How does a scientific theory differ from a scientific hypothesis? That’s a question that gets to the heart of how science actually works. It’s not just about making educated guesses; it’s about building a robust understanding of the world around us, a process that involves rigorous testing, evidence gathering, and a whole lot of debate. We’ll dive into the specifics of hypotheses and theories, exploring how they’re formed, tested, and ultimately contribute to our ever-evolving scientific knowledge.
A hypothesis is essentially an educated guess, a testable statement proposing a possible explanation for an observation. Think of it as a starting point for investigation, a specific, measurable prediction about what might happen under certain conditions. A theory, on the other hand, is a much broader explanation supported by a large body of evidence. It’s not just a hunch; it’s a well-substantiated explanation that has withstood rigorous testing and incorporates multiple related hypotheses.
We’ll explore the key differences between these two crucial elements of the scientific method, looking at their scope, testability, and the role they play in scientific progress.
Defining a Scientific Hypothesis
A scientific hypothesis, my friends, is not just a wild guess thrown into the scientific blender. It’s a much more sophisticated beast, a carefully crafted statement that proposes a possible explanation for an observation. Think of it as an educated hunch, but one that’s ready to roll up its sleeves and get to work. It’s the starting point for a journey of scientific discovery, a journey that may lead to thrilling revelations or humbling corrections.
Characteristics of a Well-Formed Hypothesis
A well-formed hypothesis possesses several key characteristics. Firstly, and perhaps most importantly, it must be falsifiable. This means that it must be possible to design an experiment or observation that could, in principle, disprove the hypothesis. A hypothesis that cannot be disproven is essentially untestable and therefore useless in the scientific realm.For example, “All swans are white” is a falsifiable hypothesis; the observation of a single black swan would disprove it.
Conversely, “There is a teapot orbiting the sun too small to be observed” is not falsifiable. No amount of observation can definitively prove or disprove its existence, making it a rather unhelpful proposition. This is why we should leave the teapots in the kitchen.Clarity and precision are also paramount. Vague or ambiguous hypotheses lead to ambiguous results.
For instance, “Social media affects teenagers” is far too broad. A better hypothesis might be: “Increased daily use of Instagram is correlated with increased levels of anxiety in adolescents aged 13-17.” See the difference? The latter is precise, measurable, and leaves less room for interpretive dance.The relationship between a hypothesis and a prediction is crucial. A prediction is a specific, testable statement derived from the hypothesis.
While the hypothesis proposes an explanation, the prediction states what we expect to observe if the hypothesis is correct. For example, if our hypothesis is “Plants grow taller with increased sunlight,” a prediction might be: “Plants exposed to 12 hours of sunlight will be taller than plants exposed to 6 hours of sunlight after two weeks.” The prediction specifies a measurable outcome under defined conditions.
Examples of Testable Hypotheses
Let’s illustrate with some real-world examples. The following table presents testable hypotheses from various scientific fields, complete with independent and dependent variables and potential methodologies. Note that the methodology is a suggestion and the actual methods used will vary based on resources and experimental design.
Scientific Field | Hypothesis | Independent Variable | Dependent Variable | Potential Methodology |
---|---|---|---|---|
Biology | Increased sunlight exposure leads to faster plant growth. | Sunlight Exposure (hours/day) | Plant Growth Rate (cm/week) | Controlled experiment with varying light levels, using identical plant species and soil conditions. Measure plant height weekly. |
Physics | The velocity of a falling object is directly proportional to its mass. | Mass of Object (kg) | Velocity of Fall (m/s) | Controlled experiment using various masses dropped from the same height, measuring the time it takes to reach the ground. Calculate velocity. |
Psychology | Exposure to violent video games increases aggression. | Exposure to Violent Video Games (hours/week) | Aggression Levels (score on standardized aggression scale) | Experiment measuring aggression levels (using a validated aggression scale) before and after participants play violent video games for a set period. Control group plays non-violent games. |
The Role of a Hypothesis in the Scientific Method
The hypothesis is the heart of the scientific method, the engine that drives the entire process. It acts as a bridge connecting observation and experimentation. The process can be visualized as follows:[Imagine a flowchart here: Observation –> Question –> Hypothesis –> Prediction –> Experiment –> Analysis –> Conclusion. Arrows connect each stage.]The hypothesis guides the design of the experiment by specifying the variables to be manipulated (independent) and measured (dependent).
It dictates what data to collect and how to analyze it.If the experiment supports the hypothesis, the hypothesis is strengthened, but not proven. Further testing and investigation are always necessary. If the experiment refutes the hypothesis, it doesn’t mean failure! It provides valuable insight, directing future research towards new hypotheses or refinements of existing theories.* Hypothesis Supported: The results generally align with the predictions.
This strengthens the hypothesis, but more research is needed for confirmation. The hypothesis might be refined or used to develop a more comprehensive theory.
Hypothesis Refuted
The results contradict the predictions. This doesn’t invalidate the entire research process. The hypothesis is revised or discarded, leading to the formulation of new hypotheses and further investigation. This is a crucial step in scientific progress.
A refuted hypothesis does not necessarily mean the end of the investigation. It provides valuable information, guiding further research and potentially leading to the refinement of existing theories or the development of new ones.
Defining a Scientific Theory: How Does A Scientific Theory Differ From A Scientific Hypothesis
A scientific theory, unlike its often-misunderstood cousin the hypothesis, isn’t just a hunch or a guess. It’s a robust, well-substantiated explanation of some aspect of the natural world, built upon a mountain of evidence and refined through rigorous testing. Think of it as the scientific equivalent of a well-aged, award-winning Cabernet Sauvignon – complex, nuanced, and deeply satisfying.
Components of a Robust Scientific Theory
The creation of a robust scientific theory is a monumental task, akin to building a skyscraper from meticulously crafted bricks. Several essential components must be in place for a theory to stand the test of time and earn its place in the pantheon of scientific understanding. These components are not mutually exclusive; they work in concert to form a cohesive and compelling explanation.
- Power: A strong theory provides a comprehensive explanation for a wide range of observations and phenomena. For instance, the Theory of Evolution explains the diversity of life on Earth, from the smallest bacteria to the largest whales, through the mechanism of natural selection. It doesn’t just explain
-that* species change; it explains
-how* they change over time.The Theory of Relativity, similarly, explains gravity not as a force but as a curvature of spacetime, elegantly unifying gravity with other forces.
- Predictive Accuracy: A robust theory makes testable predictions about future observations or experiments. The Theory of Relativity predicted the bending of light around massive objects, a prediction later confirmed during a solar eclipse. Similarly, the Theory of Evolution predicted the existence of transitional fossils, linking different species over evolutionary time, and these predictions have been repeatedly verified through fossil discoveries.
- Falsifiability: This crucial component means that a theory must be potentially disprovable. A theory that explains everything explains nothing. While the Theory of Evolution has withstood countless attempts at falsification, its predictions remain testable. The same applies to the Big Bang theory; its predictions about the cosmic microwave background radiation, for example, are subject to ongoing verification and potential refutation.
