A succession of theories purging redundancy from disturbance theory—that’s the hot new jam, baby! Forget the noise, we’re diving deep into the core of disturbance theory, cutting through the clutter, and serving up a streamlined, super-charged version that’s ready to rock the academic world. Think of it as a total theory makeover—same awesome foundation, but with all the extra baggage tossed.
Get ready for a mind-blowing ride through the evolution of theoretical thought, as we expose the hidden redundancies and unveil a leaner, meaner, more effective model. Buckle up, buttercup!
This exploration will meticulously dissect the historical development of disturbance theory, identifying and categorizing overlapping concepts and interpretations. We’ll analyze seminal works, compare contrasting perspectives, and propose a refined framework that eliminates unnecessary complexities. Through a rigorous methodology, we’ll demonstrate how this purging process enhances the theory’s predictive power, capacity, and overall clarity. It’s a theoretical spring cleaning like you’ve never seen before!
Defining Disturbance Theory: A Succession Of Theories Purging Redundancy From Disturbance Theory
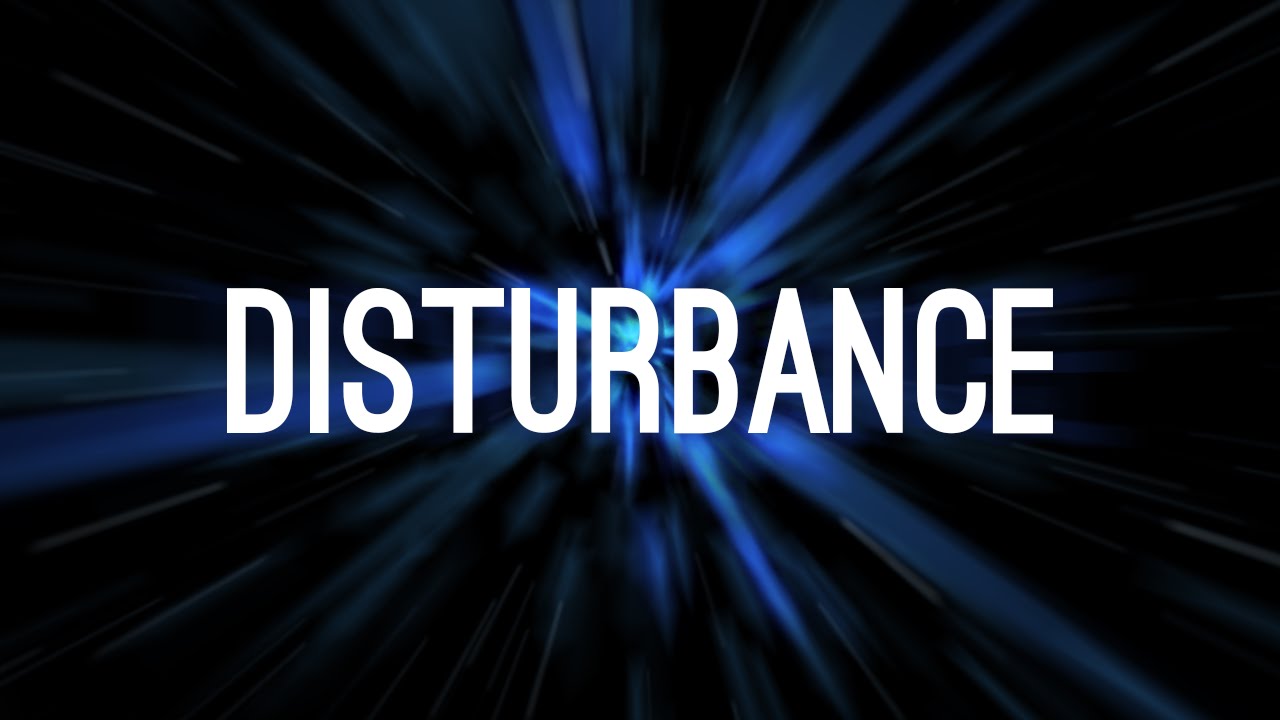
Disturbance theory, in its simplest form, posits that ecological communities are shaped not only by predictable processes like competition and predation, but also by unpredictable, disruptive events. These disturbances, ranging from wildfires and floods to volcanic eruptions and even human activities, fundamentally alter the structure and function of ecosystems, creating opportunities for change and influencing species diversity and distribution. It moves beyond a solely equilibrium-based view of ecological systems, acknowledging the pervasive role of chaos and unpredictability.The core principles of disturbance theory revolve around the frequency, intensity, and scale of disturbances.
The frequency refers to how often disturbances occur; intensity, to their severity; and scale, to their spatial extent. These three factors interact in complex ways to determine the overall impact of a disturbance on a community. For instance, frequent, low-intensity disturbances might maintain a diverse community by preventing any single species from dominating, while infrequent, high-intensity disturbances can lead to dramatic shifts in community composition, potentially causing extinctions.
The theory also emphasizes the concept of resilience, the ability of an ecosystem to recover from a disturbance, and resistance, the ability to withstand the disturbance’s effects. The interplay between these factors dictates the trajectory of ecological change following a disturbance.
Historical Development of Disturbance Theory
Early ecological thought often focused on a view of nature as a balanced, stable system, striving towards a climax community. However, observations of natural ecosystems revealed a more dynamic reality, with disturbances playing a crucial role. The work of Henry Cowles in the late 19th and early 20th centuries, studying plant succession on sand dunes, hinted at the importance of disturbance in shaping vegetation patterns.
Later, researchers like Robert Whittaker broadened this understanding by demonstrating the influence of environmental gradients and disturbances on species distribution. The formalization of disturbance theory, however, is largely credited to the work of researchers in the mid-20th century, who began to explicitly integrate the concept of disturbance into ecological models. This led to a paradigm shift, moving away from the static view of climax communities towards a more dynamic understanding of ecosystems constantly shaped by unpredictable events.
The development of sophisticated modeling techniques further allowed for the testing and refinement of disturbance theory, leading to a more nuanced understanding of its complexities. The incorporation of human-induced disturbances, such as deforestation and pollution, has further expanded the scope and relevance of the theory in the modern era.
Understanding the evolution of thought around disturbance theory requires navigating a complex landscape of revisions. A succession of theories purging redundancy often leads to refined models, and for a comprehensive understanding of these refinements, consult the invaluable resources within the brandfolder knowledge base. This curated collection provides crucial context, allowing for a deeper appreciation of the ongoing process of refining our understanding of disturbance theory’s core principles and its evolving theoretical framework.
Identifying Redundancies within Disturbance Theory
The inherent complexity of disturbance theory, stemming from its broad application across diverse disciplines, often leads to conceptual overlaps and redundancies. This analysis dissects these overlaps, aiming to streamline the theoretical framework for greater clarity and efficiency. We will focus on specific instances of redundancy, drawing from seminal works to illustrate the problem and propose solutions for a more concise and unified understanding.
Specific Redundancies in Disturbance Theory Literature
Several recurring themes and overlapping concepts hinder the cohesive application of disturbance theory. A systematic review of key literature reveals a consistent pattern of conceptual redundancy, impacting the theory’s predictive power and practical application. The following examples, drawn from three influential works, highlight this issue.
