify errors that statistical methods may miss; considers contextual information. | Subjective; may be time-consuming and expensive. | All data types |
Procedure for Identifying and Addressing Incomplete Data
A systematic procedure is implemented to handle incomplete data entries. This involves detecting missing values, determining the cause of incompleteness (e.g., sensor malfunction, data transmission errors), and applying appropriate strategies for imputation or removal. The flowchart would begin with “Data Ingestion.” The next step would be “Missing Value Detection.” This leads to a decision point: “Is the percentage of missing data acceptable?”. If yes, the data proceeds to “Data Integration.” If no, the process branches to “Determine Cause of Incompleteness.” This leads to another decision point: “Is imputation feasible?”. If yes, the process moves to “Impute Missing Values” and then to “Data Integration.” If no, the process goes to “Remove Incomplete Data” and then to “Data Integration.” Finally, “Data Integration” leads to the end point. Imputation methods include mean/median imputation, regression imputation, or using data from similar locations or time periods. Removal of incomplete data is only used as a last resort when imputation is not appropriate.
Maintaining Data Consistency Across Sources
Data consistency is maintained through data normalization, standardization of units, and conflict resolution procedures. Data normalization involves transforming data into a consistent format, addressing issues such as different date formats or data structures. Standardization ensures all data uses consistent units (e.g., metric system). Conflicting information is resolved through a priority system, giving preference to data from more reliable sources, such as government agencies or peer-reviewed publications.
For instance, if two sources provide conflicting water temperature readings for the same location and time, the reading from a validated government sensor network would be prioritized.
Data Update and Maintenance Procedures
Schedule for Regular Updates
The frequency of updates varies depending on the data type and its volatility. Real-time data, such as sensor readings from water quality monitoring stations, will be updated daily. Data with lower volatility, such as long-term climate trends, may be updated monthly or even annually. This schedule is designed to balance the need for up-to-date information with the resources required for data acquisition and processing.
Incorporating New Data Sources
The process for incorporating new data sources involves several steps: data ingestion (collecting data from the source), data transformation (converting data into a consistent format), data validation (checking data accuracy and consistency), and data integration (incorporating validated data into the knowledge base).
We utilize a Git-based version control system to track changes and manage different versions of the database. This ensures that all changes are documented, allowing for easy rollback if necessary. Each data update is treated as a new commit, with clear descriptions of the changes made.
Handling Data Revisions and Corrections
A formal procedure is implemented for handling data revisions and corrections. This involves identifying outdated or incorrect data through regular quality checks and user feedback, documenting the reasons for changes, and ensuring updated information is propagated throughout the knowledge base. A change log tracks all revisions, detailing the date, nature of the change, and the responsible personnel.
Role | Responsibilities |
---|---|
Data Analyst | Identifies outdated or incorrect data; performs data validation; implements corrections. |
Data Manager | Oversees the data revision process; ensures adherence to procedures; approves changes. |
Subject Matter Expert | Reviews data for accuracy and consistency; provides input on resolving conflicting information. |
Data Quality Management and Error Handling
Strategy for Proactive Monitoring
Data quality is proactively monitored using a range of metrics, including completeness, accuracy, and consistency. Predefined thresholds trigger alerts when data quality falls below acceptable levels. Investigations are initiated to identify and resolve quality issues. For example, if the completeness of daily water level data drops below 95%, an alert is generated, prompting an investigation into the cause (e.g., sensor malfunction).
Process for Identifying and Handling Errors
Potential errors or inconsistencies are identified through automated checks and manual reviews. Anomaly detection algorithms identify unusual patterns in the data, triggering further investigation. The causes of errors are determined through data analysis and communication with data providers. Corrective actions range from simple data corrections to more extensive data cleaning or re-processing. For instance, a sudden spike in water temperature readings might indicate a sensor malfunction, requiring data correction or sensor recalibration.
Generating and Disseminating Data Quality Reports
Data quality reports are generated regularly, summarizing key metrics (completeness, accuracy, consistency), highlighting significant issues, and outlining corrective actions. These reports are disseminated to relevant stakeholders (researchers, policymakers, water managers) through email and a dedicated online portal. Reports include a summary of data quality metrics, a list of identified issues and their resolution status, and any planned actions to improve data quality.