- Coherence and Consistency: A strong theory is internally consistent and aligns with other well-established scientific theories. For example, the Theory of Evolution is consistent with the principles of genetics and molecular biology. The Theory of Plate Tectonics, which explains the movement of Earth’s continental plates, is consistent with geological observations like earthquake patterns and the distribution of fossils.
- Empirical Support: A scientific theory is not built on speculation; it’s built on evidence. The Theory of Evolution is supported by an overwhelming amount of evidence from fields like paleontology, genetics, comparative anatomy, and biogeography. Similarly, the Theory of Relativity is supported by a vast body of experimental data, including observations of gravitational lensing and the precise measurement of time dilation.
Comparison of Strong and Weak Scientific Theories
Characteristic | Strong Theory | Weak Theory |
---|---|---|
Power | Explains a broad range of phenomena comprehensively | Explains only a limited number of phenomena or offers incomplete explanations |
Predictive Accuracy | Makes accurate and testable predictions | Makes inaccurate or untestable predictions |
Falsifiability | Is potentially disprovable through observation or experiment | Is not testable or is immune to falsification |
Scope | Applies to a wide range of contexts and situations | Applies only to a narrow range of contexts |
Theory Development: Evidence Gathering and Peer Review
The journey from a hunch to a widely accepted theory is a long and winding road, paved with rigorous investigation and critical evaluation. The process involves a series of carefully considered steps, akin to assembling a complex jigsaw puzzle, one piece of evidence at a time.
- Observation and Question Formulation: Scientists observe phenomena and formulate questions about the natural world. For example, observing the diversity of life could lead to questions about how this diversity arose.
- Hypothesis Formation: A testable hypothesis is proposed to answer the question. For instance, the hypothesis that species change over time due to natural selection.
- Evidence Gathering: Scientists gather evidence to test the hypothesis using various methods. This might involve observational studies (e.g., studying fossil records), experiments (e.g., breeding experiments with fruit flies), or simulations (e.g., climate modeling). Different disciplines will favor different methods. For example, astronomers rely heavily on observational data, while chemists often employ controlled experiments.
- Analysis and Interpretation: The collected data is analyzed to determine whether it supports or refutes the hypothesis. Statistical methods are frequently used to assess the significance of the findings.
- Theory Development: If a hypothesis consistently withstands testing and accumulates substantial support, it may evolve into a theory. This process is iterative, with ongoing refinement and modification as new evidence emerges.
- Peer Review and Publication: The findings are subjected to rigorous peer review before publication in scientific journals. This process involves critical evaluation by experts in the field to ensure the validity and rigor of the research.
A flowchart illustrating the peer-review process might show a manuscript being submitted to an editor, then sent to reviewers who assess its methodology, results, and conclusions. Reviewers provide feedback, which the editor and author use to revise the manuscript. Potential biases include the reviewers’ own preconceived notions or affiliations, but double-blind review (where neither the reviewers nor the author know each other’s identities) can mitigate this.Examples of theories that have evolved include the Germ Theory of Disease, which initially focused on bacteria but expanded to include viruses and other pathogens, and the Big Bang Theory, which has been refined through observations of cosmic microwave background radiation and the accelerating expansion of the universe.
Power vs. Predictive Power
” power” refers to a theory’s ability to provide a comprehensive and coherent explanation for observed phenomena. “Predictive power,” on the other hand, refers to a theory’s ability to accurately forecast future observations or outcomes. While related, they are distinct concepts. A theory might explain a phenomenon well but be poor at making precise predictions, or vice-versa.Examples of theories with strong power include the Theory of Evolution (explaining biodiversity), Plate Tectonics (explaining earthquakes and mountain formation), and the Germ Theory of Disease (explaining infectious illnesses).
Examples of hypotheses with strong predictive power include predictions about the trajectory of a comet based on its current velocity and gravitational influences, predictions about the outcome of a clinical trial based on a new drug’s mechanism of action, and weather forecasts based on atmospheric models.A theory might have strong power but weak predictive power if the underlying mechanisms are complex and difficult to model precisely.
For example, while the theory of climate change explains the general warming trend, predicting the precise impacts on specific regions remains challenging. Conversely, a hypothesis might have strong predictive power but limited power if it accurately predicts an outcome without fully explaining the underlying cause. For example, a statistical model might accurately predict stock market fluctuations without explaining the fundamental economic drivers.A theory’s power is intrinsically linked to its falsifiability.
A scientific hypothesis is a testable prediction, while a theory is a well-substantiated explanation encompassing many observations. Understanding this distinction is crucial; for instance, consider how drive theory, as explained in this helpful resource, what is the main idea of drive theory , posits internal states motivating behavior. This contrasts with a hypothesis which might predict a specific behavioral response under certain conditions, highlighting the broader scope of a scientific theory.
A theory that explains everything is untestable and therefore not scientific. The assessment of power is subjective, but it relies on the extent to which the theory accounts for the existing evidence, its consistency with other theories, and its ability to generate new hypotheses.
Scope and Breadth
Let’s delve into the fascinating world of scientific scope – where hypotheses tiptoe tentatively and theories strut their stuff with confident swagger. Think of it as the difference between a single, perfectly formed snowflake (hypothesis) and a blizzard (theory) – both made of the same stuff, but with vastly different impact and scale.A hypothesis, dear reader, is a modest affair.
It’s a focused, testable prediction about a specific phenomenon. It’s like a finely-tuned instrument playing a single, exquisite note. In contrast, a scientific theory is a majestic symphony, a sprawling composition built upon a mountain of evidence, explaining a broad range of observations. It’s a robust explanation encompassing numerous interconnected hypotheses, each contributing a harmonious note to the overall melody.
Hypothesis Scope Versus Theory Scope
The key difference lies in the level of explanation and the range of phenomena addressed. A hypothesis tackles a narrow, specific question; a theory provides a comprehensive framework capable of explaining many related observations. Consider the hypothesis: “Plants exposed to higher concentrations of CO2 will exhibit increased growth rates.” This is a focused prediction, easily tested in a controlled experiment.
Now, compare this to the theory of evolution by natural selection. This theory doesn’t just explain the growth of plants in elevated CO2; it explains the diversity of life on Earth, from the tiniest bacteria to the largest whales. It’s a far grander, more sweeping explanation.
Comparison of Scope and Power
The following table summarizes the key differences in scope and power between a hypothesis and a theory. We’ve avoided overly technical jargon, opting instead for a delightfully accessible explanation, because who needs more jargon in their lives?
Feature | Hypothesis | Theory | Illustrative Example |
---|---|---|---|
Scope | Narrow, specific | Broad, encompassing | Specific effect of fertilizer on plant growth vs. the entire field of plant biology |
Power | Explains a limited set of observations | Explains a wide range of observations and phenomena | Predicting the yield of a single crop vs. predicting the long-term impact of climate change on global agriculture |
Testability | Easily testable through specific experiments | Supported by a vast body of evidence from multiple lines of inquiry | A single controlled experiment vs. decades of research across various disciplines |
Predictive Power | Makes specific predictions about a limited set of variables | Makes broad predictions about a wide range of phenomena, often with high accuracy | Predicting the outcome of a single chemical reaction vs. predicting the trajectory of a hurricane |
Evidence and Support

The relationship between evidence and scientific hypotheses and theories is, dare we say, electrifying! Evidence is the lifeblood of scientific progress, the fuel that propels our understanding of the universe from tentative guesses to robust explanations. It’s the ultimate judge and jury, deciding the fate of our hypotheses and shaping the evolution of our theories. Let’s delve into the fascinating interplay between evidence and the scientific method.