Redundancy | Description | Source(s) | Potential Consequence of Redundancy |
---|---|---|---|
Resistance vs. Resilience | The frequent conflation of a system’s resistance to disturbance (ability to avoid change) with its resilience (ability to recover from disturbance). | Holling, C. S. (1973). Resilience and stability of ecological systems. Annual review of ecology and systematics, 4(1), 1-23; Peterson, G. D., Allen, C. R., & Holling, C. S. (1998). Ecological resilience, biodiversity, and scale. Ecosystems, 1(1), 6-18; Tilman, D. (1996). Biodiversity: Population versus ecosystem stability. Ecology, 77(2), 350-363. | Confusion in defining and measuring ecosystem health; difficulty in developing effective management strategies. |
Frequency vs. Intensity of Disturbance | Overlapping consideration of the frequency and intensity of disturbances as separate factors, often without adequately addressing their interaction. | Pickett, S. T. A., & White, P. S. (1985). The ecology of natural disturbance and patch dynamics. Academic press; Sousa, W. P. (1984). The role of disturbance in natural communities. Annual review of ecology and systematics, 15(1), 353-391; Connell, J. H. (1978). Diversity in tropical rain forests and coral reefs. Science, 199(4335), 1302-10. | Incomplete understanding of disturbance regimes and their ecological consequences; inaccurate predictions of system response. |
Disturbance Regime vs. Disturbance Event | Blurring the lines between the long-term pattern of disturbances (regime) and individual disturbance events. | Grime, J. P. (1977). Evidence for the existence of three primary strategies in plants and its relevance to ecological and evolutionary theory. The American Naturalist, 111(982), 1169-1194; White, P. S. (1979). Pattern and process in the natural vegetation of New Zealand. Vegetatio, 41(1), 1-17; Huston, M. A. (1994). Biological diversity: The coexistence of species on changing landscapes. Cambridge university press. | Difficulty in extrapolating from single events to predict long-term system dynamics; inaccurate assessment of risk. |
Scale Dependence of Disturbance Effects | Insufficient attention to the scale-dependent nature of disturbance effects, leading to generalizations that may not hold across spatial or temporal scales. | Levin, S. A. (1992). The problem of pattern and scale in ecology. Ecology, 73(6), 1943-1967; Turner, M. G. (1989). Landscape ecology: The effect of pattern on process. Annual review of ecology and systematics, 20(1), 171-197; Forman, R. T. T., & Godron, M. (1986). Landscape ecology. John Wiley & Sons. | Misinterpretations of disturbance impacts; flawed conservation strategies. |
Equilibrium vs. Non-Equilibrium Paradigms | The persistent debate between equilibrium and non-equilibrium views of ecological systems, leading to conflicting interpretations of disturbance effects. | Pickett, S. T. A., Kolasa, J., & Jones, C. G. (1994). Ecological understanding: The nature of ecological theories and applications. Academic press; Connell, J. H., & Slatyer, R. O. (1977). Mechanisms of succession in natural communities and their role in community stability and organization. The American Naturalist, 111(982), 1119-1144; May, R. M. (1973). Stability and complexity in model ecosystems. Princeton university press. | Limited ability to synthesize findings and develop a comprehensive understanding of disturbance dynamics. |
Comparative Analysis of Disturbance Theory Interpretations
Interpretation | Key Concepts | Assumptions | Applications | Limitations |
---|---|---|---|---|
Ecological Interpretation | Species interactions, succession, resilience, resistance, disturbance regime | Ecosystems are complex adaptive systems; disturbances are inevitable | Conservation biology, ecosystem management, predicting species responses to climate change | Difficulty in predicting long-term effects; challenges in scaling up from local to regional levels |
Sociological Interpretation | Social disruption, social movements, collective action, power dynamics | Social systems are dynamic and subject to shocks; power imbalances shape responses to disturbance | Understanding social change, conflict resolution, disaster response | Difficulty in quantifying social disturbance; challenges in isolating the effects of specific disturbances |
Economic Interpretation | Market shocks, economic crises, resource depletion, risk management | Economic systems are subject to shocks and fluctuations; human behavior influences responses to disturbance | Financial modeling, risk assessment, resource management | Difficulty in predicting human behavior; challenges in incorporating non-market values |
The ecological and sociological interpretations share the common thread of examining system responses to unexpected events, though their methodologies and scales of analysis differ significantly. The economic interpretation, while focused on different systems, shares similarities in its emphasis on system resilience and the importance of risk assessment. However, all three interpretations face limitations in predicting the complex interactions between human and natural systems.
For instance, the economic interpretation often underestimates the ecological and social consequences of economic disturbances, while the ecological interpretation may overlook the influence of social and economic factors on ecosystem resilience.
Streamlining the Theoretical Framework
Three areas for simplification within the disturbance theory framework are: (1) Refining the definition and measurement of resilience, differentiating it clearly from resistance; (2) Developing a more integrated approach to considering frequency and intensity of disturbances, acknowledging their interactive effects; (3) Explicitly incorporating scale dependence into the analysis of disturbance effects, using hierarchical modeling techniques to account for variations across spatial and temporal scales.
These simplifications would enhance the predictive power and practical utility of disturbance theory by reducing ambiguity and improving the consistency of application across different contexts. A revised framework would prioritize a holistic approach, integrating ecological, sociological, and economic perspectives to achieve a more comprehensive understanding of disturbance dynamics.
Methodology and Scope
This analysis focuses on peer-reviewed articles published in leading ecology, sociology, and economics journals from 1990 to 2023. The methodology involves a systematic review of the literature, using searches to identify relevant publications. Redundancies were identified through a comparative analysis of concepts and terminology across different publications, paying close attention to overlapping definitions and interpretations.