Accessibility and Usability
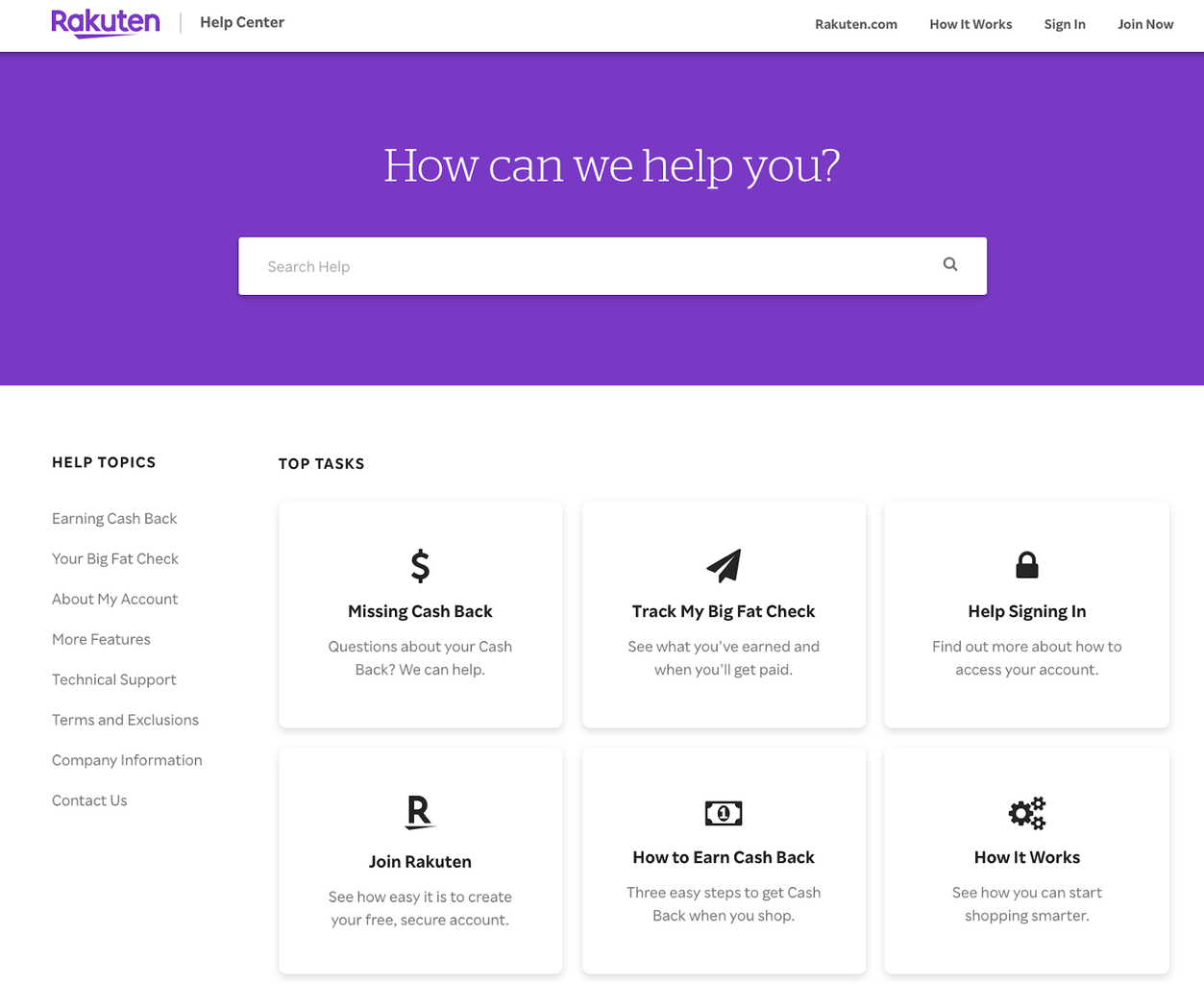
A truly useful waters knowledge base isn’t just about the data; it’s about how easily people can access and use that data. Making it accessible to everyone, regardless of their technical expertise, is key to its success. Think of it like a delicious Palembang pempek – you want everyone to be able to enjoy it, right? So, let’s explore how to make our knowledge base equally appealing and accessible to all.Designing a waters knowledge base for diverse users requires careful consideration of various factors.
We need to ensure that the information is presented in a clear, concise, and understandable manner for users with varying levels of technical knowledge, from seasoned hydrologists to curious citizens. This involves using plain language, avoiding jargon, and providing visual aids where appropriate. Imagine explaining water quality parameters to a child versus a water engineer – the approach must be different but equally effective.
Interface Design and Navigation
A user-friendly interface is crucial for a positive user experience. This means a clean and intuitive layout, clear visual hierarchy, and consistent design elements. Navigation should be straightforward, allowing users to easily find the information they need. Think of it like a well-organized market – you can easily find what you need without getting lost. For example, a clear menu structure with descriptive labels, breadcrumbs to show the user’s location within the site, and prominent search functionality are all essential elements.
A sitemap could also be provided to give users a bird’s eye view of the knowledge base’s structure. Imagine a well-organized menu with clear categories such as “Water Quality,” “Hydrology,” and “Legislation,” allowing users to quickly access relevant information.
Search and Filtering Functionalities
Effective search and filtering functionalities are essential for allowing users to quickly locate specific information within a large dataset. The search function should be robust, allowing for partial matches and wildcard searches. Filtering options should be provided to narrow down search results based on various parameters, such as date, location, or water body type. For instance, a user might search for “water quality data for the Musi River in 2023,” and the system should efficiently return relevant results.
The filters could allow the user to further refine their search by specifying parameters like specific pollutants or data collection methods. Implementing faceted search, where users can refine their results by selecting from a list of attributes, can significantly enhance the user experience.
Security and Privacy Considerations
Protecting the valuable data within a Waters Knowledge Base is paramount. A robust security framework is essential to ensure the confidentiality, integrity, and availability of this crucial information, safeguarding both the data and the reputation of the organization. This involves a multi-layered approach encompassing technical, administrative, and physical security measures.
Privacy concerns are equally critical. The Waters Knowledge Base likely contains sensitive information, potentially including personally identifiable information (PII) or confidential business data. Adherence to relevant data privacy regulations, such as GDPR, CCPA, or others depending on location and data types, is mandatory. Failing to comply can result in significant legal and financial repercussions. Therefore, implementing stringent data protection measures and maintaining transparent data handling practices are vital.