How Evidence Supports or Refutes a Hypothesis
Evidence either bolsters or dismantles a hypothesis through rigorous examination. Supporting evidence aligns with the predictions of the hypothesis, strengthening its plausibility. Conversely, refuting evidence contradicts these predictions, casting doubt on the hypothesis’s validity. This isn’t a simple “yes” or “no” situation; it’s a nuanced dance of probabilities and statistical significance.
Hypothesis Support Example: Let’s say our hypothesis is: “Increased exposure to sunlight increases vitamin D levels in humans.”
- Evidence 1: A study measuring vitamin D levels in individuals with varying sun exposure shows a statistically significant positive correlation (p <0.05). This supports the hypothesis because it demonstrates that as sun exposure increases, so do vitamin D levels, as predicted.
- Evidence 2: Blood tests reveal higher average vitamin D levels in individuals living in sunny climates compared to those in less sunny regions. This corroborates the hypothesis, suggesting a link between environmental sunlight and vitamin D synthesis.
- Evidence 3: A controlled experiment where participants are randomly assigned to different sun exposure groups demonstrates significantly higher vitamin D levels in the group with increased sun exposure. This provides strong causal evidence, further strengthening the hypothesis.
Hypothesis Refutation Example: Now, let’s consider the hypothesis: “All swans are white.”
- Evidence 1: The observation of a single black swan. This single observation is sufficient to refute the hypothesis because it directly contradicts the claim that
-all* swans are white. The presence of even one black swan proves the hypothesis false. - Evidence 2: A comprehensive survey of swan populations across various geographic locations reveals a small but significant percentage of non-white swans (black, grey, etc.). This statistical data refutes the hypothesis, demonstrating that the initial claim is not universally true.
- Evidence 3: Genetic analysis of swan populations reveals multiple distinct genetic lineages, some of which result in non-white plumage. This provides a biological mechanism explaining the existence of non-white swans, further solidifying the refutation of the original hypothesis.
The strength of evidence is often quantified using statistical measures like p-values (for example, p <0.05 indicates a statistically significant result). However, even statistically significant results can be influenced by biases, such as sampling bias (non-representative sample) or confirmation bias (favoring evidence that supports pre-existing beliefs). A rigorous methodology and careful consideration of potential biases are crucial for accurate interpretation.
Testability and Falsifiability
A scientific hypothesis, much like a particularly stubborn houseguest, needs to be put to the test. Similarly, a scientific theory, while enjoying a more permanent residence in the scientific community, must also withstand rigorous scrutiny. Without testability and falsifiability, both hypotheses and theories are merely interesting ideas, adrift in a sea of speculation, rather than sturdy vessels navigating the currents of scientific discovery.Testability and falsifiability are the cornerstones of the scientific method, ensuring that our understanding of the world is based on evidence, not wishful thinking.
A testable hypothesis or theory allows us to design experiments or observations that could potentially disprove it. This seemingly counterintuitive approach—actively seeking to refute a claim—is what allows science to progress, weeding out the weak ideas and strengthening the robust ones. Think of it as a scientific version of natural selection: only the fittest ideas survive.
Testability in Hypotheses and Theories
A hypothesis must be formulated in a way that allows for empirical testing. This means that it must be possible to collect data that either supports or refutes the hypothesis. For instance, the hypothesis “Cats prefer tuna to salmon” is testable because we can design an experiment where we offer cats both types of fish and observe their preferences.
Similarly, a scientific theory, being a well-substantiated explanation of some aspect of the natural world, must also be testable through ongoing research and experimentation. Einstein’s theory of relativity, for example, has been tested countless times through observations of celestial bodies and particle physics experiments, consistently supporting its predictions (although it’s not without its limitations and refinements).
Falsifiability: The Power of Disproof
Falsifiability is the crucial element that distinguishes scientific claims from non-scientific ones. A falsifiable statement is one that could, in principle, be proven wrong. This doesn’t mean itis* wrong, but that it’s possible to imagine an observation or experiment that would contradict it. The statement “All swans are white” was falsifiable and was indeed falsified upon the discovery of black swans in Australia.
In contrast, the statement “God exists” is not easily falsifiable because no conceivable observation could definitively disprove it. The importance of falsifiability lies in its ability to drive scientific progress. By attempting to falsify a hypothesis or theory, scientists refine and strengthen their understanding, ultimately leading to a more accurate and comprehensive picture of the world.
Testing a Hypothesis: A Step-by-Step Example
Let’s consider the hypothesis: “Plants grow taller in sunlight than in darkness.”
1. State the Hypothesis
Clearly define the hypothesis: Plants exposed to sunlight will exhibit greater height growth compared to plants kept in darkness.
2. Design the Experiment
Obtain two identical groups of seedlings (to minimize confounding variables). One group will be placed in a sunny location, the other in a completely dark environment. Control other variables like water, soil type, and temperature.
3. Collect Data
Regularly measure the height of each seedling over a specific period (e.g., four weeks). Record the data meticulously in a table.
4. Analyze Data
Calculate the average height for each group. Use statistical tests (like a t-test) to determine if the difference in average height between the two groups is statistically significant.
5. Draw Conclusions
Based on the statistical analysis, determine whether the data supports or refutes the hypothesis. If the difference is statistically significant, the hypothesis is supported. If not, the hypothesis is refuted, or at least requires further investigation and refinement.
A scientific hypothesis is a testable prediction, while a theory is a well-substantiated explanation supported by extensive evidence. Consider the character Sheldon on The Big Bang Theory; the question of whether he displays autistic traits, as explored in does sheldon big bang theory have autism , is a fascinating example. While observations about his behavior might form a hypothesis, a comprehensive understanding of autism spectrum disorder would require a robust scientific theory to explain it fully.
Predictability and Explanation

A scientific hypothesis, much like a mischievous imp, makes a prediction about a specific situation. A theory, on the other hand, is a seasoned sage, offering a broad explanation for a wide range of observations. Think of it this way: the hypothesis is a single, carefully aimed dart, while the theory is a well-aimed volley of darts hitting the same general area, consistently and repeatedly.
The difference in their predictive and powers is, quite frankly, astronomical.Theories, having accumulated substantial evidence and survived rigorous testing, possess a far greater predictive capacity than hypotheses. They don’t just predict isolated events; they provide a framework for predicting a whole class of phenomena. This predictive power isn’t just about guessing the future; it’s about understanding the underlying mechanisms that govern the observed events.