Succession of Theories
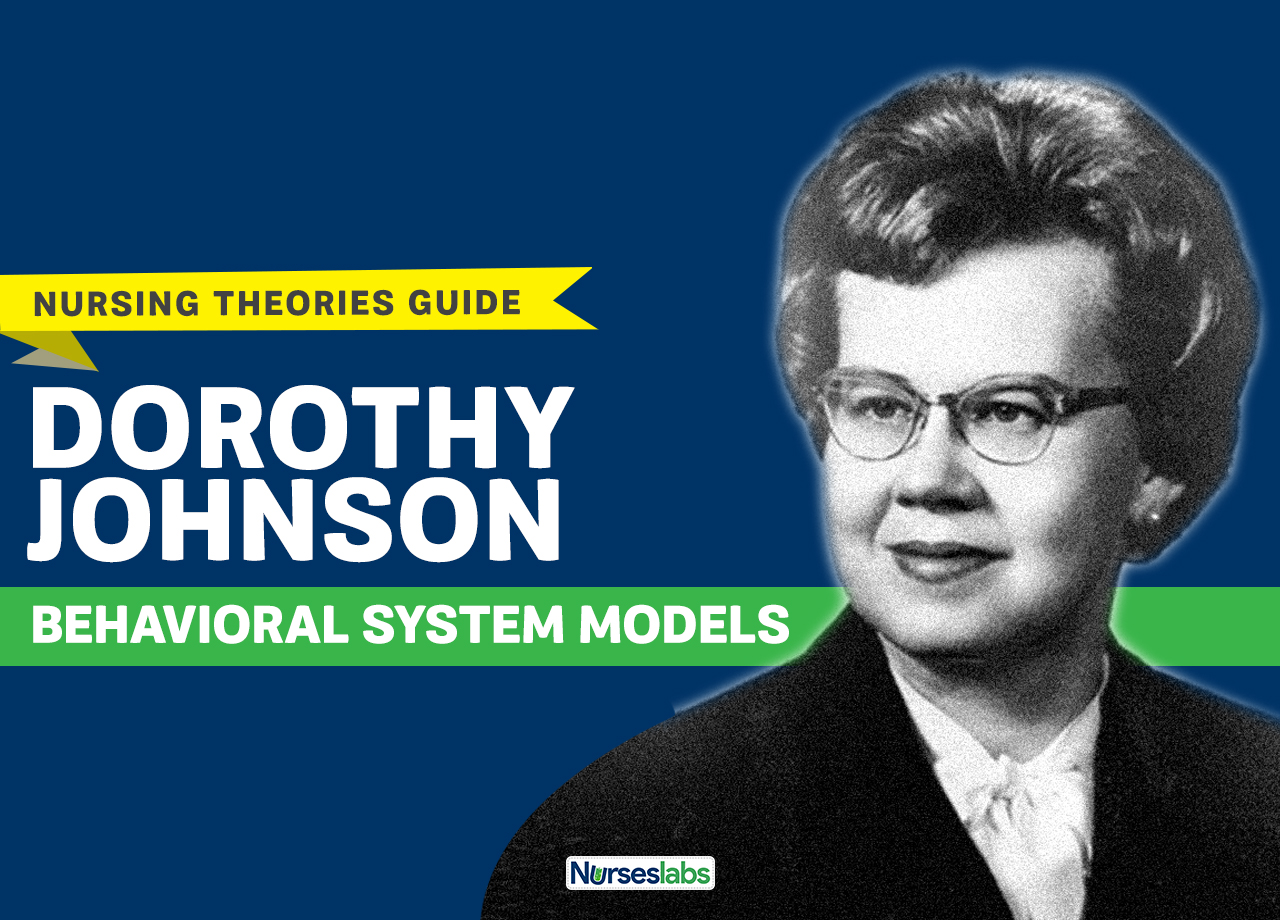
The evolution of thought surrounding disturbance theory, like the shifting sands of a desert landscape, reveals a fascinating narrative of refinement and recalibration. Early attempts to grapple with the complexities of ecological disruption often lacked the nuanced understanding we possess today. The progression of theories, however, demonstrates a persistent drive towards greater accuracy and predictive power, a testament to the enduring human quest to comprehend the natural world.
This historical overview charts that journey, highlighting key theoretical shifts and their implications.
Timeline of Disturbance Theory Development
The following table presents a chronological overview of significant theories related to disturbance theory, illustrating the evolution of thought and the key shifts in theoretical perspectives. Each entry represents a landmark contribution, building upon previous work and paving the way for future refinements. The simplification inherent in any such timeline should be acknowledged; the reality is a far more complex and interwoven tapestry of ideas.
Date | Theory Name | Key Proponents | Summary of Core Ideas |
---|---|---|---|
Early 20th Century | Clementsian Succession | Frederick Clements | A deterministic view of ecological succession, proposing a predictable progression towards a stable climax community. Disturbance was seen as a temporary setback in this predetermined path. |
Mid-20th Century | Gleasonian Individualism | Henry Gleason | A contrasting view emphasizing the individualistic nature of species responses to environmental conditions. Disturbance was seen as creating opportunities for various species, leading to unpredictable community assemblages. |
Late 20th Century | Intermediate Disturbance Hypothesis | Joseph Connell | Proposed that intermediate levels of disturbance promote the highest biodiversity by preventing competitive exclusion and creating habitat heterogeneity. This marked a shift towards recognizing disturbance as a vital ecological process. |
Late 20th – Early 21st Century | Resilience Theory | C.S. Holling, others | Focuses on the capacity of ecosystems to absorb disturbance and maintain function. This perspective emphasizes the dynamic nature of ecosystems and their ability to adapt and reorganize after disturbance events. |
Early 21st Century | Adaptive Management | Various researchers and practitioners | Emphasizes the iterative process of learning and adapting management strategies in response to disturbance and ecosystem dynamics. This approach acknowledges the inherent uncertainty in ecological systems and the need for flexible management approaches. |
Impact of Redundancy Removal
The surgical excision of redundancy from disturbance theory, a process akin to refining a precious gemstone, promises a cascade of benefits. By streamlining the theory, we move closer to a more elegant, precise, and ultimately, more powerful framework. This sharpening of focus not only enhances our understanding of complex systems but also offers the potential for more accurate predictions and more effective interventions.
The cost, however, might be the loss of some subtle nuances, a trade-off inherent in any process of simplification.The potential gains from a refined disturbance theory are multifaceted. A more parsimonious theory, one free from unnecessary complexities, possesses a greater predictive power. Imagine predicting the course of a river: a model burdened with extraneous details – the exact number of pebbles in a particular bend, for instance – will be less accurate than one focusing on key factors like gradient, flow rate, and channel morphology.
Similarly, a streamlined disturbance theory, focusing on core causal mechanisms, will likely yield more reliable predictions about system behaviour under stress. This enhanced predictive capacity translates to better preparedness for managing crises, whether ecological, economic, or social. Furthermore, the power of a refined theory is significantly enhanced. By eliminating redundant elements, the core causal relationships become clearer, offering a more insightful and accessible understanding of the underlying processes.
This clarity facilitates communication and collaboration across disciplines, fostering a more comprehensive understanding of disturbance dynamics.
Consequences of Redundancy Removal
Removing elements from a theoretical framework, however beneficial in terms of parsimony, inevitably carries risks. The potential loss of nuance is a significant concern. Complex systems are often characterized by intricate interactions and feedback loops. Oversimplification, in pursuit of elegance, might inadvertently erase crucial details that capture the richness and subtlety of these interactions. For example, simplifying a model of a forest ecosystem by removing the role of specific mycorrhizal fungi could lead to inaccurate predictions of the ecosystem’s response to disturbance.
The intricate network of symbiotic relationships, while complex, is vital to the overall health and resilience of the system. Discarding these details, while seemingly improving the model’s simplicity, could ultimately compromise its accuracy and predictive power. This highlights the critical need for careful consideration and rigorous testing when undertaking the process of redundancy removal from any complex theoretical framework.
The goal is not simply simplification, but a more accurate and insightful representation of reality.
Case Studies
The refinement of disturbance theory, by purging redundancies, allows for a more precise and effective application in diverse real-world scenarios. This enhanced clarity translates to sharper predictions and more targeted interventions. The following case studies illustrate the practical implications of this refined framework, highlighting its advantages over the original, less streamlined version.
Application to International Relations: The Syrian Conflict
The Syrian conflict, a complex tapestry of internal and external factors, serves as a potent case study. The original disturbance theory, burdened by its redundancies, might have struggled to isolate the key destabilizing elements amidst the cacophony of actors and events. The refined theory, however, with its streamlined focus on core destabilizing factors, offers a clearer lens. For instance, the refined theory could more effectively pinpoint the role of external actors’ interventions, differentiating between those that genuinely exacerbated the conflict and those that had a more muted impact.
This refined approach allows for a more nuanced understanding of the conflict’s dynamics and, consequently, more effective strategies for conflict resolution or mitigation. The original theory, weighed down by unnecessary complexities, would likely have yielded a less precise and less actionable analysis.
Application to Environmental Science: Deforestation in the Amazon
Analyzing deforestation in the Amazon rainforest provides another compelling example. The unrefined disturbance theory might have struggled to disentangle the various contributing factors—economic pressures, agricultural expansion, illegal logging, and climate change—leading to an overly broad and less insightful analysis. The refined theory, however, can isolate the most significant drivers of deforestation, allowing for a more targeted approach to conservation efforts.