Data Encryption and Access Control
Data encryption is a fundamental security measure. All data stored within the Waters Knowledge Base, both at rest and in transit, should be encrypted using strong, industry-standard encryption algorithms. This prevents unauthorized access even if a breach occurs. Access control mechanisms, implemented through role-based access control (RBAC) or attribute-based access control (ABAC), limit access to authorized personnel only.
This ensures that only individuals with a legitimate need to access specific data can do so. For example, a researcher might have access to raw data, but not the ability to modify it, while an administrator might have full access for maintenance purposes.
Regular Security Audits and Vulnerability Assessments
Regular security audits and vulnerability assessments are crucial for identifying and mitigating potential security risks. These assessments should be conducted by qualified professionals using industry-standard methodologies. This proactive approach helps identify weaknesses in the system’s security posture before they can be exploited by malicious actors. The findings from these audits should be addressed promptly and documented thoroughly. For instance, a vulnerability assessment might reveal a weakness in a web application, prompting the implementation of a security patch or a change in system configuration.
Data Backup and Disaster Recovery
Implementing a robust data backup and disaster recovery plan is essential to ensure business continuity in the event of a system failure or a security incident. Regular backups should be performed and stored securely in a separate location, ideally offline. A comprehensive disaster recovery plan should Artikel procedures for restoring data and systems in the event of a catastrophic event.
This plan should be tested regularly to ensure its effectiveness. A real-world example would be a natural disaster damaging the primary data center; a well-defined plan would ensure minimal downtime by quickly restoring services from the backup location.
User Authentication and Authorization
Strong user authentication mechanisms are vital to prevent unauthorized access. Multi-factor authentication (MFA) should be implemented wherever possible, requiring users to provide multiple forms of authentication before gaining access. Authorization controls should be granular, allowing for fine-grained control over user permissions. This ensures that each user only has access to the data and functionalities necessary for their role.
For instance, a system administrator might have full access, while a data analyst might only have read access to specific datasets.
Compliance with Data Privacy Regulations
Compliance with relevant data privacy regulations is crucial. The Waters Knowledge Base should be designed and managed in accordance with regulations such as GDPR, CCPA, and other applicable laws. This includes implementing procedures for data subject requests, such as access, rectification, and erasure requests. Regular training for staff on data privacy regulations is essential to ensure that everyone understands their responsibilities in protecting sensitive data.
Failure to comply can lead to significant fines and reputational damage.
Applications and Use Cases
A robust water knowledge base offers invaluable support for various applications, significantly impacting decision-making, research, and overall water resource management. Its comprehensive data and analytical capabilities allow for informed strategies and advancements in understanding complex hydrological systems. This section explores specific examples showcasing the practical utility of such a knowledge base across different domains.
Decision-Making in Water Resource Management
A well-structured water knowledge base, brimming with historical data, empowers effective decision-making in water resource management. By analyzing long-term trends and patterns, stakeholders can make informed choices that ensure sustainable water use and mitigate potential risks. The following examples illustrate this potential.
Example | Data Used | Analysis | Recommendation |
---|---|---|---|
Dam Construction Project A: Assessing potential impacts on downstream ecosystems | Rainfall patterns (30 years), river flow rates (30 years), groundwater levels (30 years), downstream ecosystem data (species distribution, water quality) | Statistical analysis of rainfall and river flow data to identify potential changes in flow regimes. Hydrological modeling to simulate dam impacts on downstream water availability and ecosystem health. Comparison with historical ecosystem data to predict potential changes in biodiversity and water quality. | Adjust dam design to minimize negative impacts on downstream flow regimes and ecosystems. Implement mitigation measures to compensate for any predicted losses in biodiversity or water quality. |
Dam Construction Project B: Evaluating water storage capacity and flood risk | Rainfall patterns (30 years), river flow rates (30 years), historical flood events, soil characteristics, topography data | Statistical analysis to identify extreme rainfall events and associated river flows. Hydrological modeling to simulate reservoir filling and potential overflow during extreme events. Risk assessment of flood damage to downstream communities and infrastructure. | Adjust dam height and reservoir capacity to minimize flood risk while maximizing water storage. Develop early warning systems and emergency response plans to mitigate potential flood damage. |
Dam Construction Project C: Determining optimal dam location based on minimizing environmental impact and maximizing water supply | Rainfall patterns (30 years), river flow rates (30 years), groundwater levels (30 years), geological data, biodiversity data, population density data | Spatial analysis to identify optimal dam locations based on maximizing water supply while minimizing impacts on ecologically sensitive areas and human settlements. Cost-benefit analysis to evaluate the economic viability of different dam locations. Multi-criteria decision analysis (MCDA) to integrate various factors into the decision-making process. | Construct the dam at the location that optimizes water supply, minimizes environmental impacts, and satisfies economic constraints. Implement environmental monitoring programs to track the impacts of the dam on the surrounding environment. |
A water knowledge base containing information on water quality parameters and historical pollution incidents is crucial for developing effective drought mitigation strategies. For instance, in California’s Central Valley, such a database can play a pivotal role in predicting water scarcity and identifying vulnerable populations.
- Step 1: Analyze historical water quality data to identify trends and patterns in water availability during drought periods.
- Step 2: Integrate data on pollution incidents to assess their impact on water availability and quality during droughts.
- Step 3: Use predictive modeling techniques to forecast future water scarcity based on climate projections and historical data.