For example, the theory of gravity doesn’t just predict that an apple will fall from a tree; it predicts the rate at which it will fall, the trajectory it will follow, and the effect of various factors like air resistance. A hypothesis, conversely, might only predict the apple’s fall in a specific set of conditions.
Predictive Capabilities of Hypotheses and Theories
Hypotheses make specific predictions under defined conditions. For example, a hypothesis might predict that “increasing the concentration of fertilizer will increase the growth rate of tomato plants.” This prediction is limited to tomato plants and the specific variable of fertilizer concentration. In contrast, a theory like the theory of evolution by natural selection predicts the diversification of life forms over vast spans of time, explaining the emergence of new species and the extinction of others across diverse environments.
It’s a much broader, more encompassing prediction. This difference in scope highlights the fundamental distinction between a hypothesis and a theory.
Power of Theories
Theories provide comprehensive explanations for observed phenomena. They don’t just describe what happens; they explainwhy* it happens. For instance, the germ theory of disease explains why people get sick—it’s not due to bad air or imbalances in the humors, as previously believed, but due to the presence and activity of microscopic organisms. This explanation allows us to develop effective treatments and preventative measures, something a simple observation of sickness alone couldn’t achieve.
A hypothesis, on the other hand, might only identify a correlation between a specific microorganism and a particular illness, without explaining the underlying mechanisms of infection and disease progression.
Illustrative Scenario: Predicting the Weather vs. Explaining Climate Change
Let’s imagine a scenario. A meteorologist predicts rain tomorrow (a hypothesis-like prediction, specific and short-term). This prediction is based on current weather patterns and models. However, explaining why the overall global climate is warming (a theory-based explanation, broad and long-term) requires understanding the greenhouse effect, the role of greenhouse gases, and the impact of human activities. The weather prediction is a short-term, specific prediction; the explanation for climate change is a long-term, broad explanation built upon a vast body of evidence and supported by the overarching theory of climate science.
The former is a single dart; the latter, a full quiver.
Revisions and Refinements
Scientific theories, unlike stubborn mules, are remarkably adaptable. They evolve, morph, and occasionally even undergo complete metamorphoses in the face of new evidence. This dynamic nature is a testament to the self-correcting power of science, a process that involves constant revisions and refinements, ensuring our understanding of the universe remains as accurate as possible – even if that accuracy is sometimes hilariously wrong at first.
The scientific method isn’t a straight line; it’s more like a drunken snail leaving a trail of slightly inaccurate, yet ultimately enlightening, slime. Hypotheses are the tentative stepping stones, often discarded or adjusted as we stumble along. Theories are the more robust pathways, constantly being repaved and widened as our understanding improves. Let’s delve into the fascinatingly messy details.
Hypothesis Modification and Discarding
Scientific hypotheses, those initial hunches about how the world works, are frequently refined or abandoned when confronted with stubborn reality. The process is less dramatic than a scientific revolution and more like a gentle nudge toward a better explanation. Consider these examples:
Hypothesis | Initial Prediction | Contradictory Evidence | Modification/Rejection |
---|---|---|---|
Spontaneous Generation | Life arises spontaneously from non-living matter. | Pasteur’s experiments demonstrating that microorganisms only arise from pre-existing microorganisms. | Rejected. The theory of biogenesis replaced it. |
Phlogiston Theory | Combustion involves the release of a fire-like element called phlogiston. | Lavoisier’s experiments showing that combustion involves the combination of a substance with oxygen, resulting in an increase in mass. | Rejected. The modern understanding of oxidation replaced it. |
Geocentric Model of the Solar System | The Earth is the center of the universe, with all celestial bodies revolving around it. | Kepler’s laws of planetary motion and Galileo’s telescopic observations. | Modified into the heliocentric model, placing the sun at the center. |
Theory Revision in Light of New Discoveries
The iterative nature of theory revision is beautifully illustrated by the atomic theory. Initially, Dalton proposed a simple model of indivisible atoms. Later discoveries, such as the existence of electrons, protons, and neutrons, necessitated significant modifications. The introduction of quantum mechanics further revolutionized our understanding, leading to a probabilistic model of the atom that replaced the earlier deterministic views.
This constant refinement, driven by experimental evidence and theoretical advancements, highlights the dynamic and ever-evolving nature of scientific understanding. The scientific community, through rigorous peer review, acts as a quality control mechanism, ensuring that only the most robust and well-supported theories survive the scrutiny. The discovery of the Higgs boson, for example, provided further evidence supporting and refining the Standard Model of particle physics, a testament to this iterative process.
Timeline of the Germ Theory of Disease
1847: Ignaz Semmelweis observes that handwashing significantly reduces the incidence of puerperal fever in hospitals.
1861: Louis Pasteur publishes his work demonstrating that microorganisms cause fermentation, providing crucial evidence supporting the germ theory.
1876: Robert Koch isolates and identifies
-Bacillus anthracis* as the cause of anthrax, establishing Koch’s postulates, a set of criteria for establishing a causal link between a microorganism and a disease.
1881: Pasteur develops a vaccine against anthrax.
1882: Koch discovers
-Mycobacterium tuberculosis*, the causative agent of tuberculosis.
1884: Koch develops methods for cultivating bacteria in pure cultures, further advancing microbiological research.
1900s-Present: Further advancements in microbiology, immunology, and genetics significantly expand our understanding of infectious diseases and lead to the development of numerous vaccines and antibiotics.
Impact of Paradigm Shifts
Paradigm | Key Figures | Evidence for Shift | Impact on Subsequent Research |
---|---|---|---|
Geocentric Model | Ptolemy | Observations inconsistent with the geocentric model, Kepler’s laws, Galileo’s observations. | Led to the development of Newtonian mechanics and our modern understanding of the solar system and universe. |
Newtonian Physics | Isaac Newton | Observations at high speeds and small scales that contradicted Newtonian predictions, the Michelson-Morley experiment. | Led to the development of Einstein’s theory of relativity and quantum mechanics. |
Approaches to Theory Revision
The revision of scientific theories can be either incremental or revolutionary.
Here’s a comparison:
- Incremental Theory Revision: This involves making small adjustments to an existing theory to account for minor discrepancies. For example, the periodic table has been incrementally revised with the discovery of new elements.
- Revolutionary Theory Revision: This involves a complete overhaul of an existing theory, often due to the emergence of a new paradigm. The shift from Newtonian physics to Einsteinian physics is a prime example.
The Role of Falsifiability in Theory Revision
Falsifiability is the capacity for a theory to be proven wrong. This is crucial because a theory that cannot be disproven is essentially untestable and therefore not scientific. The potential for falsification drives the revision and acceptance of scientific theories. Theories that withstand rigorous attempts at falsification gain credibility, while those that fail are revised or discarded.
Testing the falsifiability of a scientific theory typically involves these steps:
- Formulate a testable hypothesis derived from the theory.
- Design an experiment or observation to test the hypothesis.
- Collect and analyze data from the experiment or observation.
- Evaluate whether the data supports or refutes the hypothesis.
- If the data refutes the hypothesis, revise the theory or discard it.