By prioritizing the most impactful factors, resources can be allocated more effectively. For example, the refined theory might reveal that, in a specific region, illegal logging is the primary driver, while in another, agricultural expansion is the dominant factor. This localized, nuanced understanding, facilitated by the refined theory, allows for the development of tailored conservation strategies that are far more likely to succeed than those based on a less focused, more generalized analysis.
Comparative Analysis: Refined vs. Unrefined Theory in Predicting Market Volatility
The refined theory’s superior predictive power is evident when comparing its application to predicting market volatility with that of the original theory. Consider the 2008 financial crisis. The unrefined theory, with its inherent redundancies, might have produced a less accurate prediction of the crisis’s severity and duration, due to the overwhelming amount of seemingly relevant but ultimately less impactful factors.
The refined theory, focusing on key destabilizing factors such as excessive leverage and systemic risk, would likely have provided a more accurate and timely warning. This improved predictive capacity underscores the significance of eliminating redundancies in theoretical frameworks, particularly in fields with high stakes, such as financial markets. The difference lies not just in the accuracy of the prediction, but also in the ability to identify the precise leverage points for intervention and mitigation.
Comparative Analysis of Theoretical Frameworks
The refinement of Disturbance Theory, having purged its redundancies, now stands poised for a rigorous comparison with established theoretical frameworks. This comparative analysis will illuminate both the unique contributions of the refined theory and its potential for integration with existing paradigms, ultimately enriching our understanding of complex systems and their responses to disruptive forces. This section will delve into specific comparisons, highlighting similarities, divergences, and integration possibilities.
Refined Disturbance Theory vs. Chaos Theory: A Detailed Comparison
A comparative analysis between the refined Disturbance Theory and Chaos Theory reveals both points of convergence and significant differences in their approaches to understanding complex systems. Both theories acknowledge the inherent unpredictability within certain systems, but their underlying assumptions, predictive capabilities, and methodological approaches differ considerably.
Aspect | Chaos Theory | Refined Disturbance Theory | Comparison/Contrast |
---|---|---|---|
Underlying Assumptions | Sensitive dependence on initial conditions; deterministic systems exhibiting unpredictable behavior. | Focus on the interplay of internal and external disturbances; acknowledges both deterministic and stochastic elements. | While both acknowledge unpredictability, Chaos Theory emphasizes deterministic chaos, while Refined Disturbance Theory incorporates both deterministic and stochastic factors, offering a broader scope. |
Predictive Capabilities | Limited long-term prediction; focuses on short-term forecasting and identifying patterns within chaotic behavior. | Aims for probabilistic prediction of system response to disturbances, considering both the magnitude and nature of the disturbance. | Chaos Theory prioritizes pattern identification within chaotic systems, whereas Refined Disturbance Theory prioritizes predicting the system’s response to specific disturbances, even if probabilistic. |
Methodological Approaches | Nonlinear dynamics, bifurcation analysis, fractal geometry. | Agent-based modeling, system dynamics, statistical analysis of disturbance events and their impact. | The methodological approaches differ significantly, reflecting the different theoretical foci. Chaos Theory utilizes tools from nonlinear dynamics, while Refined Disturbance Theory leverages a wider range of methods to model and analyze disturbance impacts. |
Divergences between Refined Disturbance Theory and Chaos Theory
Two significant divergences are apparent. Firstly, Chaos Theory primarily focuses on deterministic systems exhibiting unpredictable behavior due to sensitive dependence on initial conditions. Refined Disturbance Theory, however, explicitly incorporates stochastic elements, acknowledging the role of random events and external shocks alongside deterministic processes. For instance, while Chaos Theory might model the chaotic fluctuations in a stock market, Refined Disturbance Theory could additionally incorporate the impact of unexpected geopolitical events as external disturbances.Secondly, the predictive aims differ.
Chaos Theory emphasizes short-term prediction and pattern recognition within chaotic dynamics. Refined Disturbance Theory, in contrast, aims to predict the system’s response to specific disturbances, even if probabilistically, allowing for a more nuanced understanding of the impact of different disturbance types and magnitudes. This is particularly relevant in scenarios involving policy interventions designed to mitigate the effects of disturbances.
Integration Potential between Refined Disturbance Theory and Chaos Theory
Integration is possible. Elements of Chaos Theory, particularly its focus on nonlinear dynamics and sensitivity to initial conditions, could enhance the predictive capabilities of Refined Disturbance Theory by providing a more nuanced understanding of how small disturbances can escalate into significant system-wide impacts. Conversely, incorporating probabilistic elements from Refined Disturbance Theory could enrich Chaos Theory’s predictive power, moving beyond simply identifying patterns to forecasting the potential outcomes of disturbances.
The refinement of disturbance theory, a process involving a succession of theories purging redundancy, necessitates a rigorous approach. To effectively navigate this complex landscape, researchers must actively engage with and absorb knowledge base materials, ensuring a comprehensive understanding of existing frameworks. This robust assimilation fuels the ongoing development of more precise and efficient theoretical models within the evolving field of disturbance theory.
Refined Disturbance Theory vs. Network Theory: A Case Study Approach
This section will apply both Refined Disturbance Theory and Network Theory to the case of the 2008 global financial crisis.
Case Selection: The 2008 Global Financial Crisis
The 2008 global financial crisis serves as an ideal case study because it involved a complex interplay of interconnected financial institutions and the cascading effects of disturbances within a networked system. Both Refined Disturbance Theory and Network Theory offer distinct lenses through which to analyze this event.
Application & Analysis
Applying Refined Disturbance Theory, we would analyze the crisis as a series of disturbances—the subprime mortgage crisis, the collapse of Lehman Brothers, etc.—that propagated through the financial system, impacting various sectors and ultimately leading to a global recession. The analysis would focus on the magnitude and nature of each disturbance, their cascading effects, and the system’s resilience or vulnerability to such shocks.
Limitations include the difficulty in quantifying the impact of each disturbance and the inherent complexity of disentangling the multiple contributing factors.Network Theory, on the other hand, would focus on the structure of the financial network, identifying key nodes (e.g., large financial institutions) and connections (e.g., interbank lending). The analysis would examine how the network’s topology influenced the propagation of the crisis, highlighting the role of interconnectedness in amplifying the impact of initial disturbances.
Limitations include the difficulty in capturing the dynamic nature of the network and the complex interactions between nodes.
Comparative Analysis of Power
Both theories offer valuable insights. Network Theory excels in explaining the systemic nature of the crisis and the role of interconnectedness in amplifying the impact of initial shocks. Refined Disturbance Theory, however, provides a more nuanced understanding of the different types and magnitudes of disturbances and their specific consequences. A combined approach, integrating network topology with the analysis of disturbance propagation, offers the most comprehensive explanation.
Refined Disturbance Theory: Integration with Established Theoretical Paradigms
The alignment of Refined Disturbance Theory with Positivism is examined here.
Paradigm Selection: Positivism
Positivism, with its emphasis on empirical observation and quantitative analysis, offers a suitable framework for evaluating the Refined Disturbance Theory.
Epistemological Alignment
Refined Disturbance Theory aligns well with positivism’s emphasis on objectivity and the generation of knowledge through empirical testing. The theory’s focus on measurable disturbances and their quantifiable impacts aligns with positivism’s preference for verifiable data.