- Step 4: Identify vulnerable populations based on their dependence on water resources and their capacity to cope with water scarcity.
- Step 5: Develop targeted drought mitigation strategies based on the identified vulnerabilities and risks, focusing on water conservation, efficient water allocation, and support for vulnerable communities.
Contribution to Water-Related Research and Scientific Discovery
A comprehensive water knowledge base significantly accelerates water-related research and scientific discovery by providing readily accessible, high-quality data for analysis. This facilitates the investigation of complex hydrological phenomena and their impacts on aquatic ecosystems.
In Lake Erie, a water knowledge base encompassing data on algal blooms, water temperature, and dissolved oxygen levels can address crucial research questions concerning the impact of climate change on aquatic ecosystems. For example, researchers can investigate the correlation between increasing water temperatures and the frequency and intensity of algal blooms. They can also analyze the relationship between dissolved oxygen levels and the health of fish populations.
The expected outcomes include a better understanding of the mechanisms driving algal blooms, improved predictions of bloom events, and the development of effective management strategies to mitigate their impacts on water quality and ecosystem health. This knowledge can inform policies to protect and restore Lake Erie’s ecosystem.
A water knowledge base integrating data from satellite imagery, hydrological models, and field measurements can significantly improve flood forecasting in urban areas like Jakarta. Specifically, satellite imagery provides real-time information on rainfall intensity and river levels. Hydrological models simulate the flow of water through the drainage system, considering factors like rainfall, topography, and land use. Field measurements from rain gauges and water level sensors provide ground truth data to calibrate and validate the model.
Validation methods include comparing model predictions with historical flood events and using statistical metrics to assess the accuracy of the model.
The following flowchart illustrates the workflow of a predictive model for flood forecasting:
[Flowchart Description: The flowchart would begin with data input from various sources (satellite imagery, hydrological models, field measurements). This data would then be pre-processed and integrated. Next, a predictive model (e.g., a statistical model or a machine learning algorithm) would be applied to the integrated data to generate flood forecasts. Finally, the forecasts would be validated using historical flood data and statistical metrics, and the results would be disseminated to stakeholders.]
Successful Applications of Similar Knowledge Bases in Other Fields
The concept of a knowledge base, encompassing structured data and analytical tools, finds successful applications in various fields beyond water resource management. These applications demonstrate the broader applicability and effectiveness of this approach.
Example 1: Medical Diagnosis Support System | Example 2: Financial Risk Assessment System |
---|---|
Structure: Relational database containing patient records, medical images, lab results, and diagnostic information. Uses ontologies to represent medical knowledge and relationships between diseases, symptoms, and treatments. Queries: Supports queries for diagnosis based on symptoms, medical history, and test results. Can also retrieve information on treatment options and prognosis. Impact: Improved diagnostic accuracy, reduced medical errors, and faster diagnosis times. | Structure: A network database integrating financial data from various sources, including market data, company financials, and credit ratings. Uses algorithms and statistical models to assess and predict risks. Queries: Supports queries for credit risk assessment, portfolio optimization, and fraud detection. Impact: Improved risk management, reduced financial losses, and better investment decisions. |
Feature | Weather Forecasting | Water Resource Management |
---|---|---|
Data Sources | Meteorological stations, satellites, radar, weather balloons | Hydrological stations, satellites, rain gauges, groundwater monitoring wells |
Analytical Techniques | Numerical weather prediction models, statistical forecasting methods | Hydrological modeling, statistical analysis, water balance calculations |
Applications | Short-term and long-term weather forecasts, severe weather warnings | Flood forecasting, drought prediction, water allocation planning |
Technological Infrastructure
A robust technological infrastructure is the backbone of any successful waters knowledge base. It ensures efficient data storage, retrieval, analysis, and sharing, ultimately supporting informed decision-making related to water resources management. The right infrastructure will depend on the scale and complexity of the knowledge base, as well as budget considerations. Choosing the appropriate components requires careful planning and consideration of future growth.The technological infrastructure for a waters knowledge base encompasses both hardware and software components working in concert.
Hardware elements include servers (potentially clustered for redundancy and scalability), storage devices (such as SSDs or HDDs, possibly including cloud storage), and network infrastructure enabling efficient data transfer and access. Software components are equally crucial and involve a database management system (DBMS), data visualization tools, user interface (UI) elements, and potentially specialized software for hydrological modeling or GIS integration.
Database Management Systems
Selecting the appropriate database management system is paramount. The choice depends on factors like data volume, data types (e.g., spatial data, time series data), query complexity, and scalability requirements. Several DBMS options exist, each with its strengths and weaknesses.Relational Database Management Systems (RDBMS) like PostgreSQL or MySQL are well-suited for structured data and offer robust query capabilities through SQL.
They are widely used and well-documented, making them a reliable choice for many applications. However, handling unstructured data, such as images or text documents, might require additional considerations or integration with other systems.NoSQL databases, such as MongoDB or Cassandra, are better suited for handling large volumes of unstructured or semi-structured data. Their flexibility makes them ideal for rapidly evolving datasets or applications requiring high scalability and availability.
However, they might lack the structured query capabilities of RDBMS, potentially complicating complex data analysis.A hybrid approach, combining RDBMS and NoSQL databases, could provide a powerful solution for a waters knowledge base containing diverse data types. For example, structured water quality data could be stored in an RDBMS, while unstructured data such as images from drone surveys could be stored in a NoSQL database.