- If the data supports the hypothesis, further testing is needed to strengthen the theory’s credibility.
The Role of Observation
Observation, my friends, is not merely the passive act of watching paint dry (though thatcan* be surprisingly insightful, depending on the paint). In the scientific realm, observation is the lifeblood of discovery, the intrepid explorer charting the uncharted territories of the universe (or, you know, a particularly interesting petri dish). It’s the foundation upon which hypotheses are built and theories are tested, a crucial step in the grand, slightly chaotic dance of scientific progress.Observations contribute to both the formulation of hypotheses and the development and support of theories in a symbiotic relationship.
A keen eye, coupled with a curious mind, can unearth patterns and anomalies that spark initial questions, leading to testable hypotheses. Further observations, carefully designed and meticulously documented, then provide the crucial evidence to either support or refute these hypotheses, ultimately shaping and refining our scientific theories. Think of it as a feedback loop of intellectual deliciousness – a cycle of questioning, observing, refining, and, occasionally, triumphantly shouting “Eureka!” (though perhaps in a more subdued, scientifically appropriate manner).
Observations in Hypothesis Formulation
Observations often serve as the genesis of scientific inquiry. Notice something unusual? A puzzling phenomenon? That’s your cue to start asking “why?”. For instance, observing that certain plants consistently grow taller near a stream might lead to a hypothesis about the role of water availability in plant growth.
The initial observation provides the raw material, the intriguing puzzle piece that sets the scientific mind whirring. Without the initial observation, the hypothesis would remain dormant, a theoretical seed never sown in the fertile ground of empirical investigation. It’s like trying to bake a cake without flour – not going to happen, folks.
Observations in Theory Development and Support
Observations don’t just start the process; they sustain it. Once a hypothesis is formulated, further, more structured observations are needed to gather data. These observations can be quantitative (e.g., measuring plant height) or qualitative (e.g., describing leaf color), but they must be systematic and repeatable to be scientifically valid. The accumulation of supporting observations over time strengthens the hypothesis, eventually leading to the development of a theory.
Conversely, observations that contradict a hypothesis may lead to its revision or rejection, thus refining our understanding of the natural world. It’s a constant process of refinement, a scientific version of sculpting a magnificent marble statue—chipping away at the excess until the masterpiece emerges.
Examples of Observation-Driven Breakthroughs
The discovery of penicillin, for instance, stemmed from Alexander Fleming’s astute observation of a mold inhibiting bacterial growth. This seemingly insignificant observation revolutionized medicine. Similarly, the observation of planetary motion by astronomers like Tycho Brahe, meticulously recorded over years, provided the data that allowed Johannes Kepler to formulate his laws of planetary motion. These are just two examples of how seemingly mundane observations, when combined with intellectual curiosity and rigorous methodology, can lead to paradigm-shifting scientific breakthroughs.
Sometimes, the most profound discoveries are hidden in plain sight – you just need to know where to look (and, perhaps, have a bit of luck).
Examples from Different Fields
This section delves into the fascinating world of hypothesis testing, showcasing how the scientific method plays out in diverse scientific disciplines. We’ll explore specific, novel hypotheses from biology, physics, and chemistry, detailing their testing methodologies and potential challenges. Buckle up, it’s going to be a wild ride through the exciting landscape of scientific inquiry!
Detailed Hypothesis Examples
Here, we present three original hypotheses, one each from biology, physics, and chemistry, ready to be put to the test. Each hypothesis clearly defines the independent and dependent variables, setting the stage for rigorous experimentation.
- Biology: Hypothesis: Increased exposure to blue light emitted from electronic devices before sleep significantly reduces melatonin production in young adults (aged 18-25), leading to impaired sleep quality. Independent Variable: Duration of blue light exposure before sleep. Dependent Variable: Melatonin levels and sleep quality (measured by polysomnography).
- Physics: Hypothesis: The resonant frequency of a simple pendulum increases linearly with the square root of the gravitational acceleration experienced by the pendulum. Independent Variable: Gravitational acceleration (simulated by tilting the pendulum setup). Dependent Variable: Resonant frequency of the pendulum.
- Chemistry: Hypothesis: The rate of the reaction between a specific concentration of sodium thiosulfate and hydrochloric acid is directly proportional to the square of the concentration of the thiosulfate ion at constant temperature. Independent Variable: Concentration of sodium thiosulfate. Dependent Variable: Reaction rate (measured by the time taken for a fixed amount of sulfur to precipitate).
Hypothesis Testing Methods
Now for the exciting part – how do we actually test these wild hypotheses? Each requires a carefully designed experiment, meticulous data collection, and robust statistical analysis to ensure scientific rigor (and hopefully avoid embarrassing blunders!).
- Biology: Methodology: A randomized controlled trial involving two groups of young adults (18-25). One group is exposed to blue light from electronic devices for a specified duration before sleep, while the control group is exposed to minimal blue light. Melatonin levels are measured using saliva samples, and sleep quality is assessed using polysomnography. Statistical Analysis: t-test to compare melatonin levels and sleep quality between groups.
Confounding variables (e.g., caffeine intake, stress levels) will be controlled through questionnaires and standardized protocols.
- Physics: Methodology: A simple pendulum is constructed, and its resonant frequency is measured at various angles of tilt, simulating changes in gravitational acceleration. The pendulum’s motion is recorded using high-speed video analysis. Statistical Analysis: Linear regression analysis to determine the relationship between gravitational acceleration and resonant frequency. Confounding variables (e.g., air resistance, friction) are minimized through controlled environmental conditions and precise measurements.
- Chemistry: Methodology: Reactions are conducted at constant temperature with varying concentrations of sodium thiosulfate and a fixed concentration of hydrochloric acid. The time taken for a specific amount of sulfur to precipitate is measured. Statistical Analysis: Non-linear regression analysis to determine the order of the reaction with respect to thiosulfate. Confounding variables (e.g., impurities, variations in temperature) are controlled through precise measurements and the use of high-purity chemicals.
Comparative Analysis Table
Let’s organize our findings into a handy table for easy comparison.
Field | Hypothesis | Independent Variable(s) | Dependent Variable(s) | Methodology | Statistical Analysis | Expected Outcome (if hypothesis is true) |
---|---|---|---|---|---|---|
Biology | Increased blue light exposure before sleep reduces melatonin production and impairs sleep quality in young adults. | Duration of blue light exposure | Melatonin levels, sleep quality | Randomized controlled trial, saliva samples, polysomnography | t-test | Significantly lower melatonin levels and poorer sleep quality in the blue light exposure group. |
Physics | The resonant frequency of a simple pendulum increases linearly with the square root of gravitational acceleration. | Gravitational acceleration (simulated by tilting) | Resonant frequency | High-speed video analysis of pendulum motion at varying angles | Linear regression | A positive linear correlation between the square root of gravitational acceleration and resonant frequency. |
Chemistry | The rate of the reaction between sodium thiosulfate and hydrochloric acid is directly proportional to the square of the thiosulfate ion concentration. | Concentration of sodium thiosulfate | Reaction rate (time for sulfur precipitation) | Timed reaction with varying thiosulfate concentrations | Non-linear regression | A second-order reaction with respect to thiosulfate ion concentration. |
Limitations and Challenges
Even the most meticulously planned experiments face hurdles. Let’s address some potential limitations.