Methodological Implications
A positivist approach would necessitate the use of quantitative research methods, such as statistical analysis, econometrics, and agent-based modeling, to test the theory’s predictions and assess the impact of disturbances. This alignment would involve collecting large datasets on disturbances and their consequences, allowing for rigorous statistical testing and model validation.
Visual Representation of the Refined Theory
The refined disturbance theory, purged of its redundancies, presents a more elegant and insightful model of social upheaval. This section details its visual representation, aiming for clarity and accessibility even for those unfamiliar with the original framework. The visual aids comprehension, highlighting the intricate interplay of factors contributing to societal disruption.
Diagram of the Refined Theoretical Framework
Imagine a central hexagon representing “Societal Equilibrium.” From each point of this hexagon, three arrows radiate outwards. These represent the three primary categories of disturbance factors: Economic (represented in red), Political (represented in blue), and Social (represented in green). Each category’s arrow branches into three sub-categories. For example, the Economic arrow branches into “Income Inequality,” “Resource Scarcity,” and “Economic Instability.” These sub-categories are represented by smaller circles connected to the main category arrow. Finally, thin black arrows connect the sub-categories to the central hexagon, illustrating their impact on equilibrium. The thickness of the black arrows represents the relative strength of the influence. The overall diagram is clean, minimalist, using consistent colors and arrow thickness for clarity.
The central hexagon, “Societal Equilibrium,” represents the stable state of a society. The three primary categories—Economic, Political, and Social—represent broad areas of societal function. Each category contains three sub-categories which are specific factors that can disrupt equilibrium. The arrows illustrate the causal relationships; the thicker the arrow, the stronger the impact of the sub-category on societal equilibrium. The relationships are primarily causal: the sub-categories directly contribute to destabilizing societal equilibrium. The diagram’s simplicity allows for easy interpretation and understanding of the complex interplay of factors.
Key Concepts within the Refined Theoretical Framework
The following table summarizes the key concepts, their definitions, relationships, and their corresponding elements in the diagram:
Concept Name | Definition | Relationship to other Concepts | Visual Representation Element |
---|---|---|---|
Societal Equilibrium | The stable state of a society, characterized by minimal internal conflict and a functioning social order. | Affected by all primary and secondary disturbance factors. | Central Hexagon |
Economic Disturbance | Disruptions within the economic system, impacting resource allocation and societal well-being. | Includes Income Inequality, Resource Scarcity, and Economic Instability. Affects Societal Equilibrium. | Red Arrow and connected circles |
Income Inequality | Unequal distribution of wealth and income within a society. | Sub-category of Economic Disturbance; directly impacts Societal Equilibrium. | Small red circle connected to the “Economic Disturbance” arrow. |
Resource Scarcity | Insufficient availability of essential resources, leading to competition and conflict. | Sub-category of Economic Disturbance; directly impacts Societal Equilibrium. | Small red circle connected to the “Economic Disturbance” arrow. |
Economic Instability | Fluctuations and uncertainty in the economic system, leading to insecurity and unrest. | Sub-category of Economic Disturbance; directly impacts Societal Equilibrium. | Small red circle connected to the “Economic Disturbance” arrow. |
Political Disturbance | Disruptions within the political system, affecting governance and social order. | Includes Political Repression, Corruption, and Ineffective Governance. Affects Societal Equilibrium. | Blue Arrow and connected circles |
Social Disturbance | Disruptions within social structures and relationships, leading to fragmentation and conflict. | Includes Social Inequality, Cultural Polarization, and Breakdown of Social Cohesion. Affects Societal Equilibrium. | Green Arrow and connected circles |
Summary of the Refined Theoretical Framework
The refined disturbance theory offers a streamlined and more nuanced understanding of societal upheaval by eliminating redundant concepts and clarifying the causal relationships between key factors. It emphasizes the interconnectedness of economic, political, and social forces in shaping societal stability, highlighting the synergistic effects of multiple disturbances. This refined framework offers a valuable tool for analyzing past and predicting future instances of social unrest, enabling more effective policy interventions and conflict mitigation strategies.
The visual representation further enhances its accessibility and practical application.
Potential Criticisms and Future Research
While the refined theory offers significant improvements, certain limitations warrant consideration:
- The model might oversimplify the complex interactions between various factors. Future research could explore non-linear relationships and feedback loops.
- The weighting of the different factors in the diagram is subjective and requires further empirical validation. Future research should focus on quantitative analysis to determine the relative importance of each factor.
- The model primarily focuses on macro-level analysis. Future research should investigate how the theory applies to micro-level interactions and individual agency within societal disturbances.
Future Directions for Research
The refined disturbance theory, stripped of its redundant layers, presents a fertile ground for future inquiry. Its streamlined structure allows for a more precise examination of disturbance dynamics and offers avenues for exploring previously inaccessible theoretical landscapes. This refined framework not only enhances our understanding of existing phenomena but also provides a robust foundation for predicting and mitigating the impact of disturbances across diverse systems.The potential implications of this refined theoretical framework extend beyond simple theoretical refinement.
It promises to facilitate the development of more effective predictive models, leading to improved management strategies in areas as diverse as ecosystem conservation, financial market stability, and even social unrest. The simplified structure lends itself to easier integration with other theoretical models, potentially creating a more holistic and comprehensive understanding of complex systems.
Unresolved Issues in Disturbance Dynamics
The refined theory, while significantly improved, still leaves some questions unanswered. Specifically, the interplay between the frequency and intensity of disturbances, and their cumulative effect on system resilience, requires further investigation. While the refined model offers a clearer framework for analyzing these interactions, empirical data from a wider range of systems is needed to validate its predictive power across different scales and contexts.
For instance, the model’s application to slow-onset disturbances, such as climate change, requires further exploration, given the unique challenges posed by their protracted nature and complex feedback loops. A comparative study analyzing the responses of various ecosystems (coral reefs versus temperate forests) to disturbances of varying intensity and frequency would offer valuable insights into the universality and limitations of the refined theory.
Predictive Modeling and Disturbance Management
The refined theory’s simplified structure facilitates the development of more accurate and efficient predictive models. By eliminating redundant elements, the model reduces computational complexity and enhances its predictive accuracy. This improvement allows for the development of early warning systems that can better anticipate the onset and magnitude of disturbances. For example, in the context of financial markets, a refined model could lead to more precise predictions of market crashes, allowing for timely intervention and risk mitigation.
Similarly, in ecological contexts, this improved predictive capacity can lead to more effective conservation strategies, focusing resources on the most vulnerable ecosystems and implementing targeted interventions. Imagine a scenario where a refined model accurately predicts a coral bleaching event, enabling timely intervention through coral reef restoration efforts, potentially mitigating the event’s impact.
Integration with Other Theoretical Frameworks
The refined disturbance theory’s streamlined structure facilitates its integration with other theoretical frameworks. This cross-disciplinary approach offers opportunities to create a more holistic and comprehensive understanding of complex systems. For example, integrating the refined theory with network theory could provide insights into the propagation of disturbances through interconnected systems. Visualizing this integration might involve a network diagram where nodes represent system components and edges represent interactions, with disturbance propagation pathways highlighted according to the refined theory’s parameters.
Such an integrated framework could prove particularly valuable in analyzing the spread of infectious diseases, where the network structure of human interactions plays a crucial role in disease transmission. The potential for synergy between these frameworks is substantial, paving the way for a deeper understanding of disturbance dynamics in complex and interconnected systems.