Cloud-Based versus On-Premise Solutions
The decision between a cloud-based and an on-premise solution significantly impacts the infrastructure and its management. Cloud-based solutions, offered by providers like AWS, Azure, or Google Cloud, offer scalability, flexibility, and reduced upfront capital expenditure. They handle infrastructure maintenance and updates, allowing organizations to focus on data management and application development. However, cloud solutions may introduce concerns about data security, vendor lock-in, and potential costs associated with data transfer and storage.On-premise solutions provide greater control over data security and infrastructure but require significant upfront investment in hardware and IT personnel for maintenance and management.
Scalability might also be more challenging and expensive to achieve compared to cloud-based alternatives. The optimal choice depends on factors like budget, security requirements, IT expertise, and the anticipated scale of the waters knowledge base. A cost-benefit analysis, considering both short-term and long-term implications, is essential for making an informed decision. For instance, a smaller organization with limited IT resources might benefit from a cloud-based solution, while a large government agency with stringent security requirements might prefer an on-premise solution.
Collaboration and Knowledge Sharing
A thriving Waters Knowledge Base relies heavily on the collaborative spirit and active participation of its diverse stakeholders. This includes researchers, policymakers, water management professionals, and community members, all contributing their unique expertise and perspectives. Effective collaboration ensures the knowledge base remains current, comprehensive, and relevant to the ever-evolving needs of water resource management. A well-defined framework is crucial for managing this collaborative process smoothly and efficiently.Effective knowledge sharing is the lifeblood of a successful Waters Knowledge Base.
It fosters a sense of community, encourages continuous learning, and ensures that valuable information is readily accessible to all stakeholders. Strategies to promote knowledge sharing must be proactive and engaging, encouraging participation from diverse backgrounds and expertise levels. This includes providing multiple channels for contribution and feedback, acknowledging contributions, and celebrating successes.
A Framework for Collaboration
This framework Artikels roles, responsibilities, and communication channels to ensure efficient collaboration. It emphasizes a transparent and inclusive process, welcoming contributions from all stakeholders. The framework utilizes a multi-tiered approach, incorporating formal governance structures alongside informal, community-driven interactions. For example, a steering committee comprising representatives from key stakeholder groups can oversee the strategic direction of the knowledge base, while dedicated working groups can focus on specific thematic areas or tasks.
Regular meetings, both virtual and in-person, are scheduled to facilitate communication and progress tracking. A project management system is employed to track tasks, deadlines, and individual contributions, ensuring accountability and transparency.
Encouraging Knowledge Sharing and Community Engagement
Several strategies are implemented to cultivate a vibrant and engaged community around the Waters Knowledge Base. These include the creation of a dedicated online forum for discussion and knowledge exchange. This forum will feature moderated discussions, Q&A sessions with experts, and opportunities for users to share their experiences and insights. Regular webinars and workshops are conducted, focusing on relevant topics and providing hands-on training on using the knowledge base.
Incentives, such as recognition for significant contributions or the opportunity to present research findings, are offered to encourage participation. Furthermore, success stories and case studies are showcased to highlight the impact of the knowledge base and inspire further contributions. This creates a positive feedback loop, encouraging continued engagement and collaboration.
Managing Contributions and Feedback
A robust system for managing contributions and feedback is essential for maintaining the quality and relevance of the Waters Knowledge Base. This system involves a clear submission process with guidelines for content format, style, and quality assurance. All submissions are reviewed by designated editors or subject matter experts to ensure accuracy, consistency, and adherence to the knowledge base’s standards.
Feedback mechanisms, including comment sections and rating systems, are integrated into the knowledge base to facilitate user input and iterative improvement. A transparent process for addressing feedback and incorporating suggested changes is established, ensuring that all contributions are valued and considered. Regular audits of the knowledge base are conducted to identify areas for improvement and ensure that the content remains up-to-date and relevant.
Scalability and Future Development
Expanding the Waters Knowledge Base requires a robust plan for scalability and future enhancements. This section details strategies to ensure the knowledge base remains efficient, accurate, and accessible as it grows, incorporating advanced technologies to unlock its full potential. We’ll explore data ingestion techniques, optimal database architectures, infrastructure scaling, and future features, including advanced analytics and AI/ML integration.
Data Ingestion Strategies
Efficient data ingestion is crucial for a scalable knowledge base. Several methods exist, each with its strengths and weaknesses. Choosing the right approach depends on factors such as data volume, velocity, and variety. The following table compares three popular approaches:
Method | Description | Pros | Cons | Example Technology |
---|---|---|---|---|
Batch Processing | Data is collected and processed in batches at regular intervals. | Cost-effective for large, static datasets; simpler to implement. | Data latency; not suitable for real-time applications. | Apache Spark |
Real-time Streaming | Data is processed as it arrives, enabling immediate analysis and response. | Low latency; suitable for real-time monitoring and alerts. | Higher infrastructure costs; complex implementation. | Apache Kafka |
Cloud-based Data Pipelines | Managed services that automate data ingestion, transformation, and loading. | Scalable and managed; easy integration with other cloud services. | Vendor lock-in; potential cost increases with data volume. | AWS Glue, Azure Data Factory |
Data validation and cleaning are critical steps to ensure data quality. This involves checking for inconsistencies, missing values, and errors, and applying appropriate transformations to standardize the data. Error handling mechanisms, such as retry logic and dead-letter queues, should be implemented to ensure data integrity.