- Biology: Individual variations in melatonin production and sleep patterns could affect results. Solution: Increase sample size and account for individual differences using statistical methods.
- Physics: Air resistance and friction can affect the pendulum’s motion. Solution: Conduct experiments in a vacuum or use a very low-friction environment.
- Chemistry: Impurities in chemicals can influence reaction rates. Solution: Use high-purity chemicals and rigorously control environmental conditions.
Illustrative Diagram (Physics)
Imagine a simple pendulum suspended from a fixed point. The pendulum is initially at rest in a vertical position. The setup is then tilted to varying angles, changing the effective gravitational acceleration acting on the pendulum. The pendulum’s oscillations are recorded using a high-speed camera.
Chemical Equation (Chemistry)
The reaction between sodium thiosulfate (Na₂S₂O₃) and hydrochloric acid (HCl) is:
Na₂S₂O₃(aq) + 2HCl(aq) → 2NaCl(aq) + H₂O(l) + S(s) + SO₂(g)
Data Interpretation (Biology)
Let’s say we collected the following hypothetical data on melatonin levels (ng/mL) in our biological experiment:| Group | Melatonin Level (ng/mL) ||—————–|————————|| Control | 10, 12, 11, 9, 10 || Blue Light | 7, 6, 8, 5, 7 |A t-test would compare the means of the two groups.
If the p-value is less than 0.05, we’d reject the null hypothesis (that there’s no difference) and conclude that blue light exposure significantly reduces melatonin levels.
Ethical Considerations (All Fields)
Ethical considerations are paramount. For the biology experiment, informed consent is crucial. In the physics experiment, ensuring safety is key. In the chemistry experiment, proper disposal of chemicals is essential.
The Relationship Between Hypothesis and Theory
The relationship between a scientific hypothesis and a theory is not a simple one-way street; it’s more like a lively, iterative dance, sometimes a tango, sometimes a waltz, occasionally a bit of a chaotic mosh pit. Hypotheses are the stepping stones, sometimes wobbly, sometimes sturdy, that lead to the grander edifice of a scientific theory. This process involves a fascinating interplay of deduction and induction, a constant back-and-forth between specific predictions and broader explanations.
Detailed Description of the Relationship
Hypothesis testing and theory building are inextricably linked in a cyclical process. A hypothesis, a testable prediction about the natural world, is often born from an existing theory or a keen observation. Through rigorous experimentation and data analysis, we test this hypothesis. The outcome—whether the hypothesis is supported or refuted—directly impacts the theory. Confirmation provides evidence bolstering the theory; falsification may lead to its refinement, modification, or even rejection.
Inductive reasoning, moving from specific observations to broader generalizations, plays a crucial role in forming hypotheses and theories. Deductive reasoning, moving from general principles (theories) to specific predictions (hypotheses), is vital in designing tests to evaluate these hypotheses. This continuous feedback loop is what drives scientific progress.A successful hypothesis, characterized by testability, falsifiability, and parsimony (simplicity), provides a building block for a theory.
Multiple successful hypotheses supporting a common underlying concept strengthen the theory’s foundation. For example, numerous experiments demonstrating the existence of gravitational force contributed to the development of Newton’s Law of Universal Gravitation. Conversely, an unsuccessful hypothesis, while seemingly a setback, provides invaluable information. It highlights flaws in existing theories, prompts researchers to re-evaluate assumptions, and guides the formulation of new, improved hypotheses.
This iterative process of hypothesis refinement, guided by both positive and negative results, ultimately leads to a more robust and accurate theory. The theory of plate tectonics, for example, emerged from the accumulation of geological observations and the testing of numerous hypotheses about continental drift.
Illustrative Flowchart
The iterative process of hypothesis testing and theory building can be visualized as follows:
1. Observation and Question
A scientist notices a peculiar phenomenon (e.g., the consistent direction of bird migration). This sparks a research question (e.g., What factors influence bird migration patterns?).
2. Hypothesis Formulation
A testable hypothesis is formulated (e.g., Birds migrate south in winter due to decreasing temperatures and reduced food availability).
3. Research Design and Data Collection
A research plan is developed, including methods for data collection (e.g., tracking bird movements using GPS tags, measuring temperature and food abundance).
4. Hypothesis Testing and Analysis
Data is collected and analyzed using appropriate statistical methods (e.g., correlation analysis, regression analysis). A decision is made to accept or reject the hypothesis based on the statistical significance of the results.
5. Interpretation of Results
The results are interpreted in the context of existing theories (e.g., the results support the hypothesis, lending further evidence to theories about animal behavior and environmental adaptation).
6. Theory Refinement or Development
The findings either refine existing theories or contribute to the development of new ones. In our example, the results might strengthen existing theories about the role of environmental factors in animal migration.
7. Generation of New Hypotheses
The refined theory leads to the generation of new, testable hypotheses (e.g., Does the intensity of magnetic fields influence bird migration routes?). The cycle continues.
Comparative Table
The following table contrasts the characteristics of hypotheses and theories:
Feature | Hypothesis Description | Theory Description |
---|---|---|
Scope | Specific, focused prediction | Broad explanation encompassing many observations |
Testability | Directly testable through experimentation | Tested indirectly through the testing of numerous hypotheses |
Tentativeness | Can be supported or refuted | Well-supported, but still subject to revision |
Level of Support | Based on limited evidence from a single study | Based on a large body of evidence from multiple studies |
Example Analysis
The discovery of the structure of DNA provides a classic example. Linus Pauling initially proposed a triple-helix structure for DNA. However, Watson and Crick’s hypothesis, based on X-ray diffraction data and Chargaff’s rules, proposed a double-helix structure. Experimental evidence overwhelmingly supported the Watson-Crick model, leading to the refinement of our understanding of genetics and the development of the central dogma of molecular biology. This highlights how a successful hypothesis (Watson-Crick model) contributed to the development of a robust theory (molecular biology). Pauling’s unsuccessful hypothesis, though incorrect, still spurred further research and contributed to the eventual acceptance of the correct model.
Limitations and Considerations, How does a scientific theory differ from a scientific hypothesis
While hypothesis testing is a cornerstone of scientific progress, it has limitations. Confirmation bias, the tendency to favor evidence supporting pre-existing beliefs, can skew interpretations. Serendipity, unexpected discoveries, also plays a significant role in scientific breakthroughs. Furthermore, dealing with complex phenomena often requires tackling multiple interacting variables, making it challenging to isolate cause-and-effect relationships. These limitations emphasize the importance of rigorous methodology, critical evaluation, and a healthy dose of scientific skepticism in the pursuit of knowledge.