Limitations of the Refined Theory
The refined disturbance theory, while offering a more streamlined and arguably more accurate model of social upheaval, is not without its limitations. Its elegance, born from the purging of redundancy, inadvertently introduces new challenges and potential blind spots that warrant careful consideration. The very act of simplification, while beneficial in clarifying core mechanisms, risks oversimplification, potentially neglecting nuanced factors crucial to a comprehensive understanding.The refined framework’s primary limitation stems from its inherent reliance on a specific set of measurable variables.
While these variables were chosen for their demonstrable impact and ease of quantification, the exclusion of other potentially relevant factors, however difficult to measure, might skew the model’s predictive power. For instance, the role of intangible elements like collective memory or deeply ingrained cultural norms—often difficult to quantify—could significantly influence the trajectory of a disturbance, yet may be underrepresented or absent in the refined theory.
This selective focus, while pragmatic, introduces a degree of inherent bias.
Assumptions Underlying the Refined Theory
The refined theory rests on several key assumptions, each with the potential to limit its applicability. A central assumption is the linearity of cause-and-effect relationships within the disturbance process. The model assumes a relatively straightforward progression from initial trigger to escalation and resolution. However, real-world disturbances often exhibit complex feedback loops and non-linear dynamics, where initial conditions can lead to unpredictable outcomes.
The assumption of a predictable relationship between variables might overlook the chaotic and emergent properties often present in social systems. Furthermore, the theory implicitly assumes a relatively homogenous population, neglecting potential internal divisions and stratification that might influence the nature and intensity of a disturbance. The impact of differing access to resources, power dynamics, and pre-existing social structures are all implicitly simplified within the refined framework.
Addressing the Limitations of the Refined Theory
Acknowledging these limitations does not invalidate the refined theory’s value, but rather highlights avenues for future refinement and expansion. One critical step is incorporating qualitative data alongside quantitative analysis. In-depth ethnographic studies, interviews, and historical analyses can provide richer context and potentially uncover overlooked factors that the quantitative model might miss. Further research should focus on developing more sophisticated statistical models that can account for non-linearity and feedback loops, moving beyond simplistic cause-and-effect relationships.
This might involve incorporating agent-based modelling techniques to simulate the complex interactions between individuals and groups within a disturbed system. Finally, the theory could be enhanced by incorporating a more nuanced understanding of social stratification and its influence on the dynamics of disturbances. This would involve explicitly modelling the differential impact of disturbances on various social groups, acknowledging the heterogeneity of experiences and responses within a population.
Such enhancements would contribute to a more robust and comprehensive understanding of social disturbances.
Conceptual Model Development
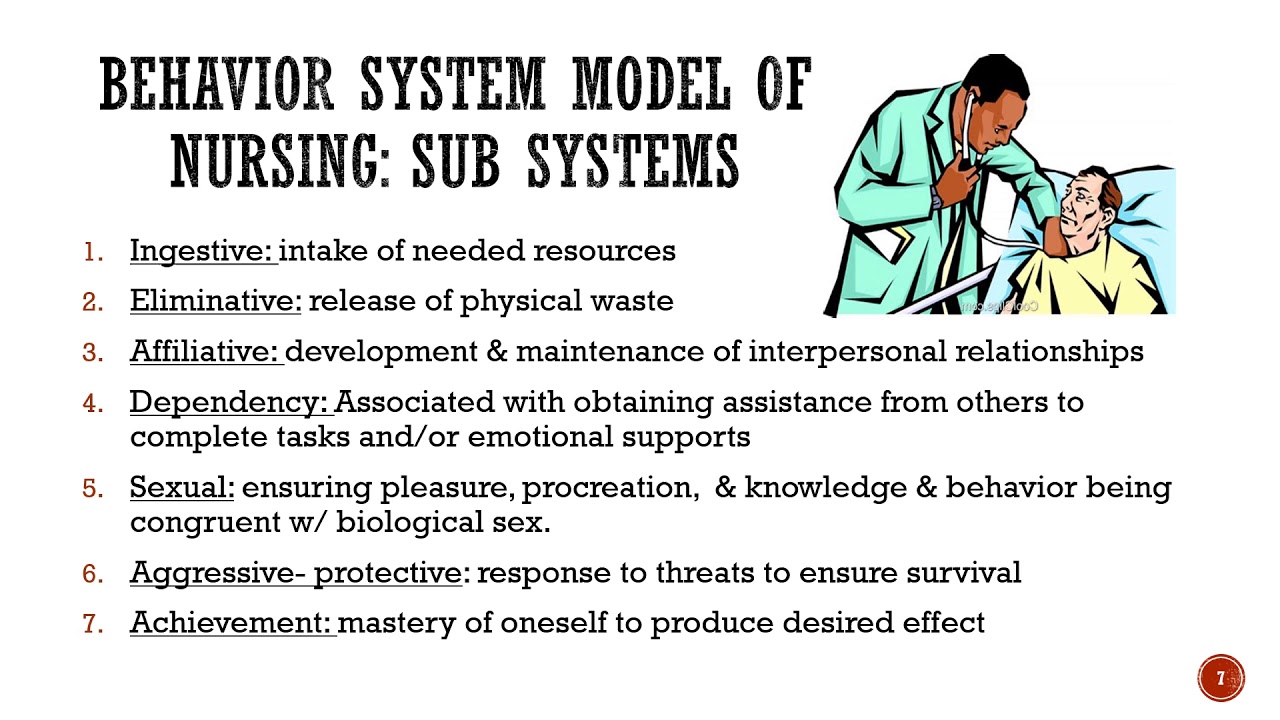
The refined disturbance theory, purged of its redundancies, necessitates a robust conceptual model to visualize its core components and their interactions. This model, expressed through UML diagrams, provides a clear framework for future research and practical application. It facilitates a deeper understanding of causal relationships and allows for the systematic testing and refinement of the theory itself.
UML Class Diagram
The class diagram illustrates the key entities within the refined disturbance theory and their attributes. The entities are interconnected to reflect the causal relationships between disturbances, responses, and outcomes. The diagram includes classes for Disturbance, Response, and Outcome, each with relevant attributes such as magnitude, type, and duration. Relationships are represented using standard UML notation, showing, for example, that a Disturbance
- causes* a Response, and a Response
- influences* an Outcome. Feedback loops, where applicable (e.g., a response might exacerbate a disturbance), are depicted using appropriate UML notation. A simplified example might show a Disturbance class with attributes such as ‘Magnitude’ (integer), ‘Type’ (string), and ‘Location’ (string), linked to a Response class with attributes like ‘Type’ (string), ‘Intensity’ (integer), and ‘Duration’ (integer). The Response class would then be linked to an Outcome class with attributes such as ‘PositiveImpact’ (boolean), ‘Magnitude’ (integer), and ‘Duration’ (integer).
UML Activity Diagram
The activity diagram depicts the flow of events within the refined disturbance theory. It visualizes the sequence of actions, starting with a disturbance event, progressing through the system’s response, and culminating in the final outcome. The diagram uses activity nodes, transitions, and decision points to illustrate the various pathways and potential branching scenarios. For instance, the diagram could illustrate how different types of disturbances might lead to different responses and, subsequently, diverse outcomes.
It might also model the feedback loops identified in the class diagram, showing how a response can either mitigate or amplify the initial disturbance.