Database Design for Scalability
A NoSQL database, specifically a document database like MongoDB, is recommended for this application. NoSQL databases offer superior scalability compared to relational databases when dealing with large, semi-structured datasets like those commonly found in water resource management. The flexibility of document databases allows for easy schema changes as the knowledge base evolves. Data partitioning and sharding can further enhance scalability by distributing the data across multiple servers.
This approach addresses the diverse data types and relationships within the knowledge base efficiently.
Understanding water’s knowledge base requires exploring the intricate relationships within its systems. To grasp the theoretical underpinnings, a helpful resource is this presentation on knowledge-based theory, knowledge based theory ppt , which offers valuable insights into the framework for analyzing complex systems. Applying this framework, we can better understand the dynamic nature of water’s knowledge base and its implications.
Infrastructure Scalability Strategies
Cloud-based solutions, such as those offered by AWS, Azure, or GCP, provide the necessary scalability and flexibility. A serverless architecture can further optimize resource utilization and reduce costs. AWS is a suitable choice due to its mature services and extensive ecosystem for data processing and analytics. Load balancing distributes traffic across multiple servers to prevent overload. Auto-scaling automatically adjusts the number of servers based on demand, ensuring optimal performance.
Redundancy mechanisms, such as geographically distributed backups, protect against data loss.
Advanced Analytics Capabilities
Predictive modeling for water quality, using machine learning algorithms like Random Forests or Gradient Boosting, can forecast future water quality parameters based on historical data and environmental factors. Anomaly detection, using techniques like time series analysis, can identify unusual patterns in water quality data, potentially indicating pollution events or equipment malfunctions. Spatial analysis, utilizing GIS platforms, can visualize water quality data geographically, identifying areas of concern and facilitating targeted interventions.
These enhancements require integrating data from various sources, including historical water quality measurements, weather data, and hydrological models.
UI/UX Improvements
Improved searchability can be achieved through advanced search algorithms and faceted search capabilities. Data visualization can be enhanced through interactive dashboards and charts, allowing users to explore data effectively. Accessibility improvements should focus on WCAG compliance, providing support for users with disabilities. Specific UI/UX design patterns, such as card-based layouts and intuitive navigation menus, can improve user experience.
Integration with External Systems
Integrating weather data APIs can provide real-time meteorological information, enriching the context of water quality data. Integrating hydrological models allows for simulating water flow and predicting water levels. GIS platforms facilitate spatial analysis and visualization of water-related data. Technical challenges include data format differences and API limitations. Solutions include data transformation tools and custom API wrappers.
AI/ML Integration
Natural language processing (NLP) can be used to analyze unstructured text data, such as reports and scientific publications, extracting valuable information and enriching the knowledge base. Machine learning algorithms can be used for predictive modeling, anomaly detection, and classification tasks. Specific algorithms like Support Vector Machines (SVMs) or Neural Networks can be applied to various water-related problems.
Knowledge Graph Implementation
Implementing a knowledge graph, using technologies like Neo4j or Amazon Neptune, can improve data discoverability and reasoning capabilities by representing the relationships between different entities and concepts within the water knowledge base. This will facilitate more complex queries and enable the discovery of hidden relationships within the data.
Semantic Web Technologies
Leveraging semantic web technologies like RDF and OWL can improve data interoperability and knowledge representation. RDF triples can represent relationships between water-related entities, while OWL ontologies can define the vocabulary and semantics of the knowledge base. This will enhance data querying and reasoning, enabling more sophisticated knowledge discovery and analysis.
Integration with Other Systems
Integrating the Waters Knowledge Base with other relevant systems is crucial for maximizing its value and ensuring seamless data flow within a larger organizational context. This integration allows for richer insights, improved decision-making, and enhanced efficiency across various departments and functions. Effective integration strategies will leverage existing infrastructure and minimize disruption to ongoing operations.The Waters Knowledge Base can be integrated with various systems using several methods, each chosen based on the specific needs and technical capabilities of the systems involved.
These methods ensure that data can be shared efficiently and effectively, preventing data silos and promoting a unified view of water-related information. Careful consideration of data formats and security protocols is essential during the integration process.
Data Exchange Methods
Several methods facilitate data exchange with external sources. Application Programming Interfaces (APIs) provide a standardized way for different systems to communicate and share data, allowing for automated updates and real-time data synchronization. Data can also be exchanged through file transfers, such as CSV or XML files, although this method may be less efficient for large datasets or frequent updates.
Database replication, mirroring changes from one database to another, ensures data consistency across systems. Finally, ETL (Extract, Transform, Load) processes can be used to move data between systems, performing necessary transformations during the transfer.
Benefits of Interoperability and Data Sharing
Interoperability, the ability of different systems to exchange and use information, brings numerous benefits. Reduced data redundancy minimizes storage costs and reduces the risk of inconsistencies. Improved data quality results from having a single source of truth, leading to better decision-making. Enhanced collaboration fosters better communication and information sharing among stakeholders. Increased efficiency streamlines workflows and reduces manual data entry, freeing up staff for more strategic tasks.
Finally, cost savings are realized through the optimization of resources and reduced operational overhead. For example, integrating the Waters Knowledge Base with a Geographic Information System (GIS) allows for visualizing water resource data geographically, leading to improved water management strategies and more effective responses to water-related emergencies. Similarly, integration with a weather forecasting system enables proactive measures to mitigate the impact of extreme weather events on water resources.