Misconceptions about Theories
Ah, scientific theories! They sound so…theoretical. Like something dreamt up by a caffeine-fueled professor in a dimly lit lab. But the truth is far more exciting (and less prone to spontaneous combustion). Let’s debunk some common misunderstandings surrounding these pillars of scientific understanding.The public often conflates “theory” with “guess,” “hypothesis,” or even “opinion.” This leads to a frustrating disconnect between how scientists use the term and how it’s perceived by the general public.
This misconception stems from a fundamental misunderstanding of the rigorous process behind the development and validation of scientific theories. It’s time to set the record straight.
The Nature of Scientific Certainty and Uncertainty
Scientific certainty isn’t about absolute, unwavering truth, like the existence of gravity (although even that’s constantly being refined!). Instead, it’s a matter of degree. Theories are supported by overwhelming evidence, rigorously tested, and constantly evaluated. Think of it like a pyramid built of meticulously examined bricks – each new piece of evidence adds to the structure’s stability. However, this doesn’t mean the pyramid is immutable.
New discoveries can lead to revisions or refinements, but this doesn’t invalidate the core structure. Uncertainty, therefore, isn’t a weakness, but an inherent part of the scientific process. It acknowledges that our understanding is always evolving and subject to further investigation. For example, our understanding of the universe’s expansion has been refined multiple times as new data becomes available from telescopes like Hubble and JWST.
This continuous refinement is a strength, not a flaw.
Improving Public Understanding of Scientific Theories
One approach to improving public understanding is to emphasize the process, not just the outcome. Instead of simply stating a theory (e.g., the theory of evolution), we should highlight the extensive body of evidence supporting it, including fossil records, genetic analysis, and observed speciation. Another approach is to use relatable analogies. For example, comparing the refinement of a scientific theory to the iterative process of software development: initial versions are released, bugs are found and fixed, and new features are added based on user feedback and improved understanding.
This emphasizes the dynamic and evolving nature of scientific knowledge, making it less intimidating and more accessible. Finally, clear, accessible communication from scientists is crucial. Explaining complex concepts in simple terms, using visual aids, and engaging in open dialogue with the public can bridge the gap between scientific understanding and public perception. Imagine explaining the intricacies of quantum physics using LEGO bricks! It might just work.
The Importance of Peer Review
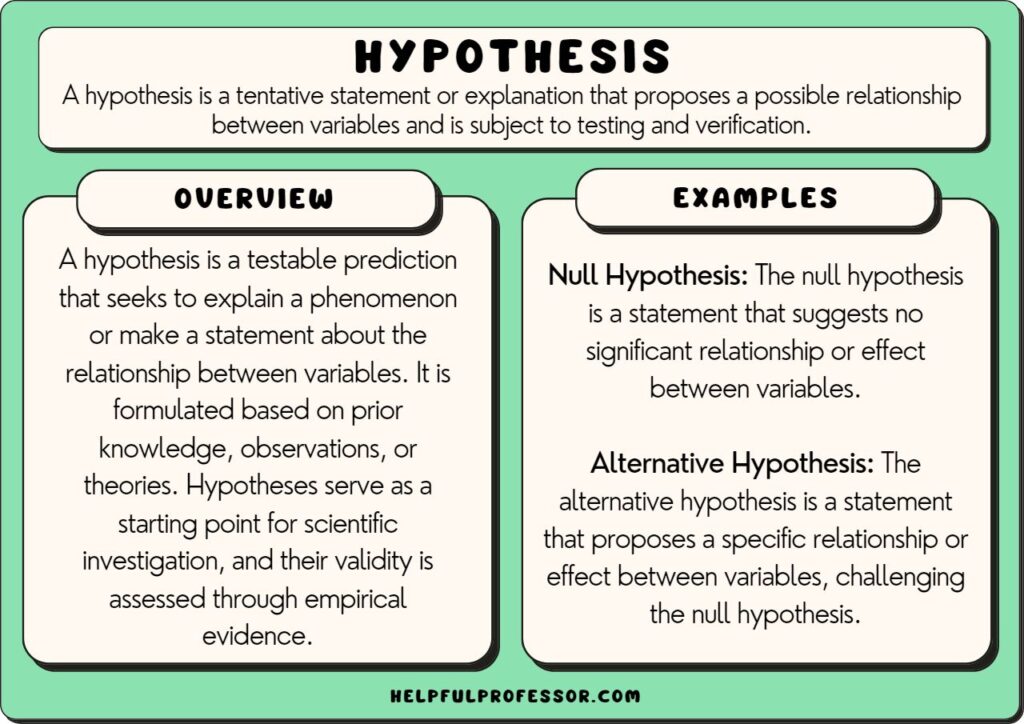
Peer review, the process by which scientific work is subjected to scrutiny by experts in the field before publication, is not merely a gatekeeping mechanism; it’s the linchpin of scientific progress, a vital safeguard against erroneous conclusions, and a critical contributor to the reliability and validity of scientific knowledge. Without this rigorous process, the scientific landscape would be a chaotic jumble of unsubstantiated claims and poorly designed experiments, a veritable Wild West of scientific inquiry.
Let’s delve into the multifaceted role of peer review in ensuring the integrity and advancement of science.
Hypothesis Evaluation Through Peer Review
Peer review plays a crucial role in refining and validating scientific hypotheses. Reviewers meticulously examine the clarity, testability, and underlying assumptions of a hypothesis, often identifying logical fallacies, methodological weaknesses, or unacknowledged biases that could compromise its validity. For example, a hypothesis stating “Eating chocolate improves mood” is far too vague. Peer review would likely demand a more precise formulation, specifying the type of chocolate, the quantity consumed, the methods of mood assessment, and the target population.
The table below illustrates this refinement process.
Feature | Poorly Formulated Hypothesis | Refined Hypothesis After Peer Review |
---|---|---|
Clarity | Eating chocolate improves mood. | Consuming 50 grams of dark chocolate (70% cacao) daily for four weeks improves self-reported mood scores (using the Profile of Mood States questionnaire) in adult women aged 25-40 compared to a control group consuming an equivalent amount of sugar-free placebo. |
Testability | Difficult to test objectively; subjective mood assessment. | Easily testable using a controlled experiment with quantitative mood measures and a control group. |
Assumptions | Unstated assumptions about the type of chocolate, mood measurement, and participant characteristics. | Clearly stated assumptions about the specific type of chocolate, the use of a validated mood scale, and the inclusion criteria for participants. |
Potential Biases | Potential for researcher bias in mood assessment. | Blind assessment of mood scores by independent researchers to minimize bias. |
Theory Evaluation Through Peer Review
Peer review also serves as a critical evaluation mechanism for scientific theories. Reviewers assess a theory’s coherence, power, and predictive accuracy, examining the evidence supporting the theory and identifying potential gaps or inconsistencies. Theories are not static entities; they evolve and are refined through rigorous peer review. For instance, the theory of continental drift, initially met with skepticism, was significantly strengthened and eventually accepted as plate tectonics after decades of peer review and the accumulation of supporting evidence from various fields, such as geophysics and paleontology.
Conversely, theories lacking robust evidence or facing insurmountable inconsistencies are often rejected or significantly revised.