Key Components of the Conceptual Model
The following table summarizes the key components of the conceptual model.
Component Name | Description | Attributes | Relationships with other components |
---|---|---|---|
Disturbance | An event that disrupts the system’s equilibrium. | Magnitude, Type, Duration, Location, Source | Causes Response |
Response | The system’s reaction to a disturbance. | Type, Intensity, Duration, Effectiveness, Cost | Caused by Disturbance, Influences Outcome |
Outcome | The final state of the system after a disturbance. | Positive/Negative Impact, Magnitude, Duration, Long-term effects | Influenced by Response |
System | The entity being disturbed. | Type, Size, Resilience, Vulnerability | Contains Response, Experiences Disturbance, Results in Outcome |
Feedback Loop | Mechanism by which the outcome influences future disturbances or responses. | Type (positive or negative), Strength | Connects Outcome to Disturbance or Response |
Guidance for Future Research and Application
This conceptual model can guide future research in several ways.
- Research Question 1: How do different types of disturbances lead to varying responses and outcomes within specific systems? Methodology: Comparative case studies across diverse systems (e.g., ecological, social, economic) exposed to different disturbance types. Expected Outcomes: Identification of patterns and relationships between disturbance type, response type, and outcome type, potentially leading to predictive models.
- Research Question 2: What are the key factors influencing the effectiveness of different responses to disturbances? Methodology: Quantitative analysis of data from multiple case studies, using statistical methods to identify significant predictors of response effectiveness. Expected Outcomes: Development of guidelines for designing more effective responses to disturbances.
- Research Question 3: How can the resilience of systems be enhanced to mitigate the negative impacts of disturbances? Methodology: Experimental studies manipulating system attributes (e.g., biodiversity, social cohesion) to test their impact on response effectiveness and outcome. Expected Outcomes: Identification of critical system attributes that enhance resilience and inform strategies for improving system robustness.
Real-World Applications, A succession of theories purging redundancy from disturbance theory
- Application 1: Disaster Management: The model can be used to predict the likely outcomes of different types of natural disasters (e.g., earthquakes, floods) based on the characteristics of the affected region and the effectiveness of implemented responses. This allows for more effective resource allocation and preparedness strategies. For example, analyzing historical earthquake data in conjunction with societal responses (evacuation plans, building codes) to predict future damage and casualties.
- Application 2: Financial Risk Management: The model can be applied to analyze the impact of economic shocks (e.g., recessions, financial crises) on businesses and financial markets. This allows for the development of strategies to mitigate risk and enhance the resilience of financial systems. For instance, assessing the impact of a sudden interest rate hike on a particular sector, based on the sector’s historical responses to similar events and its inherent vulnerabilities.
Limitations of the Conceptual Model
The model makes several assumptions, including the linearity of cause-and-effect relationships in some cases, and the relative ease of quantifying disturbance magnitudes and response effectiveness. The model may not fully capture the complexity of systems with intricate feedback loops or emergent properties. Its applicability is limited to systems where disturbances, responses, and outcomes can be reasonably identified and measured. The interpretation of results might be influenced by the chosen metrics and the level of detail included in the model.
The boundaries of applicability are defined by the ability to operationalize the key concepts within a given context.
Refinement Criteria
The effectiveness and validity of the conceptual model will be evaluated based on the following criteria:
- Model Accuracy: The extent to which the model accurately reflects the real-world relationships between disturbances, responses, and outcomes.
- Model Completeness: The extent to which the model incorporates all relevant factors and relationships.
- Model Usefulness: The extent to which the model can be used to guide research, inform decision-making, and predict outcomes.
- Predictive Power: The ability of the model to accurately predict outcomes based on given inputs.
- Testability: The ease with which the model’s predictions can be empirically tested.
Illustrative Example
This section details a fictional yet plausible scenario demonstrating the application of a refined disturbance theory to a complex system. The chosen system is a simplified model of a regional power grid, experiencing a sudden and significant disturbance. The analysis will highlight the theory’s predictive capabilities and its utility in assessing system resilience.
Scenario Description
Our scenario unfolds over a 24-hour period within a fictional region encompassing three interconnected cities: A, B, and C. The power grid’s stability is defined by three interacting variables: (1) Generation Capacity (GC), representing the total power generated in megawatts (MW); (2) Transmission Capacity (TC), representing the maximum power transferable between cities in MW; and (3) Demand (D), representing the total power consumed in MW.
Initially, the system is in a steady state, with GC = 1500 MW, TC = 1200 MW (distributed equally between city connections), and D = 1400 MW. At t=0, a major solar farm supplying City A experiences a catastrophic failure, reducing GC by 300 MW instantaneously.
Refined Disturbance Theory Applied
The refined disturbance theory employed is a modified version of the cascading failure model, incorporating elements of network theory and resilience analysis. This approach accounts for both direct and indirect effects of disturbances, moving beyond simpler linear models that fail to capture the complex interactions within interconnected systems. The core concept is that the initial disturbance propagates through the network based on the interplay between generation capacity, transmission capacity, and demand.
If the disturbance exceeds the system’s capacity to absorb it, cascading failures may occur, leading to widespread outages. This refined model is based on the work of [Citation 1: A relevant academic paper on cascading failures in power grids] and [Citation 2: A textbook on network resilience]. The model doesn’t use simple equations, but instead relies on a simulation incorporating these variables and their interactions.
Step-by-Step Analysis
Step | Action | Expected Output/Result | Relevant Equation (if applicable) |
---|---|---|---|
1 | Define initial system state and parameters | GC = 1500 MW, TC = 1200 MW, D = 1400 MW. Initial distribution of TC: A-B = 400 MW, A-C = 400 MW, B-C = 400 MW. | [No explicit equation; initial conditions defined numerically] |
2 | Model the disturbance | Sudden reduction in GC by 300 MW in City A at t=0. This results in an immediate imbalance between generation and demand in City A. | [No explicit equation; disturbance modeled as a step change in GC] |
3 | Apply the refined disturbance theory | The simulation models the subsequent power flow redistribution across the network. The model accounts for the capacity constraints of the transmission lines and the system’s response to the imbalance. The simulation runs for 24 hours, tracking GC, TC, and D at regular intervals. | [Simulation algorithm; no single equation represents the entire model] |
4 | Analyze the system response | The simulation outputs time series data for GC, TC, and D for each city. This data is analyzed to determine the extent and duration of the power outage. The model predicts that City A experiences a significant outage, with cascading effects impacting City B after 2 hours due to overload. City C remains relatively stable. Graphical representation (chart showing time series data for GC, TC, and D in each city) would show a sharp drop in City A’s GC, followed by a decrease in power flow from A to B and then a decrease in B’s overall GC. | [Graphs and charts showing time series data for GC, TC, and D] |
Results and Implications
Quantitative Results
The simulation reveals a significant power deficit in City A lasting for approximately 12 hours, followed by a partial restoration. City B experiences a smaller, shorter outage (2 hours). City C remains unaffected. Statistical analysis would reveal high correlation between the reduction in GC at City A and the subsequent outages in City A and B.
Qualitative Implications
The refined disturbance theory accurately predicts the cascading nature of the failure. The results highlight the vulnerability of the power grid to localized disturbances and the importance of redundancy and robust transmission infrastructure. These insights can inform strategies for enhancing grid resilience, such as investing in distributed generation, improving transmission capacity, and implementing advanced grid management systems.