Cost-Benefit Analysis
A thorough cost-benefit analysis (CBA) is crucial for justifying the investment in a water knowledge base. This analysis will demonstrate the long-term value and return on investment, highlighting the economic and societal benefits that outweigh the initial and ongoing costs. The following sections detail a framework for conducting a comprehensive CBA, considering both tangible and intangible factors.
Framework for Cost-Benefit Analysis
A robust framework is essential for a reliable CBA. This framework involves a systematic approach to identify, quantify, and evaluate the costs and benefits associated with establishing and maintaining a water knowledge base. The process incorporates methods for handling uncertainty and presenting the findings in a clear and understandable manner. The steps Artikeld below provide a comprehensive guide.
- Identifying and Defining Relevant Costs and Benefits: This initial step involves a comprehensive inventory of all potential costs (development, implementation, maintenance) and benefits (improved decision-making, cost savings, enhanced research). This requires input from stakeholders across various sectors.
- Quantifying Tangible and Intangible Costs and Benefits: Tangible costs and benefits are easily measured in monetary terms (e.g., software licenses, reduced water bills). Intangible benefits, such as improved public trust or enhanced environmental protection, require more qualitative assessment and potential monetary valuation techniques.
- Discounting Future Costs and Benefits to Present Value: Future costs and benefits must be discounted to their present value using an appropriate discount rate to account for the time value of money. This ensures a fair comparison between present and future cash flows.
- Assessing the Uncertainty Associated with Cost and Benefit Estimations: Uncertainty is inherent in any forecasting exercise. Sensitivity analysis will be performed to assess the impact of variations in key assumptions on the overall CBA results.
- Presenting the Results in a Clear and Concise Manner, Including a Sensitivity Analysis: The final report should clearly present the findings in a user-friendly format, including tables, charts, and a detailed sensitivity analysis. This allows stakeholders to easily understand the results and their implications.
Quantifying Benefits
The benefits of a water knowledge base are substantial and far-reaching. They span improved decision-making, significant cost savings, and enhanced research capabilities across various sectors.
- Improved Decision-Making: A well-structured knowledge base facilitates data-driven decision-making, leading to more efficient water resource management. For example, a municipality could reduce water leakage by 15% (based on a similar project in Singapore) resulting in annual savings of $500,000 based on current water costs. This represents a quantifiable benefit.
- Cost Savings: Improved water management practices, enabled by the knowledge base, lead to cost reductions in agriculture (optimized irrigation), industry (reduced water consumption in manufacturing), and municipalities (leak detection and repair). For instance, an industrial plant might reduce water usage by 10%, translating to annual savings of $200,000 based on their current water bill.
- Enhanced Research: The knowledge base streamlines research by providing easy access to relevant data, reducing research time and accelerating the pace of discovery. For example, a research team might reduce their data collection time by 50%, saving approximately 6 months of researcher salaries and accelerating publication by at least one year, leading to a potential increase in research grants and other funding opportunities.
Detailing Costs
Developing, implementing, and maintaining a water knowledge base involves significant costs. A detailed breakdown is necessary for a comprehensive CBA.
- Development Costs: These include data acquisition ($50,000 – estimated cost for data cleaning and integration), database design and implementation ($100,000 – estimated cost based on similar projects), software development ($150,000 – estimated cost for custom software development), and personnel costs (data scientists, engineers: $200,000 annual salary for a team of 5 for one year).
- Implementation Costs: This includes training personnel ($20,000 – estimated cost for training 10 personnel), system integration ($30,000 – estimated cost for integration with existing systems), and initial data population ($10,000 – estimated cost for data entry and validation).
- Maintenance Costs: Ongoing maintenance, data updates, software upgrades, and personnel costs (system administrators, data curators) are projected over 5 and 10 years. Annual maintenance costs are estimated at $50,000, including software licenses, cloud storage, and personnel salaries.
Presentation of Findings
The CBA results will be presented in a comprehensive report, including a summary table comparing total costs and benefits, a graphical representation of the cost-benefit ratio over time, and a sensitivity analysis. This will clearly demonstrate the financial viability and long-term value of the water knowledge base. For example, a Net Present Value (NPV) calculation will be performed, considering a discount rate of 5% over a 10-year period.
The report will include scenarios reflecting variations in key cost and benefit assumptions (e.g., higher or lower water usage reduction rates, changes in personnel costs).
Consideration of Intangible Factors
Intangible benefits, such as improved public trust and enhanced environmental protection, are crucial but challenging to quantify. Qualitative assessments, incorporating expert opinions and stakeholder feedback, will be used to capture their importance. Methods like contingent valuation or scoring systems can be employed to incorporate these intangible factors into the overall CBA. For example, improved public trust could be indirectly quantified by surveying public opinion and relating changes in trust to potential increases in tourism or property values.
Data Sources and Assumptions
The CBA relies on various data sources and assumptions, as detailed in the table below. The justification for each assumption is based on available data, expert opinions, and similar projects.