Peer Review’s Enhancement of Reliability and Validity
Peer review significantly enhances the reliability and validity of scientific findings. Reviewers scrutinize the research methodology, data analysis, and interpretation of results, identifying potential sources of error, inconsistencies, and methodological flaws. The demand for replication studies, often prompted by peer review comments, further bolsters the reliability of findings. By ensuring the appropriateness of research methods and the accuracy of interpretations, peer review enhances the validity of scientific conclusions, minimizing the impact of potential biases that could compromise the integrity of the research.
The Peer-Review Process and Scientific Integrity
The peer-review process typically involves several steps: submission of the manuscript, assignment to appropriate reviewers, review by the reviewers (who often remain anonymous), an editorial decision based on the reviews, and potential revisions based on reviewer feedback. This process, while not foolproof, serves as a crucial safeguard against scientific misconduct, such as plagiarism and data fabrication. The anonymity of reviewers can both protect them from potential retribution and allow for unbiased evaluation, although it can also hinder the process if reviewers lack sufficient transparency or accountability.
However, inherent biases within the peer-review system, such as publication bias favoring positive results, remain a concern. Strategies to mitigate these biases include employing diverse review panels, implementing standardized review criteria, and employing open peer review models.
- Manuscript Submission
- Assignment to Reviewers
- Reviewer Evaluation
- Editor Decision
- Revision (if necessary)
- Publication
Peer review is a cornerstone of scientific integrity, demanding honesty, objectivity, and a commitment to rigorous evaluation from both authors and reviewers. Reviewers have a responsibility to provide constructive criticism, identify potential flaws, and ensure the accuracy and validity of published research. Authors, in turn, must be transparent in their methods and data, and receptive to constructive feedback.
Limitations of Peer Review
Despite its importance, peer review is not without limitations. The system can be susceptible to biases, particularly in highly specialized fields where finding truly independent and unbiased reviewers can be challenging. Furthermore, even the most rigorous peer review process cannot guarantee the complete absence of errors or the detection of subtle flaws. The time constraints and workload of reviewers can also impact the thoroughness of the review process.
The Evolution of Scientific Understanding
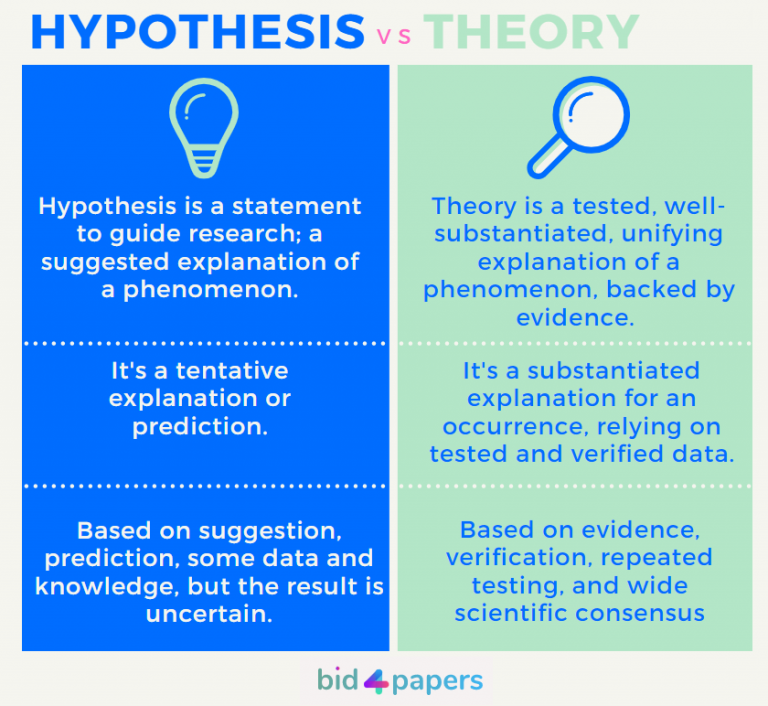
Scientific understanding isn’t a static monument; it’s more like a thrilling rollercoaster ride through the landscape of knowledge, with plenty of twists, turns, and the occasional stomach-churning drop. The interplay between hypotheses and theories drives this exhilarating journey, constantly refining our comprehension of the universe and everything in it (well, almost everything). This process, while sometimes messy and unpredictable, is what allows science to progress and achieve ever-greater accuracy in its descriptions of reality.The evolution of scientific understanding is a dynamic process fueled by the constant testing and refinement of both hypotheses and theories.
Hypotheses, those tentative explanations for specific observations, act as the building blocks. When numerous experiments and observations consistently support a hypothesis, it may develop into a theory – a more comprehensive and robust explanation supported by a wide body of evidence. However, even theories are not immune to change; as new evidence emerges or experimental techniques improve, theories can be revised, refined, or even replaced entirely.
This isn’t a sign of failure, but rather a testament to the self-correcting nature of the scientific method.
Revised and Replaced Theories
Several prominent scientific theories have undergone significant revisions or have been superseded by more comprehensive models. For instance, the geocentric model of the universe, which placed the Earth at the center, was eventually replaced by the heliocentric model, placing the Sun at the center. This shift, driven by meticulous observations and mathematical modeling, revolutionized astronomy. Similarly, Newtonian physics, incredibly successful for centuries in explaining many aspects of motion and gravity, was later refined and extended by Einstein’s theory of relativity, which better accounts for phenomena at very high speeds and strong gravitational fields.
Consider the evolution of atomic theory: from Dalton’s simple model of indivisible atoms to the complex quantum mechanical model that describes atoms as systems of interacting subatomic particles. Each revision built upon previous understanding, correcting limitations and incorporating new discoveries. The story of scientific progress is filled with such examples of refinement and replacement, demonstrating the dynamic and iterative nature of scientific knowledge.
The Dynamic Nature of Scientific Knowledge
Scientific knowledge is not a collection of immutable truths, but rather a constantly evolving body of understanding. It’s a process of continuous refinement, with new data and insights constantly challenging and reshaping existing theories. The refinement of scientific understanding is an iterative process: a theory is proposed, tested, refined, and potentially replaced by a better theory as new evidence accumulates.
This continuous process is essential for the advancement of science, ensuring that our understanding of the world remains accurate and comprehensive. The inherent uncertainty in science is not a weakness, but rather a crucial element of its strength, allowing for continuous progress and improvement. This dynamic nature is what makes science such a powerful tool for understanding the world.
Detailed FAQs
What if a hypothesis is proven wrong?
That’s totally normal! A refuted hypothesis doesn’t mean the end of the research. It just means you’ve learned something valuable and can refine your approach or explore alternative explanations.
Can a theory ever be proven absolutely true?
Nope. Scientific theories are always open to revision based on new evidence. The strength of a theory lies in its power and the robustness of the evidence supporting it, not in absolute certainty.
How long does it take for a hypothesis to become a theory?
There’s no set timeline. Some hypotheses quickly lead to robust theories, while others take decades or even centuries of research to fully develop.
What’s the difference between a scientific theory and a everyday theory?
A scientific theory is backed by rigorous testing and evidence. An everyday theory is just a guess or speculation.