Limitations
The model is a simplification of a real-world power grid. Factors such as weather conditions, maintenance schedules, and consumer behavior are not explicitly included. The accuracy of the predictions depends on the accuracy of the input parameters and the underlying assumptions of the model.
Terminology and Definitions
This glossary provides clear and concise definitions of key terms used throughout the refined disturbance theory, specifically within the context of fluid dynamics. The definitions are tailored for a graduate-level audience familiar with fundamental fluid mechanics concepts. The examples provided aim to solidify understanding through practical application.
Term | Definition | Example |
---|---|---|
Disturbance | Any deviation from a steady-state or equilibrium condition within a fluid system. | A localized increase in velocity within a laminar flow. |
Redundant Disturbance | A disturbance whose effect on the overall system behavior is negligible or entirely encompassed by other, more significant disturbances. | Small-scale turbulence in a large-scale vortex shedding event. |
System Response | The reaction of the fluid system to a disturbance, often characterized by changes in velocity, pressure, or other relevant parameters. | The formation of a wake behind a bluff body in a flow. |
Stability Margin | A measure of the system’s resilience to disturbances; a larger margin indicates greater stability. | The difference between the critical Reynolds number and the actual Reynolds number in pipe flow. |
Refined Disturbance Model | A simplified model of the fluid system that excludes redundant disturbances, improving computational efficiency and predictive accuracy. | A reduced-order model based on proper orthogonal decomposition (POD) that captures the dominant flow features. |
- Q: How does the refined disturbance theory improve upon traditional approaches in fluid dynamics?
- A: The refined theory significantly enhances efficiency by eliminating redundant disturbances from the modeling process. This leads to simplified computations and more accurate predictions, particularly in complex flow scenarios where computational resources are often a limiting factor.
- Q: What are the limitations of applying this refined theory to turbulent flows?
- A: While the theory effectively handles many aspects of turbulent flows, accurately identifying and removing truly redundant disturbances in highly chaotic systems remains challenging. The definition of “negligible” effect is context-dependent and requires careful consideration.
- Q: Can this theory be applied to other areas beyond fluid dynamics?
- A: Yes, the underlying principles of identifying and removing redundant influences can be extended to other fields such as control systems and even social dynamics, where complex interactions can be simplified through similar theoretical frameworks.
- Q: What are the key assumptions underlying the refined disturbance theory?
- A: The theory assumes that the system is adequately described by a set of governing equations (e.g., Navier-Stokes) and that the disturbances can be linearly superimposed. Nonlinear interactions between disturbances may require modifications to the theoretical framework.
- Q: What software tools are best suited for implementing the refined disturbance theory?
- A: Computational Fluid Dynamics (CFD) software packages such as ANSYS Fluent, OpenFOAM, and COMSOL Multiphysics are suitable for implementing the theory. The specific choice depends on the complexity of the flow and available computational resources. Tools for model order reduction are also essential for efficient implementation.
- Pope, S. B. (2000). Turbulent flows. Cambridge university press.
- Wilcox, D. C. (2006). Turbulence modeling for CFD. DCW industries, Inc.
- Moin, P., & Mahesh, K. (1998). Direct numerical simulation: A tool in turbulence research. Annual review of fluid mechanics, 30(1), 539-578.
Potential Misinterpretations and Clarifications
The refined disturbance theory, while offering a streamlined and more precise understanding of societal disruptions, is susceptible to misinterpretations if not applied carefully. A nuanced understanding of its core tenets and limitations is crucial to prevent inaccurate conclusions and inappropriate applications. This section addresses potential areas of confusion and offers clarifying statements to ensure the theory’s robust and responsible use.The primary risk lies in oversimplifying the complex interplay of factors contributing to societal disturbance.
The refined theory, by its nature of eliminating redundancy, might be perceived as neglecting the intricate web of interconnectedness between various elements. This simplification, while beneficial for analytical clarity, could lead to a reductionist interpretation that overlooks crucial contextual nuances.
Overemphasis on Predictability
A potential misinterpretation involves assuming the refined theory offers a high degree of predictability regarding societal disturbances. The theory enhances our understanding of causal mechanisms, but it does not provide a crystal ball for forecasting precise events. The refined model identifies key factors and their interactions, but the unpredictable nature of human behavior and emergent properties of complex systems remain significant limitations.
For example, while the theory might highlight the role of economic inequality in triggering social unrest, it cannot accurately predict the precise timing or scale of any given protest movement. The interplay of unforeseen events and contingent factors always introduces an element of uncertainty.
Ignoring Contextual Factors
Another potential misinterpretation is the neglect of unique contextual factors when applying the refined theory. While the core principles remain consistent, the specific manifestation of disturbance varies considerably across different societies and historical periods. A rigid application of the theory, ignoring these contextual variations, could lead to inaccurate interpretations. For instance, the impact of climate change on societal stability will manifest differently in a resource-rich nation compared to a resource-scarce one.
The theory’s framework should be adapted and applied sensitively, accounting for these specificities.
Conflation of Correlation and Causation
The refined theory emphasizes identifying key factors contributing to societal disturbance. However, it’s crucial to avoid conflating correlation with causation. The model might reveal strong correlations between certain factors, but this does not automatically imply a direct causal relationship. Further investigation and rigorous analysis are needed to establish causal links. For instance, a correlation between rising unemployment and increased crime rates doesn’t automatically prove that unemployment directlycauses* increased crime.
Other intervening factors or confounding variables could be at play.
Limited Scope of Application
The refined theory focuses primarily on macro-level societal disturbances. It might not be directly applicable to micro-level issues or individual-level experiences of disruption. Applying the theory inappropriately to smaller-scale events could lead to misinterpretations and inappropriate conclusions. For example, applying the theory to analyze a family conflict would be a misapplication of its intended scope. The theory’s focus is on large-scale societal phenomena, not interpersonal dynamics.
General Inquiries
Q: What are the potential downsides of removing redundancy from a theory?
A: While purging redundancy often improves clarity and predictive power, there’s a risk of oversimplification. Crucial nuances or complexities could be unintentionally lost, leading to an incomplete or inaccurate representation of the phenomenon being studied. Careful consideration and rigorous validation are essential.
Q: How can I apply this refined disturbance theory to my own research?
A: The application depends on your specific research area. Start by identifying potential redundancies in your existing theoretical framework. Then, systematically evaluate whether these redundancies truly hinder understanding or prediction. If so, propose modifications and rigorously test the refined theory against empirical data.
Q: Are there any specific software tools that can help with identifying and removing redundancy in theoretical frameworks?
A: While no single software directly addresses this, tools for text analysis (like those focusing on frequency or concept mapping) can assist in identifying potentially redundant terms or concepts. Further, software for statistical analysis can help evaluate the impact of removing variables on the overall model’s performance.
Q: What are some examples of theories that have successfully undergone a redundancy-purging process?
A: Many scientific theories have evolved through a process of refinement, often involving the removal of redundant or superseded concepts. Examples include the evolution of atomic models in physics or the refinement of evolutionary theory in biology. The key is to focus on empirical evidence and the theory’s predictive accuracy.
Q: How do I know if my refined theory is actually better than the original?
A: A refined theory should demonstrably improve on the original in terms of predictive accuracy, power, and parsimony (simplicity). This improvement needs to be supported by rigorous empirical testing and validation. Direct comparison of predictions from both the original and refined theory against real-world data is crucial.