Data Source Category | Specific Data Source Examples | Assumptions Made | Justification for Assumptions |
---|---|---|---|
Water Usage Data | Government agencies, utility companies, agricultural surveys | Average water usage per sector remains consistent for the first 5 years, then increases by 2% annually. | Based on historical data and projected growth rates for the region. |
Cost Data | Market research, vendor quotes, internal cost estimates | Inflation rates remain at an average of 3% annually. | Based on current economic forecasts for the region. |
Benefit Data | Expert interviews, case studies, literature reviews | Success rates of similar projects are comparable and applicable to this context. | Based on a thorough review of relevant literature and expert consultations. |
Case Studies
This section presents three case studies showcasing successful implementations of waters knowledge bases, highlighting key success factors and lessons learned for future endeavors. These examples demonstrate the practical application and benefits of a well-structured and accessible water knowledge base.
Case Study 1: Improved Water Management in the Mekong Delta
The Mekong Delta, a crucial agricultural region, faced challenges in managing its water resources due to seasonal variations, saltwater intrusion, and increasing demand. A comprehensive water knowledge base was developed, integrating data from various sources including hydrological models, satellite imagery, rainfall data, and local community knowledge. This centralized repository enabled improved water resource allocation, optimized irrigation strategies, and enhanced early warning systems for floods and droughts.
The knowledge base facilitated better collaboration between government agencies, researchers, and farmers, leading to more informed decision-making and improved water security. The system’s success was attributed to its user-friendly interface, the comprehensive integration of diverse data sources, and the active involvement of local communities in data collection and knowledge sharing.
Case Study 2: Enhanced Water Quality Monitoring in the Great Lakes
The Great Lakes region implemented a water quality knowledge base to monitor and manage pollution sources, track water quality trends, and support informed policy decisions. The system integrated data from various monitoring stations, citizen science initiatives, and research studies, providing a comprehensive overview of water quality parameters across the region. This enabled effective identification of pollution hotspots, timely interventions to address water quality issues, and better assessment of the effectiveness of pollution control measures.
The key to the success of this implementation was the standardization of data collection methods, the development of robust data quality control procedures, and the creation of user-friendly visualization tools that facilitated easy access and interpretation of complex data.
Case Study 3: Optimized Water Distribution in a Metropolitan Area
A large metropolitan area improved its water distribution efficiency by implementing a water knowledge base that integrated data from various sources including water meters, sensor networks, and customer service records. This system provided real-time insights into water consumption patterns, identified leaks and inefficiencies, and supported proactive maintenance of the water distribution infrastructure. The knowledge base facilitated the optimization of water pressure management, reduced water loss, and improved the overall reliability of the water supply.
The project’s success stemmed from the seamless integration of data from diverse sources, the development of sophisticated analytical tools, and the implementation of a robust data security framework.
Ethical Considerations: Waters Knowledge Base
Building a robust and reliable Waters Knowledge Base requires careful consideration of ethical implications alongside technical aspects. The responsible collection, storage, and use of water-related data are paramount to ensuring fairness, transparency, and the overall benefit of the community. Ignoring ethical considerations can lead to biased results, misinformed decisions, and a loss of public trust.Data Integrity and Bias MitigationEnsuring the accuracy and reliability of data within the Waters Knowledge Base is crucial.
Data integrity involves maintaining the completeness, consistency, and accuracy of information throughout its lifecycle. Bias, whether conscious or unconscious, can significantly skew results and lead to flawed conclusions. Strategies for mitigating bias include diverse data collection methods, rigorous data validation procedures, and employing statistical techniques to identify and correct for potential biases. For example, using multiple independent sources for rainfall data and comparing them against established meteorological station readings can help identify and correct outliers or inconsistencies, thus minimizing bias.
Employing a standardized protocol for data collection and processing across all contributing sources also significantly improves data integrity.Transparency and Accountability MechanismsTransparency and accountability are fundamental to building trust and ensuring the ethical use of the Waters Knowledge Base. This involves making the data collection methods, data processing techniques, and decision-making processes readily available for scrutiny. Detailed documentation of data sources, methodologies, and any limitations should be publicly accessible.
A clear chain of custody for data, along with mechanisms for tracking changes and resolving discrepancies, fosters accountability. Regular audits of the data and processes within the Knowledge Base can help identify and rectify potential issues. Establishing an independent review board to oversee the ethical considerations of the Knowledge Base could further enhance transparency and accountability. For example, publishing a detailed methodology document outlining the data sources used for water quality assessments, the algorithms employed for analysis, and any assumptions made would ensure complete transparency.
This would allow independent researchers or stakeholders to verify the findings and assess the validity of the conclusions.
FAQ Section
What are the limitations of using solely publicly available data sources for a waters knowledge base?
Publicly available data often suffers from inconsistencies in format, quality, and temporal/spatial resolution. It may also lack crucial data points due to restricted access to certain datasets. Relying solely on public sources risks creating an incomplete or biased knowledge base.
How can bias be mitigated in a waters knowledge base?
Bias mitigation requires a multi-faceted approach. This includes critically evaluating data sources for potential biases, employing diverse data sources to offset individual biases, using transparent data processing techniques, and actively involving diverse stakeholders in the design and implementation of the knowledge base.
What is the role of metadata in a waters knowledge base?
Metadata is crucial for discoverability and usability. Comprehensive metadata allows users to easily search, filter, and understand the context of data within the knowledge base, improving data quality and enabling more effective analysis.
How can the long-term maintainability of a waters knowledge base be ensured?
Long-term maintainability requires a well-defined data governance structure, robust data management procedures, a scalable technological infrastructure, and a dedicated team responsible for data updates, quality control, and system maintenance. Regular audits and evaluations are also essential.